New Approach Revealed for Understanding an AI’s Internal Processes
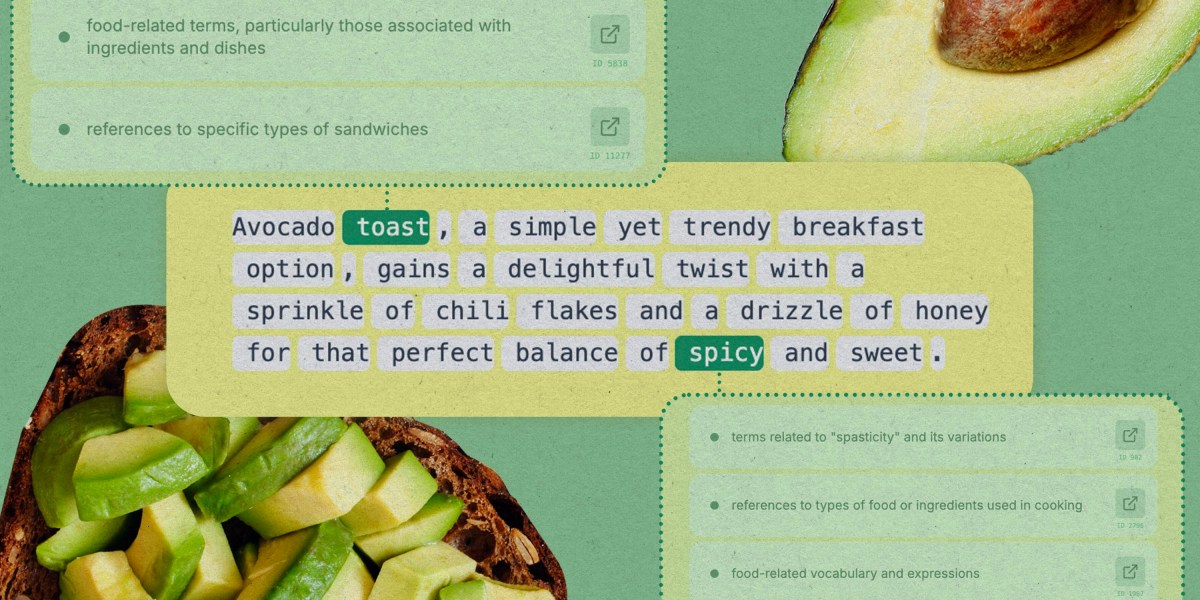
Understanding Neuronpedia and Gemma Scope: Mechanistic Interpretability in AI
Introduction to Neuronpedia and Gemma Scope
Neuronpedia is an innovative platform focused on mechanistic interpretability, which is essential for understanding how AI models process information. In July, Neuronpedia collaborated with DeepMind to create a demo called Gemma Scope, which users can explore interactively. The demo allows you to input various prompts and observe how the AI model dissects these inputs, showcasing which "features" or parts of the model are activated in response to your queries.
Interactive Features of Gemma Scope
By using Gemma Scope, users can experiment with different settings and witness how the model reacts. For instance, if you amplify the feature related to dogs and then ask the model about U.S. presidents, it may surprising introduce unrelated dog content or even simulate barking. This playful interaction reveals the quirky side of AI responses and highlights the underlying mechanics at play.
The Role of Sparse Autoencoders in Understanding AI
One of the key components of this research is sparse autoencoders, which are designed to identify features independently without pre-labeled data. This unsupervised learning approach often leads to unexpected insights into how models deconstruct human concepts. Joseph Bloom, the scientific lead at Neuronpedia, described a feature related to the concept of "cringe." This feature tends to activate in critical discussions about texts and films, illustrating how AI can connect with human emotions.
Analyzing Features with Neuronpedia
Neuronpedia serves as a valuable tool for exploring these features. When you search for specific concepts, the platform highlights which features are activated by particular words in a text and the intensity of that activation. For example, a strong activation of the "cringe" feature often appears in scenarios where someone is being overly preachy, as indicated by the model’s response.
Challenges in Identifying Features
While some features, like "cringe," are easier to identify, others are not as straightforward. Johnny Lin, the founder of Neuronpedia, pointed out that finding an accurate representation of "deception" within a model remains elusive. Despite rigorous efforts, researchers have struggled to pinpoint a specific feature that would decisively indicate when a model is generating deceptive information.
Similar Research Efforts
DeepMind’s work mirrors similar experiments conducted by other AI companies, such as Anthropic’s Golden Gate Claude project. In that instance, the researchers utilized sparse autoencoders to identify activations related to the Golden Gate Bridge. They exaggerated these activations, causing Claude to respond as if it were the bridge itself, further emphasizing the model’s unique interpretations.
Practical Applications of Mechanistic Interpretability
The implications of mechanistic interpretability research extend beyond academic curiosity. According to researchers, by understanding how models generalize and function at different levels of abstraction, designers can improve the reliability and fairness of AI systems. For instance, a team led by Samuel Marks utilized sparse autoencoders to expose biases related to gender associations in specific professions. By disabling these biased features, they aimed to create a more balanced model. However, it’s important to note that these experiments have been conducted on smaller models, raising questions about their applicability to larger systems.
Uncovering AI Errors
Research into mechanistic interpretability also sheds light on how AI makes mistakes. A standout example involved a misconception where an AI model asserted that "9.11 is greater than 9.8." Researchers from Transluce discovered that this confusion arose because the model associated these numbers with significant historical dates. Recognizing the source of the error allowed them to adjust the model’s activations related to those dates, resulting in correct future answers.
Through ongoing exploration of these features and behaviors, researchers aim to enhance the transparency and functionality of AI systems, ensuring they better align with human values and understanding.