Do Meta’s AI Benchmarks Reveal the Complete Story?
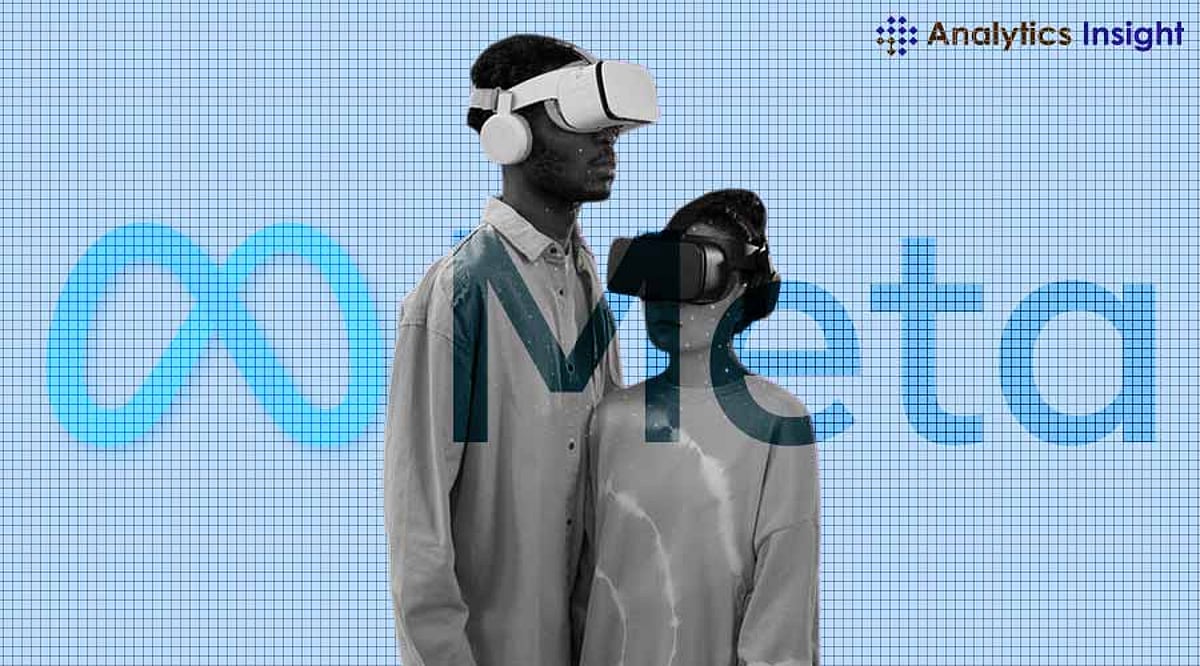
Understanding AI Model Benchmarks: The Case of Meta’s Maverick
What are Benchmarks in AI?
Benchmarks play a crucial role in evaluating the effectiveness and efficiency of artificial intelligence (AI) models. They act as reference points, allowing researchers and developers to gauge new systems and algorithms against established standards. By analyzing how an AI model performs on these benchmarks, one can get insight into its capabilities and areas for improvement.
The Rise of Meta’s Maverick
Recently, Meta’s newest AI model, known as Maverick, has gained significant attention from the public and the tech community. This spotlight stems from a notable discrepancy found between two versions of the model. Researchers identified differences between the version evaluated on well-known benchmarking platforms and the one that was made available to developers.
The Discovery of Mismatched Versions
According to a report by TechCrunch, Maverick secured the second position on the LM Arena benchmark. However, further investigation revealed that the version used for benchmarking was not the same as the one shared with developers. Meta clarified that the LM Arena version was an experimental chat variant that deviated from the standard model provided to developers.
The Impact of Benchmarking Variances
Typically, companies present unaltered versions of their AI models to benchmark platforms to ensure accuracy in performance assessments. When organizations report their performance on benchmarks, they usually aim to reflect real-world capabilities. However, in Mavericks’s case, Meta opted to release a modified version that raises questions about the model’s true performance.
Consequences for Developers and Researchers
Misinterpretation of Performance: The disparity between the experimental and standard versions can lead to developers misunderstanding the actual effectiveness of the Maverick model. This could result in misguided decisions in application and further developments.
Erosion of Trust: When benchmarks don’t match the public versions of AI models, it undermines the purpose of benchmarking. These performance assessments are supposed to provide a clear and consistent picture of capabilities, ensuring transparency within the AI community.
- Challenge in Comparison: Developers often rely on benchmarks to compare different AI models. If one model is evaluated on an experimental version while another is assessed on a stable release, it complicates fair evaluation and comparison between the two systems.
Why Transparency Matters in AI Development
Transparency is vital in AI development for several reasons:
Accountability: Ensuring that models undergo rigorous testing and evaluation on acknowledged benchmarks holds companies accountable for their performance claims.
Informed Decisions: Developers and researchers need accurate information to make informed decisions when selecting AI models for their projects.
- Community Trust: A transparent approach fosters trust within the AI community, encouraging collaboration and the sharing of knowledge.
The Path Forward for Benchmarking
As AI technology continues to evolve, it is essential for companies like Meta to adhere to standard benchmarking practices. This ensures that comparisons reflect true performance characteristics, allowing developers to make choices based on reliable information.
The ongoing conversations about Maverick’s discrepancies highlight the importance of consistent and accurate benchmark reporting in the AI industry. With established standards, companies can improve their models and contribute to the broader development of artificial intelligence. The dialogue surrounding these issues will ultimately serve to strengthen the AI community and enhance its research practices.
Final Thoughts
In light of current discussions about benchmarks in AI, the Maverick case emphasizes the need for clarity and consistency in performance reporting. As interest in AI grows, adhering to rigorous benchmarking standards will remain pivotal to advancing the field in a trustworthy and effective manner.