Nvidia’s Llama-3.1 Nemotron Ultra Surpasses DeepSeek R1 with Half the Size
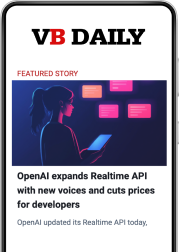
Nvidia’s New LLM: Llama-3.1-Nemotron-Ultra-253B
Recently, Nvidia launched an innovative open-source large language model (LLM) called Llama-3.1-Nemotron-Ultra-253B. This model is built on Meta’s previous Llama-3.1-405B-Instruct model and reportedly demonstrates impressive performance across various benchmarks, surpassing other established models like DeepSeek R1.
Technical Specifications
Llama-3.1-Nemotron-Ultra-253B features a comprehensive architecture with 253 billion parameters. Designed to facilitate complex reasoning and support AI assistant workflows, it emphasizes performance enhancement through innovative architecture and targeted post-training techniques. Nvidia revealed the model code on Hugging Face, making it readily accessible for developers.
Efficient Inference Design
This model prioritizes efficient inference, demonstrated through a unique architecture tailored for optimized performance. Innovations such as skipped attention layers, fused feedforward networks (FFNs), and adjustable FFN compression ratios allow the model to have a lower memory footprint. This results in not only cost-effective deployment but also effective functioning on a dedicated 8x H100 GPU node.
Additionally, Llama-3.1-Nemotron-Ultra-253B supports Nvidia’s B100 and Hopper microarchitectures and is validated for operation in both BF16 and FP8 precision modes, enhancing flexibility in various environments.
Post-Training Improvements
The model underwent a comprehensive post-training process that included supervised fine-tuning in diverse areas such as mathematics, generating computer code, and chat functionalities. Nvidia implemented a multi-phase pipeline, using Group Relative Policy Optimization (GRPO) to elevate instruction-following accuracy and reasoning capabilities.
Moreover, the model’s training involved processing over 153 billion tokens enriched with data from varied sources, enabling it to improve significantly in understanding different reasoning modes.
Performance Boost Across Different Benchmarks
The Llama-3.1-Nemotron-Ultra-253B model exhibited significant improvements over standard modes, achieving outstanding results across multiple benchmarks. For example, performance in the MATH500 benchmark surged from 80.40% to 97.00% in reasoning mode. A similar trend occurred on the AIME25 benchmark, climbing from 16.67% to 72.50%, while LiveCodeBench results more than doubled from 29.03% to 66.31%.
These enhancements were observed in various tasks including tool-based activities and general question-answering formats, with the model achieving a score of 76.01% in reasoning mode compared to 56.60% in standard mode.
Compatibility and Usage
The model is designed to be compatible with the Hugging Face Transformers library, specifically version 4.48.3. Supporting input and output sequence lengths of up to 128,000 tokens offers developers significant flexibility when implementing it into their applications.
For reasoning tasks, Nvidia suggests utilizing temperature sampling (0.6) with a top-p value of 0.95 to optimize outcomes, while recommending greedy decoding for deterministic outputs. Furthermore, Llama-3.1-Nemotron-Ultra-253B accommodates multilingual applications, covering languages such as English, German, French, Italian, Portuguese, Hindi, Spanish, and Thai.
Licensing for Commercial Use
This model is released under the Nvidia Open Model License, allowing for commercial applications. Nvidia emphasizes the necessity of responsible AI development, urging organizations to evaluate the model’s alignment, safety, and bias in relation to their specific projects.
As shared by Oleksii Kuchaiev, Director of AI Model Post-Training at Nvidia, the model brings together innovative design with open accessibility. By offering a robust 253 billion parameter architecture, it aims to enhance a wide range of applications in AI development.