Is Quantum-Inspired AI Capable of Competing with Modern Large Language Models?
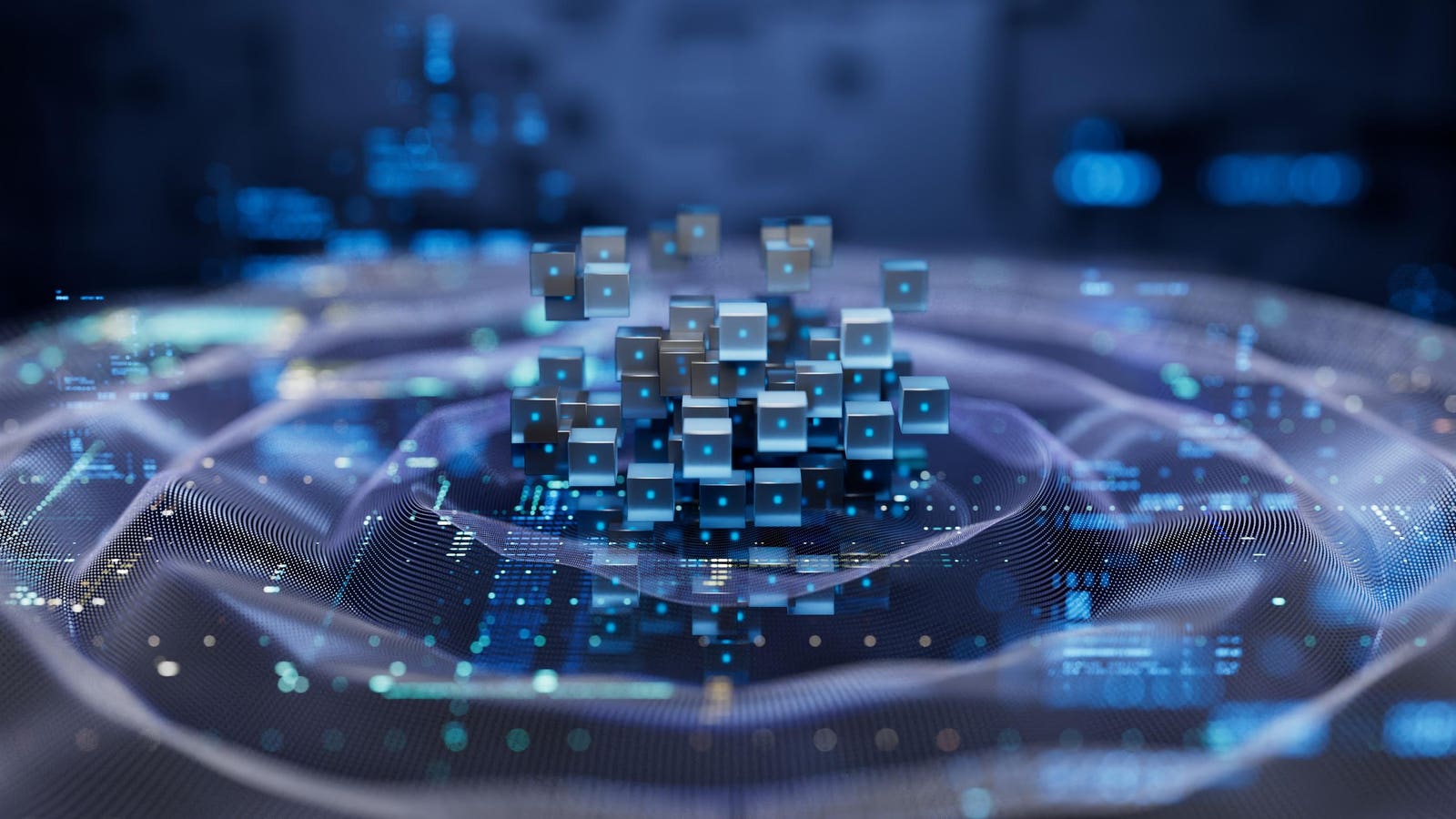
The Intersection of Quantum Computing and Artificial Intelligence
As the world of generative AI evolves, a fascinating development is occurring at the intersection of quantum computing and artificial intelligence (AI). Researchers and companies are starting to explore how quantum computing principles can tackle some challenges of current AI technologies, particularly in areas like scalability, efficiency, and reasoning complexity.
