Forget DeepSeek: The Cost of Large Language Models Continues to Decrease
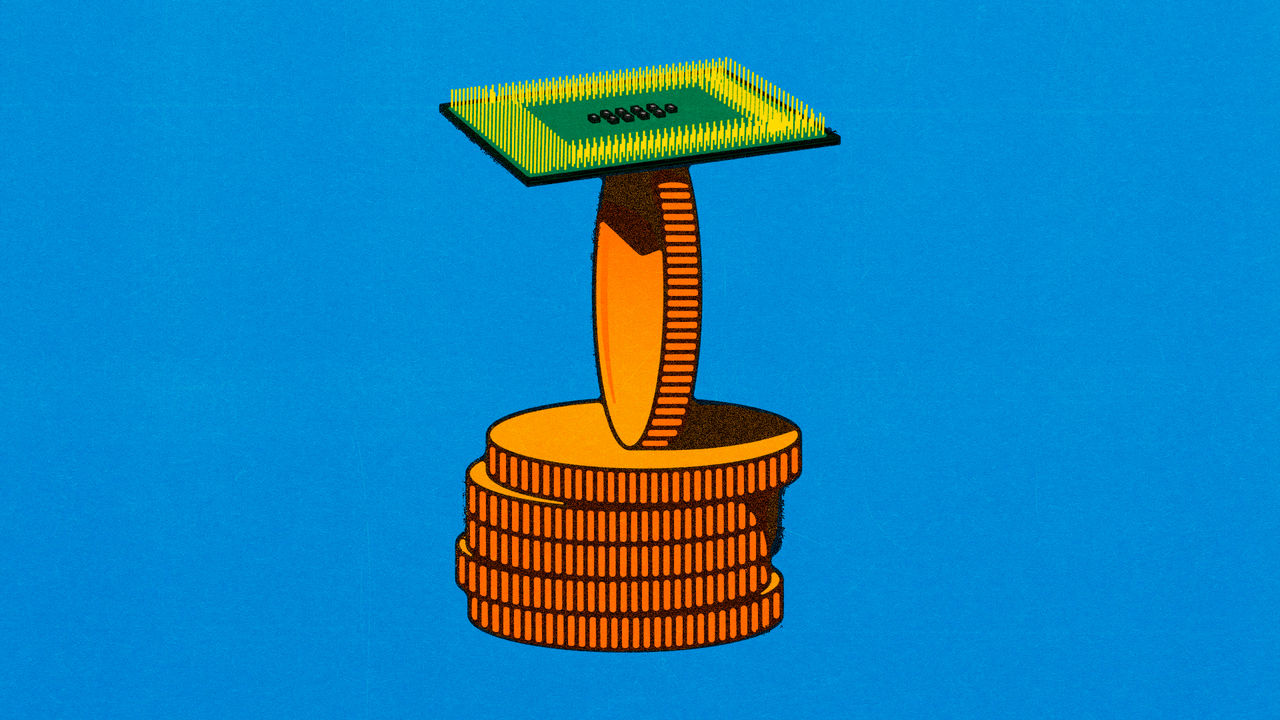
The Evolution of Large Language Models in AI
A Shift in Focus for AI Labs
As recent as 2022, creating a large language model (LLM) was considered a significant achievement in the field of artificial intelligence (AI). The landscape of AI engineering was dynamic, and innovations in LLMs provided a glimpse into a future filled with advanced capabilities. However, as we move further into 2023, the standards for what constitutes a groundbreaking achievement in this realm have changed. Nowadays, it’s not enough for AI labs to simply develop a high-performance model; they are also under pressure to do so at a lower cost.
The Growing Demand for Efficiency
Why Cost Matters
The surge in interest and investment in AI has led to numerous organizations entering the competition to develop LLMs. This explosion in the market has resulted in an increased focus on not just the quality of models but also the financial efficiency of their development. Companies now recognize that budget constraints can significantly impact their innovation potential. Thus, AI labs are challenged to balance performance with affordability.
So, What Defines a High-Quality LLM?
A high-quality LLM is characterized by several factors:
- Accuracy: It’s crucial for an LLM to produce reliable and coherent outputs based on the input it receives.
- Scalability: The model should handle various tasks and adapt to different datasets without significant degradation in performance.
- Efficiency: The computational resources required for training and deploying the model need to be optimized.
Strategies for Building Affordable LLMs
AI labs are now exploring various strategies to create LLMs that are not only robust but also cost-effective. Here are some effective approaches:
Open Source Collaboration
Increasingly, many organizations are turning to open-source frameworks to reduce development costs. Utilizing freely available tools and libraries allows for faster development cycles and broader collaboration among developers. This community-driven approach can also help in sharing best practices and innovations.
Leveraging Cloud Computing
Cloud platforms provide scalable infrastructure that can significantly lower the cost of model training and deployment. By using cloud services, organizations can access necessary computational power without having to invest heavily in their own hardware.
Focus on Transfer Learning
Transfer learning has emerged as a significant method for reducing the resource burden when developing LLMs. By fine-tuning pre-trained models on specific tasks rather than starting from scratch, developers can save time and computational resources while still achieving impressive results.
Enhanced Data Efficiency
Improved techniques in data selection and augmentation can streamline the training process. Efficiently using existing data and ensuring it is of high quality can contribute to the overall performance without incurring extra costs associated with acquiring large datasets.
Conclusion
In today’s competitive arena, AI labs are faced with the dual challenge of producing high-performing LLMs while also being mindful of their development costs. As the landscape continues to change, the focus on innovation in building efficient, high-quality models is likely to drive future advancements in artificial intelligence. As a result, organizations that master the balance between effectiveness and cost will stand out in this evolving field.