Understanding the Audio Token Costs of OpenAI, ElevenLabs, and DeepGram
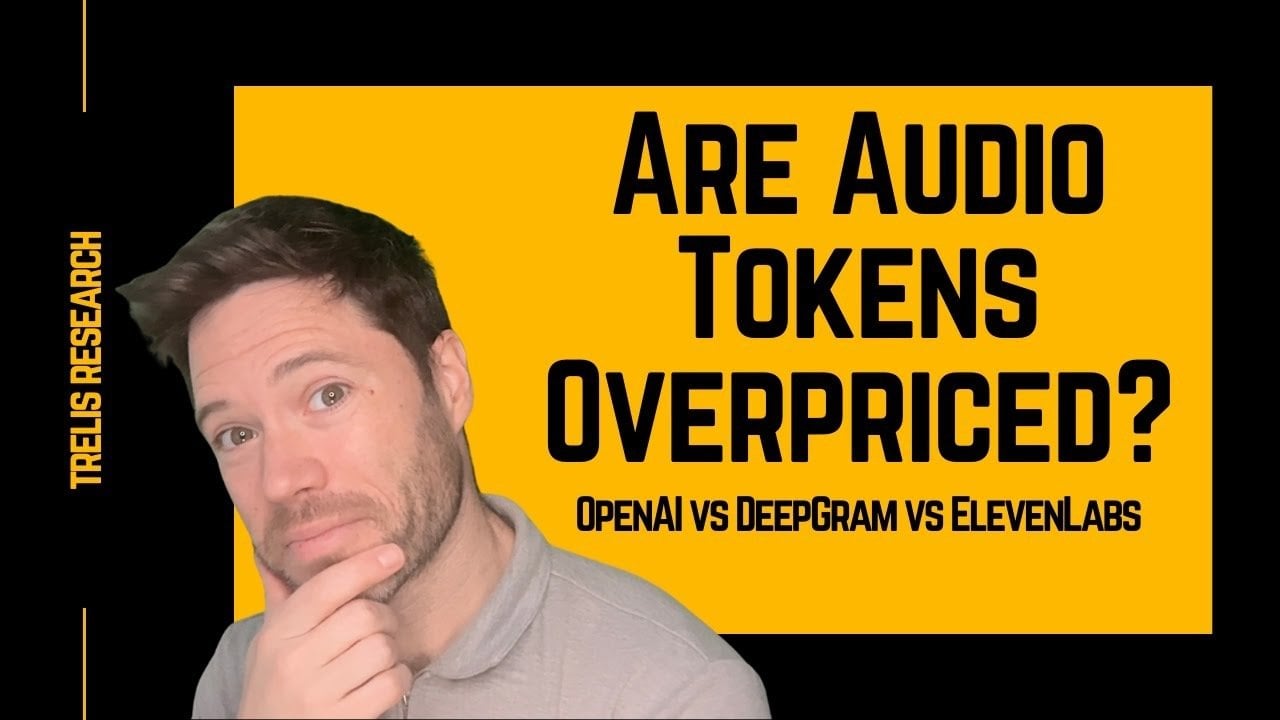
The audio processing landscape is undergoing significant changes as major companies like OpenAI, ElevenLabs, and DeepGram strive to set themselves apart in a competitive market. This ongoing rivalry is leading to a collective effort aimed at lowering costs associated with text-to-speech (TTS), speech-to-text (STT), and conversational AI technologies. The innovation in this sector is reshaping pricing strategies and helping different industries adopt these advancements more easily. Trelis Research analyzes several trends that reveal how the audio tokenization market is evolving.
Understanding Audio Tokenization and Its Costs
Key Points:
- Audio tokenization is costly due to the extensive number of tokens needed for audio data processing compared to text, raising operational expenses for TTS, STT, and conversational AI.
- OpenAI, ElevenLabs, and DeepGram utilize different pricing models: OpenAI Whisper specializes in premium STT services, ElevenLabs focuses on high-quality TTS with a higher price tag, and DeepGram offers cost-effective STT options.
- Open-source models like Fireworks and MOSI challenge the market by providing similar performance at lower costs, increasing competitive pressure on proprietary pricing.