The Rise of Quantum Diffusion Large Language Models (qdLLM)
A noteworthy pioneer in this space is Dynex, a company based in Liechtenstein. They recently introduced their Quantum Diffusion Large Language Model (qdLLM), making headlines as a finalist in the SXSW 2025 innovation awards. Dynex claims that the qdLLM can generate AI outputs faster and more effectively than traditional AI models based on existing technologies.
Understanding Quantum Computing
What Sets Quantum Computing Apart?
Quantum computing operates differently from classical computing by utilizing quantum bits, or qubits. Unlike classical bits, which exist in one of two states (0 or 1), qubits can be in multiple states at once, thanks to a property called superposition. This enables quantum computers to process numerous possible solutions simultaneously, providing potential advantages in optimization, simulation, and pattern recognition tasks.
In the realm of AI, several research efforts are investigating how quantum features could improve areas such as natural language processing, machine learning optimization, and the efficiency of training models. Companies like IBM and MIT are looking into hybrid quantum-classical models to shorten training times for specific deep learning tasks, while start-ups such as Zapata AI are trying out quantum-enhanced models for tasks like sentiment analysis and forecasting.
The Innovative Design of Dynex’s qdLLM
A New Approach to AI Models
Dynex’s qdLLM differentiates itself by employing a diffusion model that allows for parallel token generation rather than the sequential token generation used by traditional models like GPT-4. Co-founder Daniela Herrmann explains that qdLLM mirrors human brain processing by handling multiple patterns simultaneously, rather than producing responses one word at a time.
This parallel processing approach is supported by research from various institutions, including Stanford and Google DeepMind, which are also probing diffusion-based transformers.
Integration of Quantum Annealing
A further distinction in Dynex’s model is its incorporation of quantum annealing. This technique is a type of quantum optimization that enhances the selection of tokens during text generation, potentially leading to improved coherence and reduced computational demands compared to standard language models.
Decentralized Emulation of Quantum Hardware
One of the standout features of Dynex’s model is its use of a decentralized GPU network that simulates quantum behaviors instead of relying on actual quantum hardware. This architecture can scale up to what Dynex claims could be one million algorithmic qubits.
Herrmann clarifies that the computations required for algorithms like qdLLM are run on a decentralized network of GPUs that efficiently imitate quantum calculations. This approach is somewhat similar to Google’s TensorFlow Quantum, which models quantum circuits on classical hardware.
Keeping an eye on the future, Dynex is also set to introduce its own neuromorphic quantum chip named Apollo by 2025. Unlike other quantum chips that necessitate extreme cooling, Apollo is engineered to function at room temperature, enabling it to be integrated into edge devices.
Enhancing AI Efficiency and Environmental Considerations
Dynex asserts that its qdLLM achieves significant reductions in model size, operates ten times faster, and utilizes only 10% of the GPU resources typically required for similar tasks. These claims are particularly relevant in the current climate of heightened scrutiny over AI’s energy consumption.
According to Herrmann, the combination of efficiency and the ability to perform tasks more quickly results in lower energy usage due to the reduced number of GPUs required. Though these claims await independent verification, similar initiatives from companies like Cerebras Systems and Graphcore show a broader trend toward more efficient AI workloads.
In performance evaluations, Dynex claims that qdLLM competes well against other leading models like ChatGPT and Grok, especially in tasks that require strong reasoning. Though detailed public benchmark data is not yet made available, the company plans to share comparative studies as they approach their 2025 launch goal.
The Future of AI and Quantum Computing
Dynex envisions a future where quantum computing plays a central role in the AI field. Herrmann predicts significant advancements within the next five years. While industry analysts from firms like McKinsey and Gartner suggest that quantum computing could enhance optimization and simulation tasks, widespread implementations may not emerge until after 2030.
In the meantime, Dynex is among a growing number of innovators exploring the possibilities of quantum-enhanced or quantum-inspired AI techniques. Their approach offers a glimpse into the ongoing search for foundational advancements in AI.