Meta AI Leader Claims Large Language Models Will Not Achieve Human-Level Intelligence
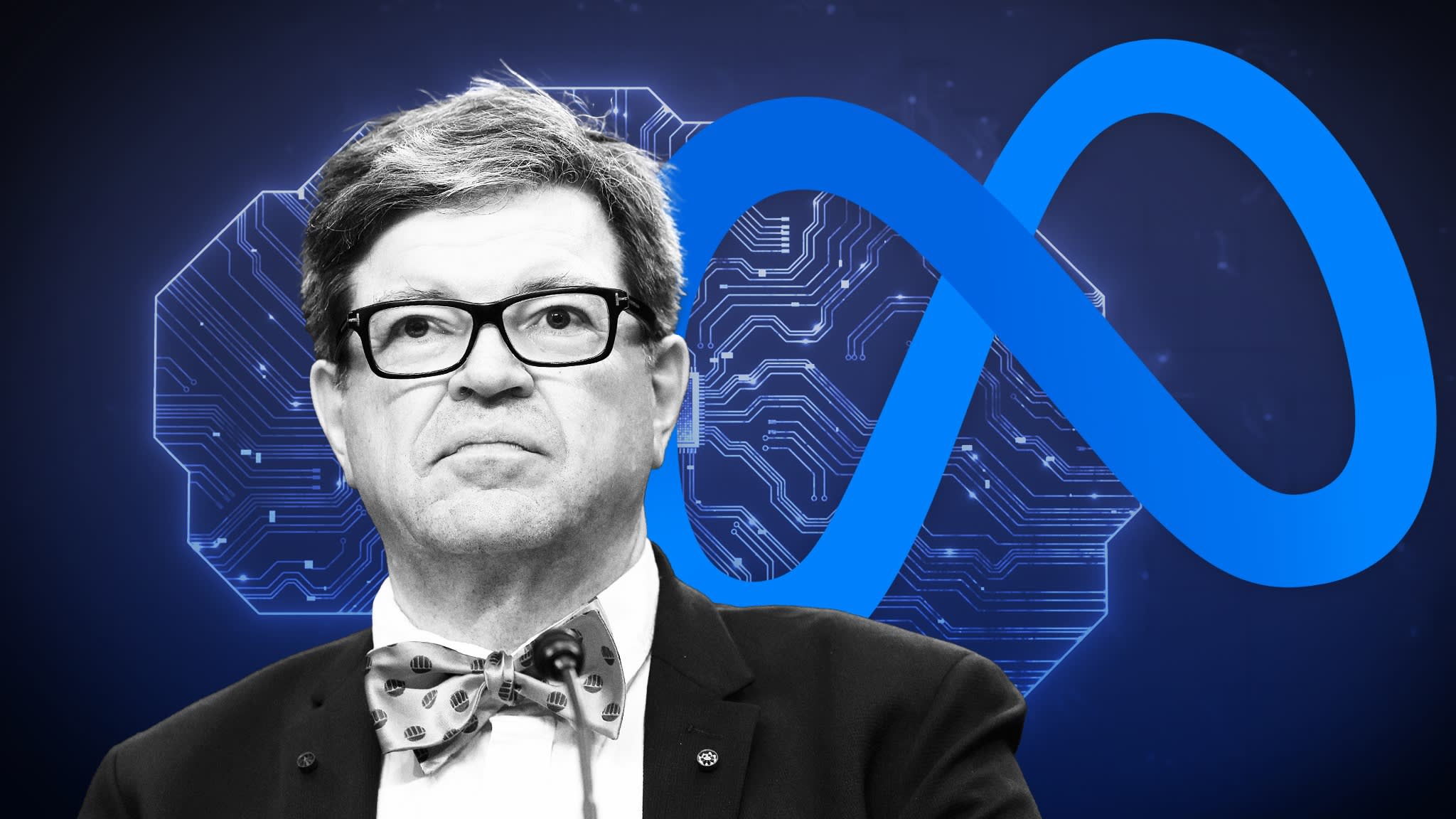
Understanding the Limitations of Large Language Models
Recent discussions in the technology community, especially from Meta’s AI leadership, highlight an important topic: the limitations of large language models (LLMs) like GPT. While today’s advancements in artificial intelligence are impressive, the suggestion that these models could achieve human-like intelligence remains contentious.
What Are Large Language Models?
Large language models are a type of artificial intelligence designed to understand, generate, and respond to human language. These models are trained on vast datasets, enabling them to produce text that resembles human speech or writing. They work through complex algorithms and machine learning techniques but fundamentally differ from human cognition.
Key Characteristics of LLMs
Data-Driven Learning: LLMs rely heavily on the data they’re trained on. They identify patterns in language from extensive collections of text, allowing them to predict and generate coherent sentences. However, they do not possess an understanding of context in the same way humans do.
Pattern Recognition: At their core, these models excel at recognizing patterns rather than comprehending meaning. This means they can provide sensible responses based on learned data but lack true comprehension of the concepts or emotions behind the language.
- Mechanical Output: The outputs from LLMs are based entirely on statistical probabilities. They generate text that seems relevant or logical based on previous patterns but do so without any intrinsic understanding.
The Debate Over Intelligence
Meta’s AI chief has made a bold statement regarding the future of LLMs: they are unlikely to reach the level of human intelligence. Here’s why this claim is gaining attention:
Cognitive Differences
Understanding vs. Mimicking: Human intelligence encompasses self-awareness, emotions, and the ability to understand complex social nuances. In contrast, LLMs merely mimic language rather than genuinely understanding it.
- Common Sense Knowledge: Humans naturally apply a variety of frameworks when interacting with the world. LLMs, although trained on extensive data, lack this depth of common sense reasoning and are limited by the information they were trained on.
Limitations in Learning
Static Learning: Once trained, LLMs do not learn or adapt unless they undergo additional training sessions. In contrast, human cognition involves continuous learning adapted from daily experiences and social interactions.
- Contextual Awareness: While LLMs can process and generate dialogue based on immediate prompts, they struggle with long-term contextual awareness—a crucial aspect of human conversations.
Implications for the Future of AI
Given these limitations, it’s vital to have realistic expectations about the capabilities of large language models. Here are some considerations for stakeholders in AI development:
Ethical Guidelines: As AI continues to evolve, establishing ethical policies for development and application is essential. This ensures that AI tools are used responsibly and do not mislead or create false expectations.
Complementing Human Skills: Instead of attempting to replicate human intelligence, LLMs should be seen as tools that can enhance human capabilities. They can assist in various tasks, such as drafting text or providing suggestions, thus allowing humans to focus on more complex decision-making processes.
- Ongoing Research: The AI field remains active and enthusiastic about improving technology. While LLMs may never reach human-like understanding, advancements could lead to more sophisticated models that further bridge the gap between human and machine interactions.
Summary
The advancements of large language models have raised significant discussions about the nature of intelligence in machines. As we explore the potential applications and limits of these models, clarity on their capabilities, as well as their distinctions from human cognition, will be critical for shaping the future of artificial intelligence.