Achieving Strategic AI Preparedness in Cybersecurity: Turning Aspirations into Action
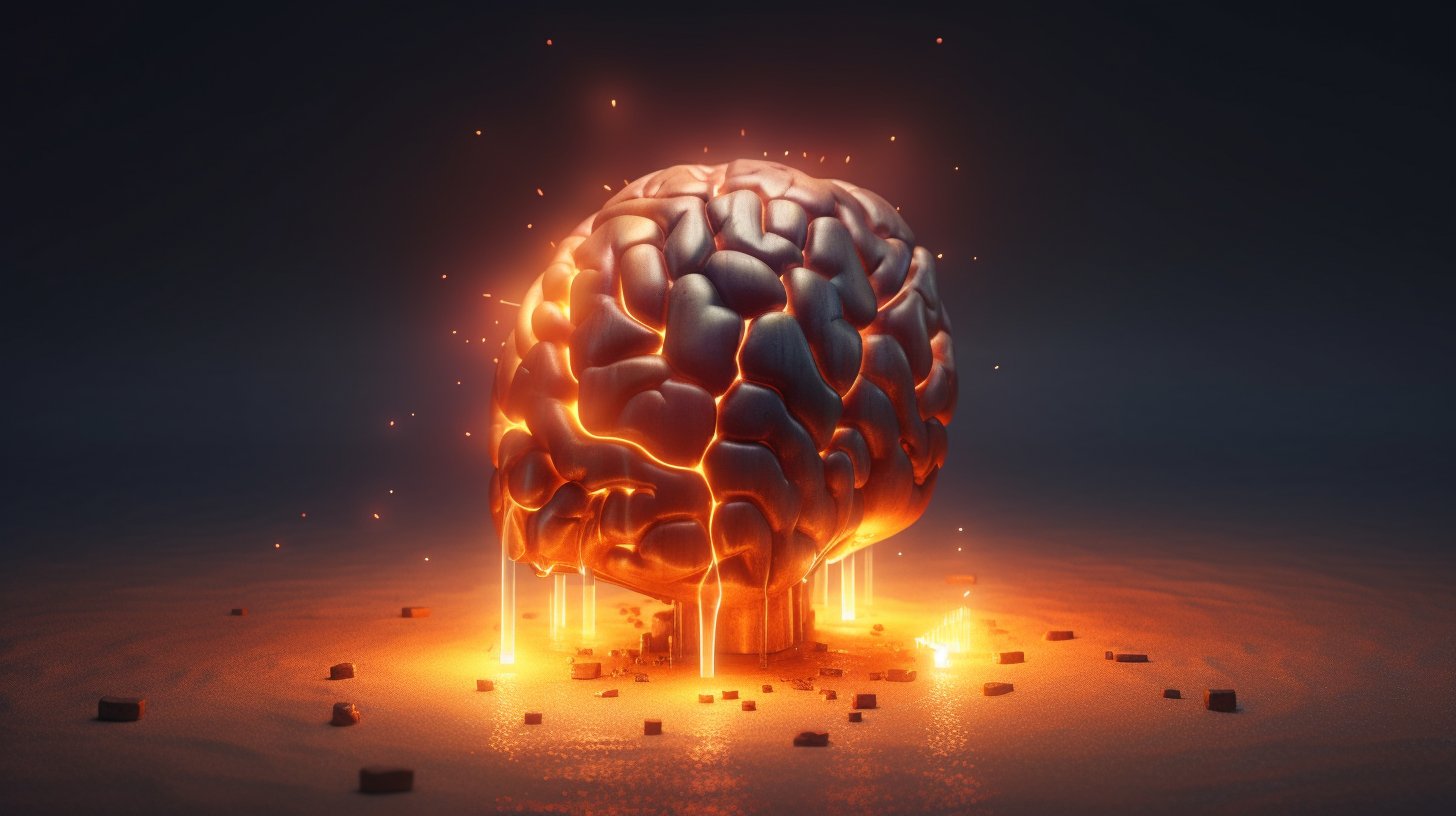
Understanding AI Readiness in Cybersecurity
Being ready to implement Artificial Intelligence (AI) in cybersecurity isn’t just about having the latest technology. Instead, it represents a crucial strategy for organizations. Without clear goals, adequate data preparation, and alignment with business needs, companies could face severe risks, such as an increase in sophisticated cyber threats. This underscores the importance of developing a solid framework to ensure AI is effectively integrated into cybersecurity practices.
