DeepSeek AI Operates in Near Real-Time on Unconventional Chips
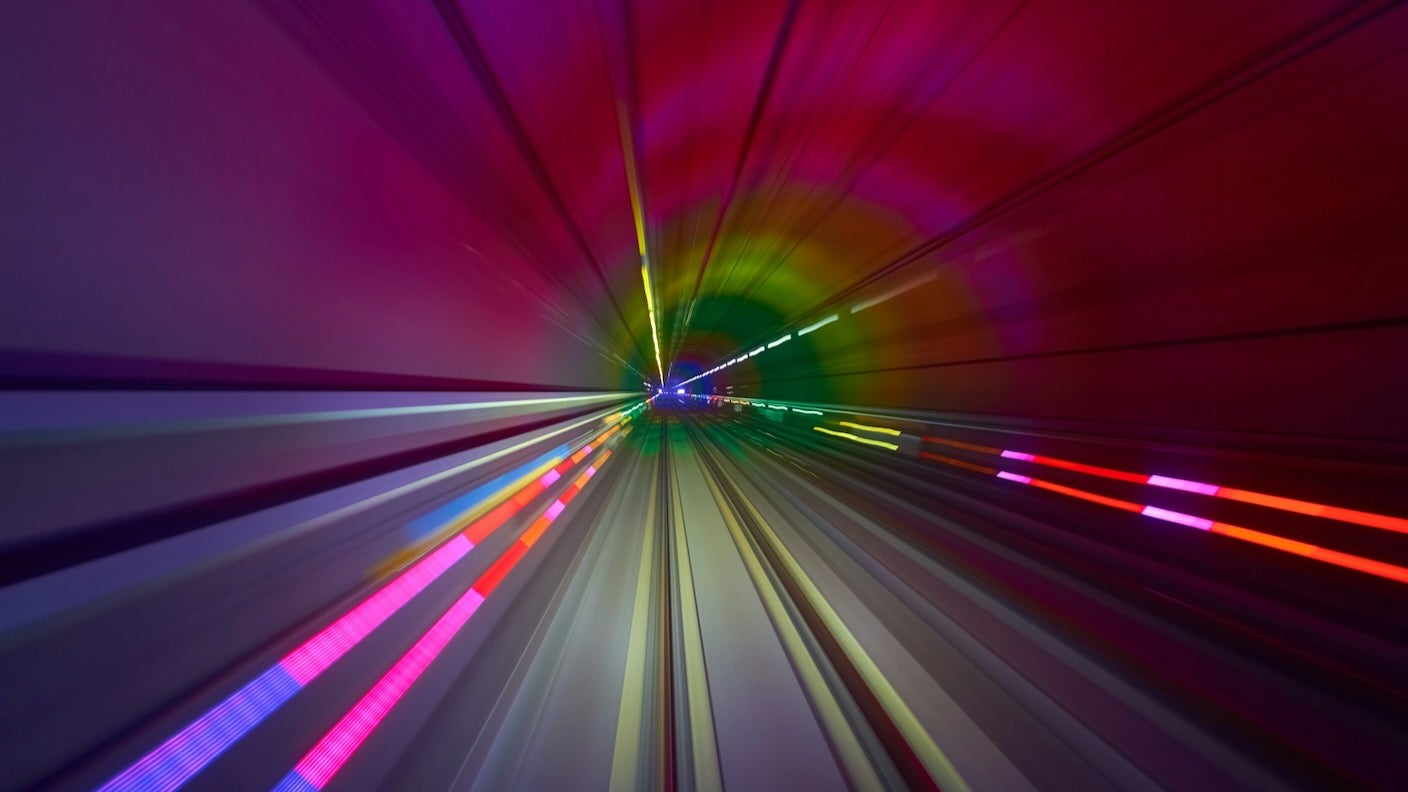
The Rise of Alternative AI Hardware
The Shift in AI Performance
Recently, the tech world has been abuzz over DeepSeek AI, which made headlines with its impressive performance while being cost-effective. This success has sparked interest in two innovative chip startups, Cerebras Systems and Groq, both of which are rising to the occasion with novel designs and capabilities.
