Exploring Microsoft’s AI Red Team
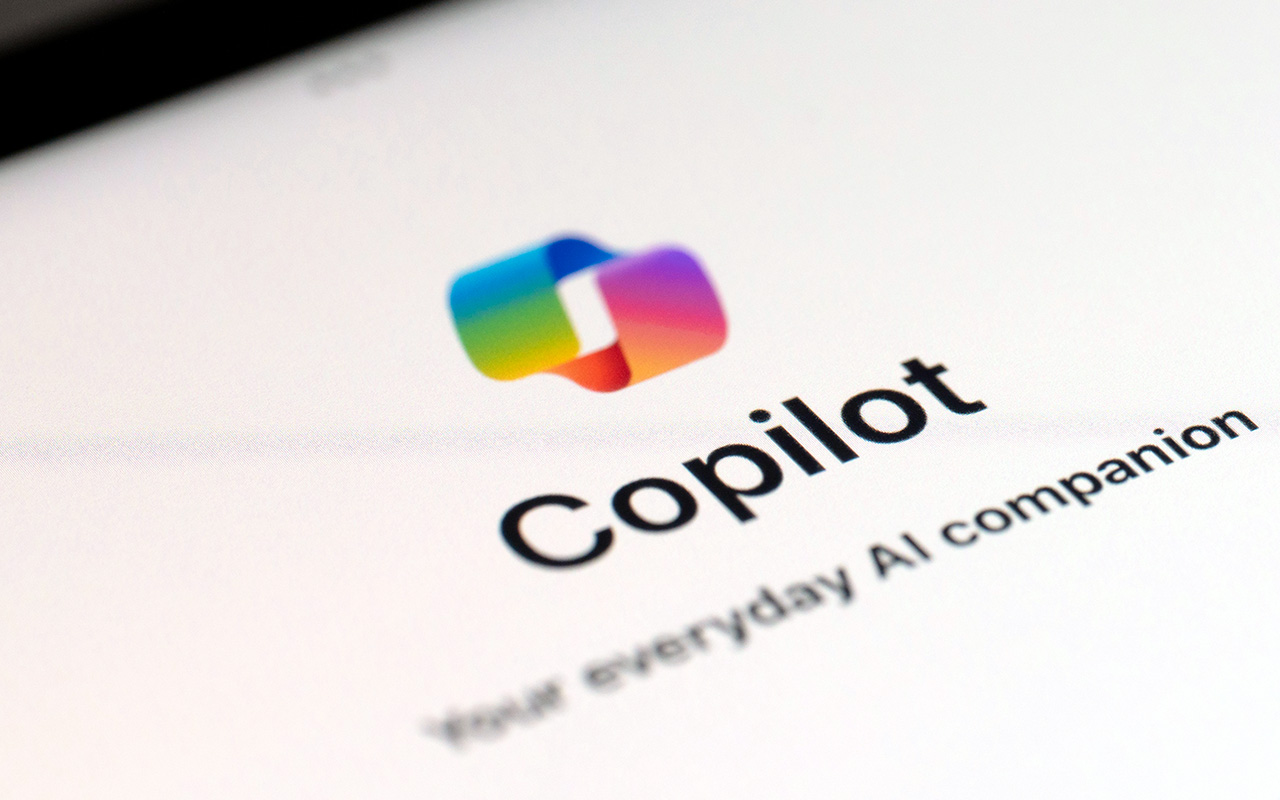
Understanding AI Red Teaming: A Vital Component for AI Security
AI red teaming, also known as adversarial machine learning, has its roots in the computer science field but has gained significant importance in recent years. As artificial intelligence systems become widespread, the role of red teams—groups that simulate attackers to identify vulnerabilities—has become crucial for securing a safe AI future.
What is AI Red Teaming?
At its essence, red teaming involves imitating real-world attacks to test AI systems. This could mean role-playing as malicious actors or even unsuspecting users who could unintentionally exploit weaknesses in an AI system. The primary goal is to "break" the technology in order to enhance its resilience and security.
The Journey of AI Red Teams
In 2018, I established the AI Red Team at Microsoft. Since its inception, we have evaluated over 100 Generative AI (GenAI) applications. This includes flagship models available on Azure OpenAI and various Copilot releases. If a company executive showcases a Copilot, the likelihood is that our team has rigorously tested it beforehand.
Key Observations in Red Teaming
As we dove deeper into AI red teaming, several patterns emerged:
- Safety of Smaller Models: Generally, smaller models tend to be more secure than larger ones.
- Continuing Relevance of Traditional Security Exploits: Conventional hacking strategies still pose significant threats.
- Diverse Expertise Required: Evaluating AI systems effectively necessitates insight from a variety of fields beyond simple technical skills, including life sciences and social science.
Challenges in AI Security
Lack of Inherent Security in AI
Warnings about AI systems lacking security measures have been present for many years. As early as 2002, researchers cautioned that AI designs often overlook security risks. In a noteworthy demonstration in 2016, researchers tricked an image recognition system into misidentifying a panda as a gibbon. Unfortunately, this didn’t grab much attention from industry leaders.
Against this backdrop, we initiated the first industry-specific red team focused on AI, prioritizing a proactive approach to identifying failures.
A Shift in Paradigm
When we accessed GPT-4 before its public release in 2022, it became clear that our previous frameworks no longer applied. The sudden shift required us to understand anew how AI systems could be attacked, who the potential attackers might be, and the implications of these vulnerabilities.
Addressing the New Kind of Threats
Evolving Adversaries in the AI Landscape
With the rise of Generative AI, a new category of adversaries has emerged. Traditional threats from experienced hackers or nation-state actors still exist, but now we also face individuals with minimal technical skills who can employ creativity for harmful purposes. The barrier to entry for malicious actors has sharply decreased.
Some threats stem from so-called "dangerous capabilities." For instance, we must ensure that foundational AI models do not have the ability to inadvertently generate harmful content. Other risks are more psychosocial in nature; users might turn to AI for support during emotionally challenging times, and if the system misguides them, it could compound their distress.
Understanding Jailbreaks in AI
A common misconception is that AI jailbreaks solely serve to bypass content safety measures. However, our findings reveal that they can also facilitate security breaches through methods aimed at system manipulation. Although users on forums might discuss complex jailbreaks, the most widespread methods often focus on simplistic approaches.
Interestingly, larger models tend to be more prone to these jailbreaks due to their tendency to comply with extensive reinforcement learning. In contrast, smaller models often resist following instructions, making them surprisingly more secure.
The Importance of Human Expertise
As we engage in AI red teaming, human insight becomes indispensable. While tools like PyRIT aid our efforts, the evaluation of potential dangerous capabilities must rely on human judgment. Only skilled professionals can discern subtle biases or discomfort in AI-generated outputs.
Because our AI red team operates globally, we incorporate members fluent in various languages—this linguistic diversity helps address potential cultural and societal impacts stemming from AI usage. Our team’s composition includes experts from numerous fields, reflecting various life experiences and backgrounds. This diversity enriches our capability to identify and mitigate risks in AI systems.
In conclusion, the ongoing challenge of enhancing AI security requires continuous effort and adaptability. Collaboration among various disciplines is essential to ensuring that AI technologies remain safe and effective for all users. As threats evolve, so must our strategies, emphasizing the integration of human expertise with technical capabilities.