Determining the Right AI Solution for You Is More Challenging Than It Appears
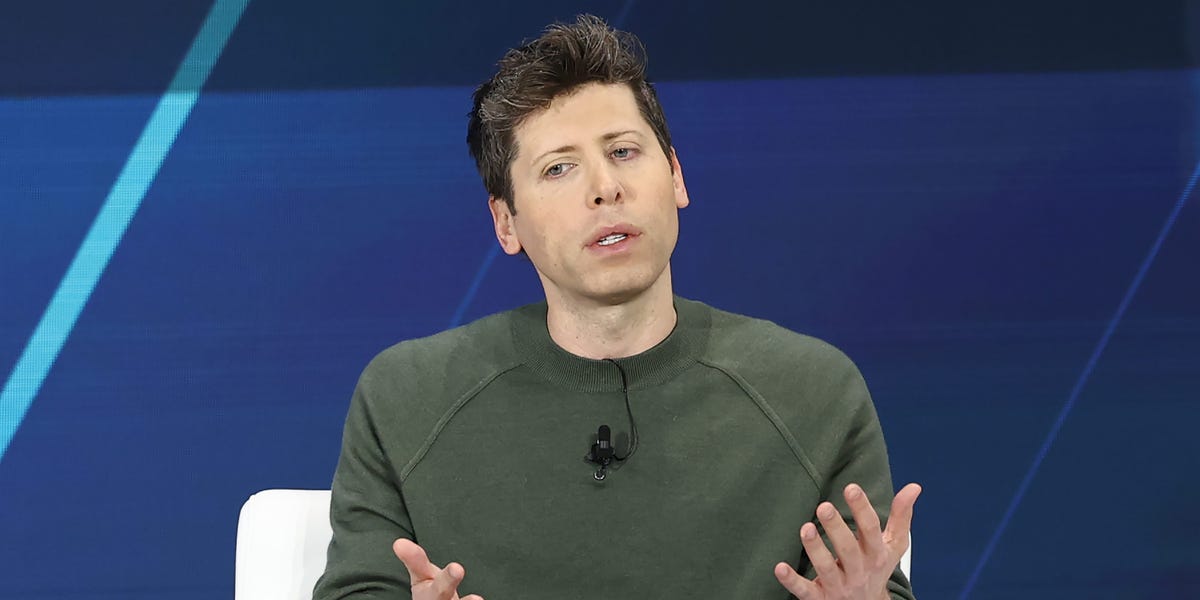
Navigating the Evolving Landscape of AI Models
The challenge of selecting the best artificial intelligence (AI) model for your needs can be quite overwhelming. Major players like OpenAI, Meta, Google, and Anthropic have introduced various models, including GPT-4 and its iterations, as well as several others. With many new releases appearing throughout the year, it might be hard to determine which AI has the leading edge.
Understanding the Benchmarking Challenge
Recent developments in AI have raised questions about the reliability of benchmark tests used to evaluate different models. These benchmarks often claim that new models outperform their predecessors, yet concerns have arisen regarding the validity of these comparisons. For example, Meta recently introduced new models in its Llama series, claiming better performance than similar models from Google and Mistral. However, accusations suggest that Meta manipulated the benchmark process.
An AI benchmark called LMArena, which collects user feedback on performance, indicated that Meta did not clearly communicate that it submitted a customized version of Llama for evaluation. Such incidents highlight significant issues within the AI benchmarking process.
The Systemic Flaws in AI Benchmarks
The AI sector invests billions into enhancing performance metrics, but this creates pressure on developers, leading them to tailor their training data specifically for these tests. Cognitive scientist Gary Marcus expressed that this trend could undermine the integrity of benchmarks, suggesting they may not truly evaluate what they purport to.
In a critical paper from the European Commission’s Joint Research Center, researchers identified "systemic flaws" in current AI benchmarking practices. They argued that many benchmarks prioritize state-of-the-art results over significant societal concerns.
Dean Valentine, CEO of AI security startup ZeroPath, echoed these sentiments, suggesting that recent AI model updates might not add significant value in real-world applications. According to him, while newer models may be engaging to interact with, they often fail to demonstrate substantial economic effectiveness.
Alternative Perspectives on Benchmarking
Nathan Habib, a machine learning engineer at Hugging Face, noted that many benchmarks traditionally skew towards user preference. This can incentivize developers to focus on making models “likable” rather than functionally effective. He advocates for essential safeguards in benchmarking practices, including updated data and transparent, independent evaluations. Habib mentioned the GAIA benchmark as an example of a tool designed to improve evaluation practices.
Despite acknowledging the imperfections of benchmarks, Habib emphasized that they still provide useful guidance on advancements in AI. Marcus further elaborated on the complexity of creating effective tests within the field, pointing out that many current tests are susceptible to manipulation.
Choosing the Right AI Model for Your Needs
With numerous models like DeepSeek-R1, Claude 3.7 Sonnet, and others available, it’s vital to approach AI model selection thoughtfully. Clémentine Fourrier, an AI research scientist, advised users to focus on models that specifically address their needs rather than solely chasing scores that do not align with their objectives.
She stated that finding a model that scores high concerning your particular requirements is far more valuable than merely searching for the highest-rated model overall.
In summary, as AI continues to evolve and an increasing number of models hit the market, understanding the benchmarks and performance claims is crucial for making informed choices that meet your unique needs. The field may be complicated, but with careful evaluation and consideration, you can discover the model that best suits your specific requirements.