Google DeepMind Releases Paper Addressing Criticism of AlphaChip AI Chip Design Platform
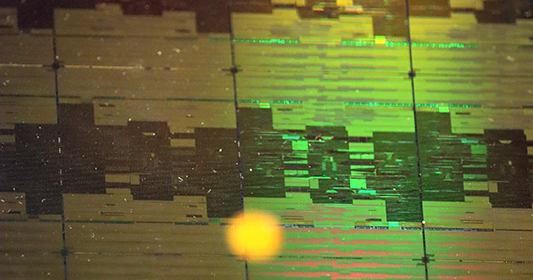
Google DeepMind’s AlphaChip: Addressing Controversy
Introduction to AlphaChip
Google DeepMind released a research paper aimed at countering criticisms concerning its AI chip design system called AlphaChip. First announced in 2020, this innovative reinforcement learning method focuses on designing chip layouts, particularly for Tensor Processing Units (TPUs). The findings were initially detailed in a 2021 paper published in Nature, which asserted that AlphaChip could significantly reduce the time and effort required for each new TPUs generation by thousands of hours.
How AlphaChip Works
AlphaChip employs a sophisticated reinforcement learning methodology to optimize chip layouts. By streamlining the design process, it not only saves time but also aids in creating more efficient hardware. Since its open-source release in 2022, the system has been utilized to improve Google’s Axion Arm-based CPUs and other internal chip projects yet to be announced.
Key Features of AlphaChip:
- Reinforcement Learning: Utilizes machine learning to adaptively improve chip layout designs.
- Open Source: Released to enable broader access for researchers and developers.
- Application Range: Used in various chip types including TPUs and CPUs.
Criticism and Controversies
Despite its innovative approach, AlphaChip faced skepticism in 2023. Two significant papers raised concerns about its effectiveness. One paper authored by Chang et al. reported difficulties replicating Google’s findings, while a meta-analysis by Igor Markov from Synopsys categorically labeled the technology a "false dawn."
Points of Criticism:
- Reproducibility Issues: Chang et al. claimed they could not reproduce the results presented by Google.
- Performance Analysis: Markov asserted that Google’s reinforcement learning (RL) methods fell short when compared to human designers and established algorithms like Simulated Annealing.
- Whistleblower Statements: Markov cited a Google whistleblower who alleged potential fraud related to the underlying research.
Google’s Response
In light of mounting criticism, Nature added an editor’s note to its original publication and began an investigation into the validity of Google’s claims. An expert who initially supported Google’s work later withdrew his commentary, adding further pressure on the tech giant.
Google countered the criticisms, stating that Chang’s research lacked necessary pre-training and computational resources. Additionally, they dismissed Markov’s allegations of fraud as unsubstantiated, asserting that an internal inquiry into the whistleblower’s claims found no evidence of wrongdoing.
Recent Developments
In September 2023, Nature concluded its investigation and reportedly sided with Google, lifting the editor’s note from their paper. Shortly thereafter, MediaTek announced plans to implement AlphaChip in its chip development processes, signaling renewed industry confidence in the technology.
However, Markov remained critical and published an updated analysis, stating that many concerns raised previously remained unaddressed. He claimed AlphaChip was not genuinely open-source due to missing key components necessary for reproducing results and the reliance on proprietary data.
Achievements and Future Directions
Despite the challenges faced, Google claims that AlphaChip has already changed the landscape for AI in chip design. According to their blog, the system has led to improved chip layouts, enhanced performance, and has even broadened its applications to other crucial aspects of chip design, such as logic synthesis and macro selection.
Advantages of AlphaChip:
- Accelerated Design Cycle: Reduces the time needed for chip development.
- Improved Performance: Claims of higher performance in chips designed using the system.
- Inspirational Impact: Sparked increased interest and development in AI-driven chip design globally.
In summary, while Google DeepMind’s AlphaChip has made significant strides in the field of chip design, ongoing discussions around its validity and efficacy illustrate the complexities of integrating AI into hardware development. The contrasting views present a window into the challenges and opportunities that AI technologies face as they become more prevalent in various industries.