Cerebras Systems is known for its extraordinarily large computer chips, which are about the size of dinner plates, and feature a unique architecture. In contrast, Groq specializes in producing chips specifically designed for large language models (LLMs). Both companies have demonstrated superior performance in processing tasks related to DeepSeek’s latest AI model compared to traditional methods.
Speed and Efficiency
Cerebras claims that its version of the DeepSeek AI can complete certain coding tasks in just 1.5 seconds, significantly faster than standard GPUs, which may take several minutes. According to Artificial Analysis, Cerebras’s chips have been measured to be 57 times quicker than their GPU counterparts in handling these tasks. Just when Cerebras seemed to lead the charge, Groq introduced a new product that surpassed Cerebras’s performance.
Despite the complexities behind DeepSeek’s advancements, the overall trend indicates that AI algorithms are becoming remarkably more efficient. While major companies like Nvidia continue to invest heavily in AI hardware, startups such as Cerebras and Groq are emerging as strong competitors, focusing on more efficient inference performance.
Understanding DeepSeek’s R1 Model
The Reasoning Capabilities
DeepSeek’s new AI model, known as R1, is described as a "reasoning" model. This distinguishes it from other models, like OpenAI’s, by allowing it to analyze problems systematically rather than providing immediate, unprocessed answers.
While this approach may seem trivial in casual conversations, it offers significant advantages for more complex tasks such as coding and mathematical computations, marking a step forward in AI technology.
Last week, the focus was on how R1 was not only economical to train—reportedly costing about $6 million—but also cost-effective to operate. Unlike traditional proprietary AI systems requiring hefty investments, R1’s open architecture invites wider collaboration and innovation.
Implications for the Market
This development has raised eyebrows among investors, suggesting a potential reduction in required funding for AI projects. Following this news, Nvidia suffered a noticeable dip in its stock price as concerns grew about its market dominance.
Innovative Chip Performance
Speed of Execution
The advancements aren’t limited to software; the underlying chips are also evolving to deliver better performance. For example, Groq, a startup founded by Jonathan Ross, the engineer behind Google’s AI chips, has been at the forefront of producing models tailored for LLMs. These chips have previously enabled instant responses, optimizing chatbot performance significantly.
However, the latest reasoning models intentionally take longer to generate answers. This process—known as "test-time compute"—involves generating multiple possibilities simultaneously, selecting the best response, and providing a rationale for it, thus enhancing the quality of answers.
Competitive Landscape
Cerebras, Groq, and other providers have recently collaborated to offer a simplified version of R1, known as Llama-70B, which maintains 70 billion parameters. Despite being smaller, this model has shown to outperform certain benchmarks when pitted against OpenAI’s counterparts.
Artificial Analysis also found that Cerebras’s optimized version of DeepSeek could produce around 1,500 tokens per second, eclipsing Groq and others, which managed significantly lower outputs. Though the smaller models might not equal the larger iterations pound for pound, they are demonstrating remarkable speed, especially in the realm of reasoning.
Fostering Greater Efficiency
Industry Trends
The recent developments highlight a growing movement towards enhanced efficiency in AI. Since OpenAI introduced its model, the company quickly transitioned to a newer version, and Google has been advancing its own reasoning models that closely follow DeepSeek’s efficiency metrics.
Meanwhile, major players in tech such as Google, Microsoft, Amazon, and Meta are set to invest $300 billion in AI data centers this year, indicating their commitment to scalable AI solutions. Other initiatives, such as OpenAI’s new data-center project named Stargate, further underline this trend.
The Road Ahead
Dario Amodei, CEO of Anthropic, notes a cyclical process where larger models lead to boosted capabilities, with companies refining these models over time—generating smaller, more efficient algorithms along the way.
This interplay between hardware and software advancements means a growing demand for AI chips. The shift towards more efficient, flexible models is paving the way for new applications in AI, potentially creating fresh opportunities and markets across various sectors.