Nasa Discovers Reliability Issues with Generative AI
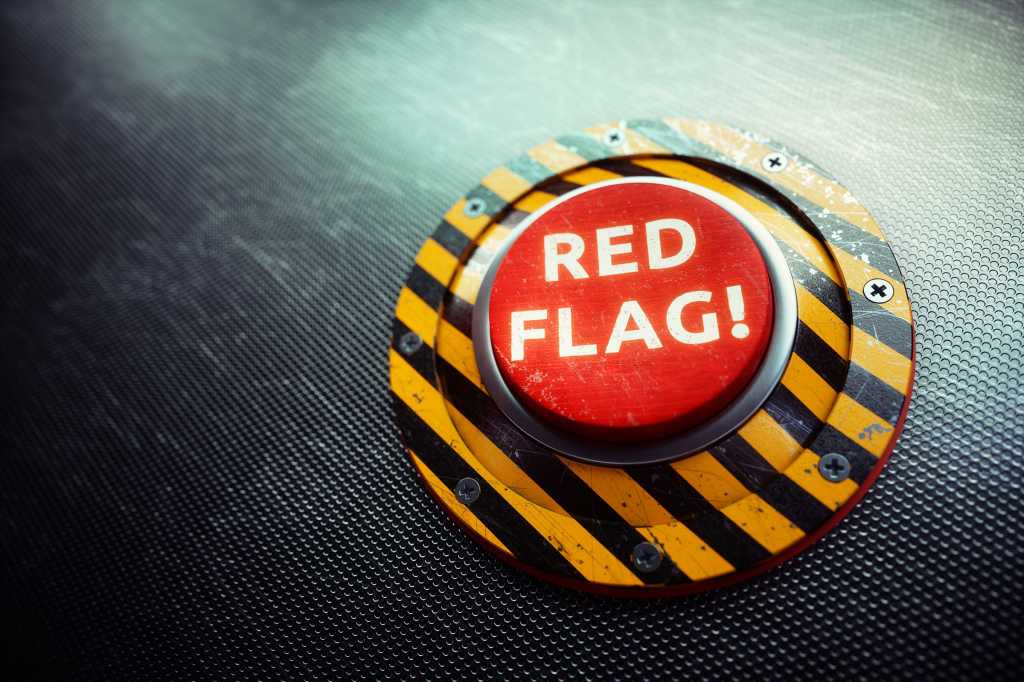
NASA’s Insights on Generative AI Trustworthiness
In a recent assessment, NASA has raised concerns about the reliability of generative artificial intelligence (AI) technologies. As AI continues to advance and permeate various sectors, including space exploration, understanding its limitations is crucial.
What is Generative AI?
Generative AI refers to systems that can generate text, images, audio, and other forms of content. This technology leverages vast datasets and sophisticated algorithms to create outputs that often mimic human creativity and decision-making. However, despite its capabilities, questions around its reliability and trustworthiness have emerged.
Key Features of Generative AI
- Data-driven Creation: It generates content based on patterns and structures learned from large datasets.
- Versatility: It can be applied in numerous fields such as art, writing, music, and even scientific research.
- Continuous Learning: These systems can improve over time as they are exposed to more data and user interactions.
NASA’s Findings on AI’s Trust Issues
NASA’s evaluation of generative AI highlighted several fundamental issues that raise doubts about its dependability:
Inaccurate Outputs
One of the main concerns is that generative AI can produce misinformation or unreliable content. This is especially critical in fields where accuracy is paramount, such as science and engineering.
Lack of Accountability
Generative AI systems operate without human oversight, leading to difficulties in tracing accountability when errors occur. When incorrect information is disseminated, it can be challenging to pinpoint the source or rectify the situation.
Ethical Concerns
The use of generative AI also raises ethical dilemmas. For instance, AI-generated content can blur the lines between human-generated and machine-generated materials, leading to issues of authenticity and authorship.
Bias in Algorithms
Another major problem is the potential for bias within AI systems. These biases originate from the datasets used for training AI models, which may reflect societal inequalities or stereotypes. Consequently, the outputs can perpetuate these biases, resulting in unfair or discriminatory content.
Implications for Industry and Society
As generative AI becomes more prevalent in industries ranging from healthcare to entertainment, its implications cannot be overlooked.
Application in Various Sectors
- Healthcare: Generative AI is being tested for creating personalized treatment plans, but it must be reliable to ensure patient safety.
- Education: AI can assist in creating educational materials, but incorrect information could mislead learners.
- Entertainment: In creative industries, while generative AI can enhance content production, its inaccuracies might undermine artistic integrity.
Need for Regulation and Oversight
Given the risks, experts advocate for more stringent regulations and oversight of generative AI. This includes developing standardized practices for AI usage and creating frameworks for accountability.
Future Directions for Generative AI
To improve the trustworthiness of generative AI, several steps can be taken:
Enhanced Data Curation
Ensuring that the data used to train AI systems is accurate, diverse, and representative can help mitigate biases and inaccuracies.
Greater Transparency
Developing AI systems that are transparent about their decision-making processes can foster trust and allow users to understand how outputs are generated.
Human-AI Collaboration
Encouraging human involvement in the AI generation process can help ensure that outputs are vetted and accurate, reducing the likelihood of misinformation.
Ongoing Research
Continuous research into the ethical implications and technical improvements of generative AI will be essential for its safe integration into society.
By addressing the challenges identified by NASA, stakeholders can work towards creating a future where generative AI technologies are not only innovative but also trustworthy and beneficial for all.