AI Has Evolved: Are Companies Claiming It Can Now ‘Reason’?
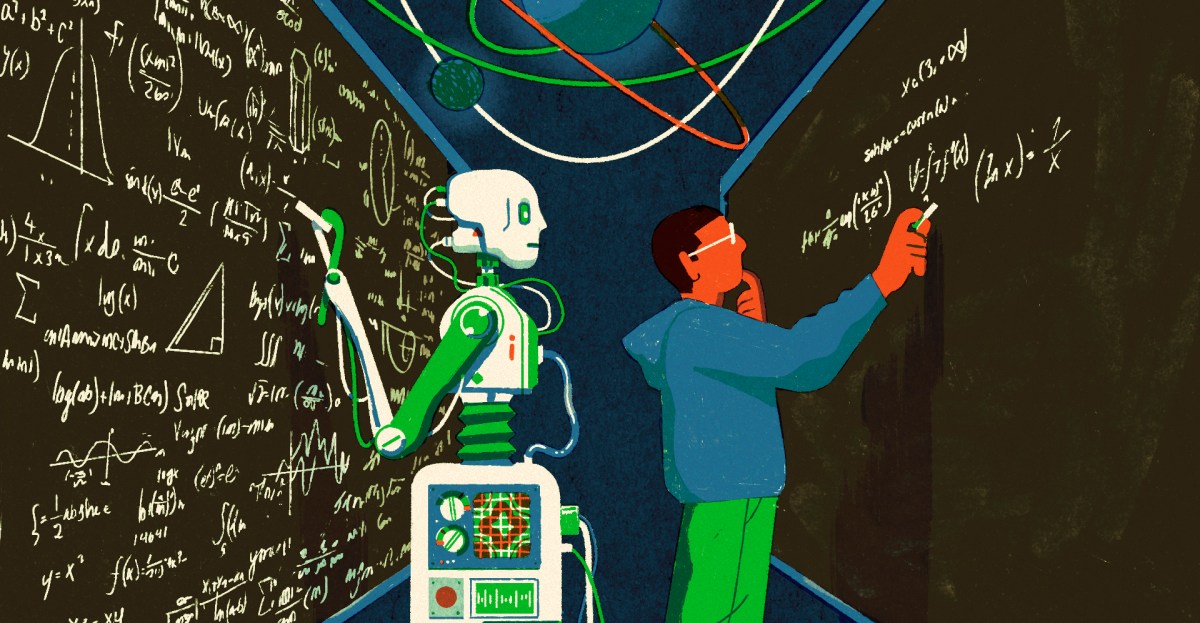
Understanding AI Reasoning: What It Means and Its Implications
Artificial Intelligence (AI) is evolving rapidly, and with each new model release, like those from OpenAI or companies such as DeepSeek, it’s easy to get overwhelmed. However, the central issue isn’t just about the latest features; it’s about whether these AI models genuinely possess reasoning capabilities similar to human thinking.
What Is Reasoning in AI?
At the core of the conversation about AI is the term "reasoning." Companies like OpenAI use this term to describe how their models can take complex problems, break them down into smaller parts, and tackle them one step at a time. This method is known as "chain-of-thought reasoning."
Different Types of Reasoning
Reasoning is not one single concept; it’s a mix of various types:
- Deductive Reasoning: Drawing specific conclusions from general statements.
- Inductive Reasoning: Making broad generalizations based on specific observations.
- Causal Reasoning: Understanding cause-and-effect relationships.
- Analogical Reasoning: Relating different situations based on similarities.
When we think through a challenging math question, for instance, most people do better when they can take their time and break down the problem, akin to AI’s approach.
Current Capabilities of AI Models
Recent models claim to show impressive skills, successfully solving tricky logic puzzles, passing math tests, and generating code without errors. Yet, they still struggle with simpler, more straightforward tasks. This mismatch leaves many AI experts questioning the true nature of AI reasoning.
Skeptics vs. Believers
The debate is polarized:
- Skeptics argue that what AI does more closely resembles imitation rather than authentic reasoning. They cite instances where models produce incorrect responses to simple problems as evidence that true reasoning is lacking.
- Believers, on the other hand, contend that, while AI reasoning may not yet match human flexibility, it is developing towards that goal.
The Debate Over Generalization
A significant factor in this discussion is whether AI can generalize knowledge the way humans do. Young children can learn rules from few examples and apply them to new situations, a skill that is still under scrutiny in AI systems.
Some experts caution that recent AI models might merely simulate reasoning. For example, philosopher Shannon Vallor describes this as "meta-mimicry," where AI seems to replicate human thought processes based on its training data without genuinely understanding.
Transparency and Computational Limitations
OpenAI’s models are increasingly complex but lack transparency about their inner workings. Research shows that AI often relies on generating intermediate steps or "filler tokens," which can mislead observers into believing it’s performing genuine reasoning.
Additionally, researchers have found that adding meaningless tokens can enhance problem-solving capacity without providing real reasoning.
Heuristics and AI Performance
Experts suggest that AI operates more like a collection of heuristics—shortcuts that help derive answers without deep thought—rather than through true reasoning. This thinking can sometimes lead to superficial success, where models perform well on specific tasks yet fall short in others.
The Spectrum of Intelligence
The phenomenon of "jagged intelligence" describes the uneven nature of AI capabilities. Modern AI models can excel at complicated tasks while struggling with simpler ones. It poses a stark contrast with human intelligence, which tends to be more consistent across various domains.
AI vs. Human Intelligence
In comparing AI to humans, it’s essential to understand that artificial intelligence should not be seen solely as smarter or dumber. Instead, viewing it as a different form of intelligence helps clarify its strengths and weaknesses.
Practical Applications of AI Reasoning
Understanding AI’s capabilities can guide how we leverage these tools effectively:
- Best Use Cases: Scenarios that require verification are ideal for AI assistance, such as code generation or data analysis.
- Cautious Usage: In more subjective areas like moral dilemmas, it is wise to treat AI as a supportive tool rather than a decisive authority.
By recognizing the areas where AI can help and where it struggles, users can make informed decisions about how to employ this technology responsibly.