Understanding Sparsity: Insights from Apple Researchers on DeepSeek AI
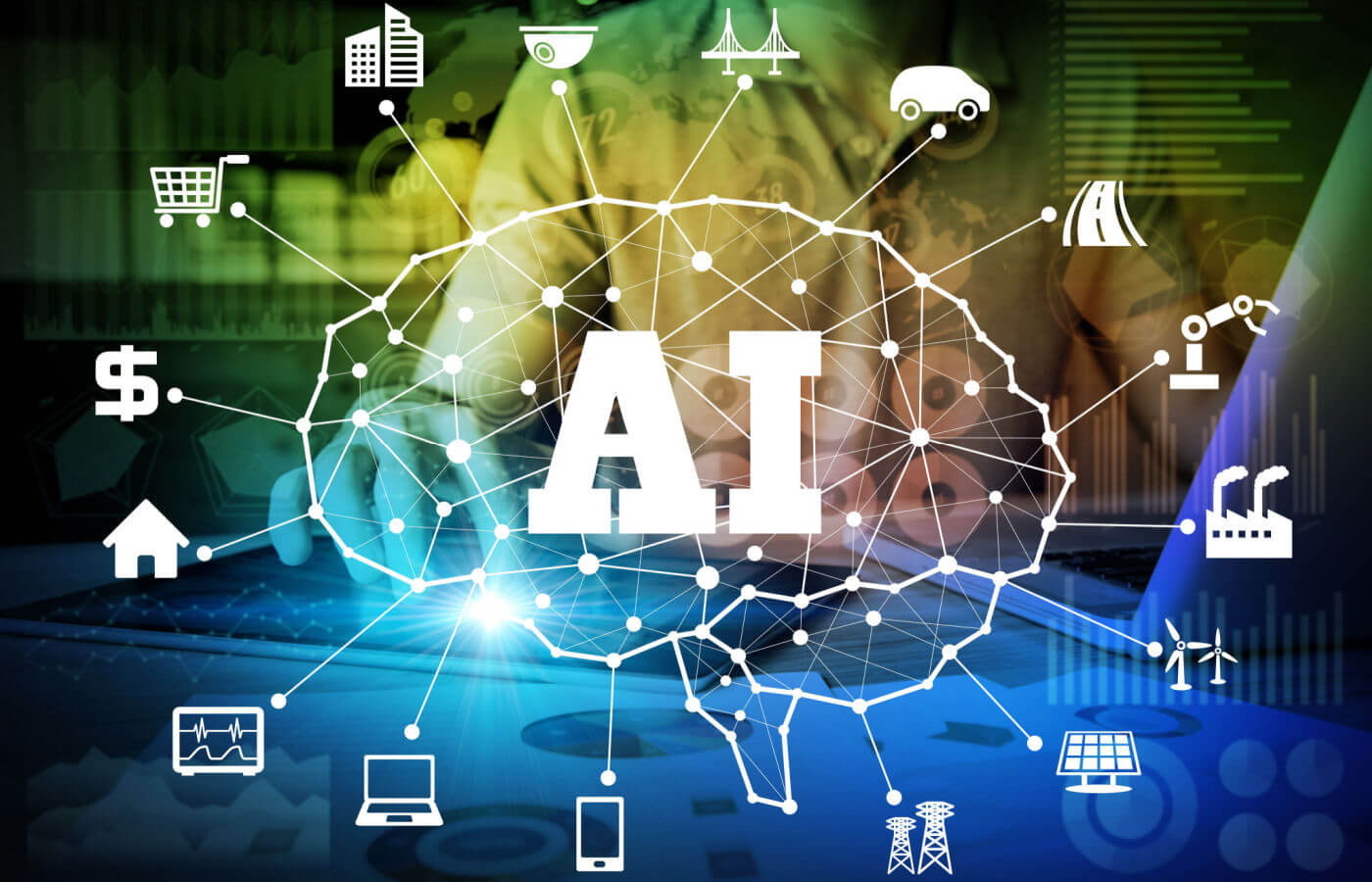
Introduction to DeepSeek’s Impact on AI
Recently, the artificial intelligence (AI) market and the broader stock market experienced a significant shift due to the emergence of DeepSeek, a powerful open-source large language model (LLM) created by a China-based hedge fund. Not only has it outperformed some of the best models from OpenAI on specific tasks, but it also does so at a fraction of the cost. This development marks a pivotal moment for smaller research labs and developers, allowing them to create competitive models and expand the diversity of options in the AI landscape.
Understanding DeepSeek’s Success
The Role of Sparsity in AI
DeepSeek’s effectiveness stems from a unique approach within deep learning known as "sparsity." Sparsity involves focusing computational efforts only on the most vital elements of data. By ignoring data components that don’t significantly influence model outcomes, AI models can operate more efficiently.
There are two forms of sparsity:
- Selective Data Removal: This technique eliminates parts of the data set that do not drive meaningful outputs.
- Network Simplification: This involves disabling entire sections of neural networks if they do not impact model performance.
DeepSeek exemplifies the latter strategy by performing parsimonious computations, allowing it to use fewer resources effectively.
Neural Network Parameters
A notable feature of DeepSeek is its capability to activate or deactivate vast sections of its neural network parameters, which determine how the model processes input data to generate text or images. When parameters are turned off, there’s a direct consequence on computation time—fewer active parameters generally lead to quicker calculations and lower energy requirements.
The Importance of Sparsity
Computing Efficiency
In a January report by Apple AI researchers, the significance of sparsity was highlighted. Their findings demonstrated that systems like DeepSeek leverage this concept to enhance performance within a fixed computational framework. By examining scenarios where they turned off various parts of a neural network, the researchers aimed to discover the optimal balance for using parameters effectively.
Sparsity’s principle is straightforward: for any given size of a neural network, less can be more. Researchers found that turning off unnecessary parameters allows models to achieve the same or even improved accuracy in tasks, such as math and question answering, without needing additional computational power.
Optimal Sparsity for Performance
The study published on the arXiv preprint server revealed that for every computing level, there exists an optimal amount of neural weights that can be deactivated to maintain accuracy. Essentially, this optimal sparsity ensures the balance between computational efficiency and performance accuracy. This concept mirrors economic principles observed in personal computing—better results for the same cost or equivalent results for less expenditure.
Achieving Better Results with Fewer Resources
As researchers observed, increasing the sparsity of a model while also expanding the total number of parameters typically leads to improved performance, as measured by pretraining loss—a metric indicating the model’s accuracy. By shutting down more parts of a neural network, DeepSeek can deliver competitive results despite having lower computational resources.
Sparsity as a Strategic Tool
Sparsity acts like a "magic dial" for AI models, enabling developers to find the ideal fit for their specific computing scenarios and budgets. This strategic use not only optimizes performance on a limited budget but also allows for enhanced results when more resources are available.
The Future of Sparsity Research in AI
Sparsity is not a new topic in AI research, but it continues to evolve and shape the field. Companies like Intel and various startups have long recognized its potential and are actively exploring new methods to leverage it for superior performance.
In summary, the insights gained from DeepSeek’s development underscore broader approaches to AI optimization. As more research and innovations emerge, the principles established by DeepSeek will almost certainly influence future AI technologies and applications, highlighting the critical role that efficiency and computational resource management play in advancing artificial intelligence.