- Multimodal models, such as OpenAI’s GPT-4, show promise in integrating diverse data types, though achieving efficient real-time performance remains difficult.
- While costs for TTS and STT are likely to decrease, conversational AI may remain a premium service due to its complex demands.
Audio tokenization is the process of converting audio into machine-readable tokens, allowing AI to process and analyze sound. This method requires significantly more computational power than handling text, as one second of high-quality audio can generate hundreds of tokens, in contrast to just a few tokens for a single text sentence. This difference explains the increased operational costs for audio processing models.
Models like OpenAI’s Whisper depend on sophisticated token generation methods and substantial computational resources, contributing to the high costs of real-time applications. However, innovations in smaller, more efficient models are emerging, allowing for more budget-friendly solutions without losing quality, indicating a potential shift in how costs are approached within the industry.
Price Comparisons: OpenAI, ElevenLabs, and DeepGram
The pricing frameworks of OpenAI, ElevenLabs, and DeepGram mirror their individual market approaches and strategies. Each has crafted unique offerings that balance performance with affordability according to user needs.
- OpenAI Whisper: This service is recognized for its powerful STT capabilities, offering competitive rates for high-end services. Although its prices are higher than some open-source options, they reflect the advanced performance of proprietary technology.
- ElevenLabs: Known for its lifelike TTS solutions, ElevenLabs commands a premium for its focus on audio quality, thus making it the costliest option among its competitors.
- DeepGram: This platform appeals to budget-sensitive businesses with its lower-priced STT solutions. However, DeepGram may need to adapt its pricing to stay competitive as the market evolves.
Additionally, open-source models such as Fireworks and MOSI are gaining popularity, providing effective alternatives that drive prices down industry-wide. This trend suggests these open-source solutions will play a more crucial role in shaping market dynamics moving forward.
Market Trends Influencing Pricing
Several ongoing trends are pushing audio tokenization costs downward. One of the most impactful is the rise of open-source audio models. Smaller, efficient architectures have shown that high-quality audio can be achieved without extensive computational costs. This focus on efficiency is expected to grow as developers prioritize making technology more scalable and cost-effective.
Meanwhile, multimodal models that combine audio, text, and visual data are also on the rise. OpenAI’s GPT-4 is an example that illustrates how unified models can streamline processes and potentially lower costs. However, these models face challenges in delivering real-time performance while remaining budget-friendly. The path forward will require careful balancing of these competing needs as innovations continue to develop.
Implications of Current Pricing for Businesses
The pricing strategies of TTS and STT service providers highlight considerable profit margins, especially in premium offerings. For instance, ElevenLabs charges for high-quality TTS solutions, while OpenAI’s audio services generally cost more than their text-only variants. These pricing variances represent possibilities for optimization as competition intensifies.
The high costs of audio services can present barriers to entry for many businesses, including startups and smaller enterprises. However, as more cost-effective models arrive and providers revise their pricing, these technologies are likely to become increasingly accessible. This evolution could potentially expand audio processing applications across various sectors.
Challenges in Reducing Conversational AI Costs
Conversational AI remains one of the most challenging and resource-heavy applications in the audio processing arena. These advanced systems need large models equipped for real-time reasoning and natural language comprehension. As a result, costs for conversational AI may not decline at the same rate as those for TTS and STT services.
OpenAI’s multimodal models, which bring together audio with other data forms, have the potential to enhance performance in this area. Balancing their complex reasoning capabilities with real-time needs could help mitigate some of the high costs associated with conversational AI. Significant reductions in expenses will demand ongoing advancements in efficiency and computational methods.
Future Prospects for Audio Tokenization Costs
The scope of audio tokenization costs is on the verge of substantial change. With advancements in smaller, more efficient models, the expenses linked to TTS and STT services will likely experience notable decreases. Open-source initiatives are set to play a vital role in this transition, providing affordable alternatives to proprietary systems and enhancing competition in the marketplace.
Conversational AI, however, may still occupy the higher end of the cost spectrum due to the complexities involved. Continuous innovation will be essential to ensure these services remain both high-performing and affordable, catering to a diverse range of users across different sectors. As the field evolves, the interaction of proprietary and open-source solutions will significantly influence the future of audio processing costs, igniting new prospects for businesses and developers.