What Does AI Readiness Entail?
The true advantage of AI in cybersecurity lies in its capacity to automate processes, predict threats, and enhance decision-making. As cyber threats grow increasingly complex, organizations can rely on AI to analyze network traffic patterns and identify unusual activities or predict possible attack methods based on past data.
When thoughtfully deployed, AI can significantly enhance an organization’s protection against threats, speed up incident response times, and bolster overall resilience. Here’s a breakdown of essential components necessary for a successful AI readiness framework in cybersecurity:
Key Components of an AI Readiness Framework
- Alignment with Business Goals: AI initiatives should not be based solely on trends. They must support specific business objectives that yield measurable results. Organizations need to concentrate on actual cybersecurity challenges and ensure that AI solutions fit seamlessly into existing operations.
Action: Clearly define how AI will be used to enhance cybersecurity measures, streamline operations, and improve threat response. Establish success metrics to evaluate the integration of AI into cybersecurity efforts, aligning these with broader corporate goals like cost control and compliance. Misalignment can lead to wasted resources and ineffective security measures.
- Data Quality and Availability: AI systems heavily depend on high-quality data to function correctly. Accurate and structured data from various sources, including network logs and user behavior, is crucial for effective threat detection.
Action: Establish a strong data governance strategy to ensure data accuracy, completeness, and the elimination of biases.
- Scalable Infrastructure for Secure Deployment: AI models demand significant computing power to analyze extensive data quickly and effectively. It’s essential that the infrastructure adheres to secure deployment principles.
Adopting a secure-by-design approach means embedding security measures into the infrastructure from the outset. This includes implementing principles like least privilege and network segmentation. A secure-by-default method ensures that necessary security features are automatically enabled, minimizing vulnerabilities.
Real-time operations are vital; AI systems must be capable of swiftly identifying and responding to threats.
Action: Utilize cloud AI solutions or flexible hybrid models that can adjust to fluctuations in network traffic and incidents while ensuring secure deployment protocols are followed.
- Ethical AI and Explainability: AI systems must operate ethically, especially in decision-making contexts like fraud detection. Being able to explain AI’s decisions is essential for maintaining trust and accountability.
Action: Adopt ethical and explainable AI frameworks to guarantee ethical data usage. This promotes transparency and the ability to audit AI responses to cybersecurity incidents.
- Continuous Learning and Adaptation: AI systems must continually evolve and learn from real-time data to stay ahead of emerging threats. Outdated models can fail to recognize new attack patterns.
Action: Implement an LLMOps framework combined with AIOps to ensure ongoing updates and retraining of AI models, allowing them to adapt to the latest cybersecurity threats.
- Collaboration Between Humans and AI: The ideal approach combines human intelligence with AI capabilities. AI can automate routine tasks, leaving cybersecurity professionals to focus on complex decision-making.
Action: Create workflows that foster collaboration between AI tools and cybersecurity experts, allowing for a more integrated approach to threat detection and response.
- Governance and Compliance: AI initiatives in cybersecurity must adhere to regulations such as GDPR and CCPA to ensure data privacy. Non-compliance can lead to serious legal and financial repercussions.
Action: Establish governance structures for AI that prioritize ethical usage, data privacy, and alignment with relevant regulations, ensuring proper oversight throughout the AI model lifecycle.
Building a Robust AI Framework
To harness the potential of AI in cybersecurity, organizations should adopt a holistic approach that considers data quality, effective governance, ethical practices, and collaboration. By addressing these critical areas, businesses can enable AI to enhance real-time threat detection, proactive responses, and adaptive defenses against complex and evolving security threats.