Deepseek Unveils Innovative Technologies for AI
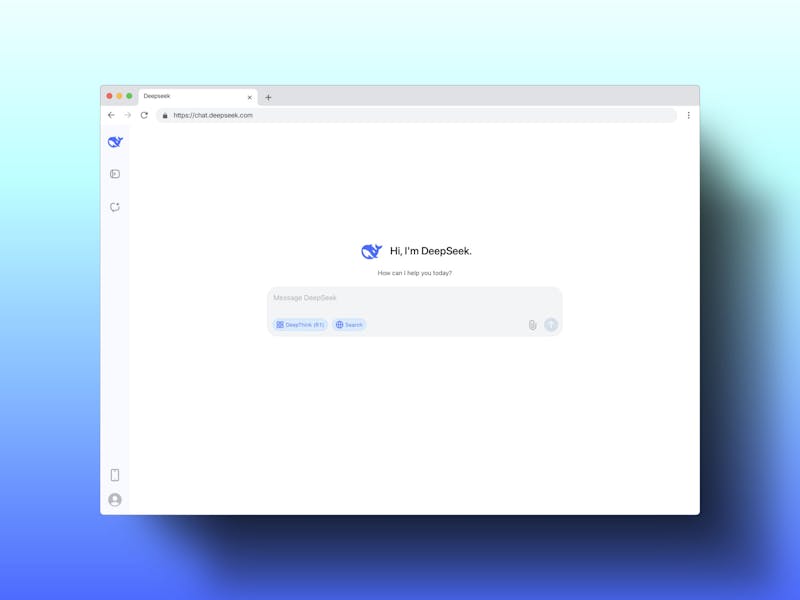
Introduction to Deepseek AI Models
On December 24, 2024, the Chinese AI company Deepseek launched its new large language model, Deepseek-V3, alongside Deepseek-R1—an AI model designed for complex logical tasks—on January 20, 2025. These models have become significant players in the AI landscape due to their impressive performance, which rivals that of existing giants like OpenAI, yet at a fraction of the cost.
Key Features of Deepseek Models
Performance Comparison
Assistant Professor Kangwook Lee from the University of Wisconsin-Madison compared Deepseek-R1’s functioning to OpenAI’s models, particularly highlighting its advanced reasoning capabilities. Both models by Deepseek consist of a massive 671 billion parameters, but there is a crucial distinction—only a fraction of these parameters are activated during processing. This selective activation greatly enhances efficiency.
Efficient Activation and MIxture-of-Experts Approach
Deepseek’s models utilize a sparse mixture-of-experts (MoE) architecture, diverging from the traditional dense approach that many AI models adopt. In a dense model, all parameters process each piece of data, while in an MoE setup, only specific parts (or "experts") are activated for different tokens. This method allows the models to operate more efficiently, as not every parameter needs to be used every time.
- Comparison of Dense vs. Sparse Approaches:
- Dense Model: Utilizes all components regardless of necessity.
- Sparse MoE Model: Activates a minimal set of parameters for each token, reducing computational load.
Lee explained that the MoE method is akin to using a circuit selectively: while a dense model engages the entire circuit for generating a token, the sparse model operates only with necessary components, thus optimizing processing time and efficiency.
Advancements in Deepseek Technology
Innovations in Resource Management
Deepseek also introduced innovations to enhance performance despite limited resources. For instance, the Multi-head Latent Attention (MLA) technique reduces data dimensions for more efficient processing. This attention mechanism allows the model to focus on different components of input data simultaneously, leading to improved results without compromising information quality.
Training Techniques
Moreover, Deepseek-R1 utilized Multi-Token Prediction, allowing the model to predict multiple tokens at once, unlike traditional models that only predict the next token sequentially. This approach ensures a more holistic understanding of contextual data.
- Differences in Training Methodologies:
- Traditional Models: Sequential prediction of tokens.
- Deepseek-R1: Simultaneous prediction of multiple tokens, improving contextual grasp.
Deepseek adopted an efficient Floating-Point 8 (FP8) mixed-precision training approach, which allows for the representation of model data in smaller bits. This method enables cost-effective data processing without sacrificing final accuracy, as opposed to conventional methods that use 16 or 32 bits.
Unique Model Construction
Deepseek’s architecture also showcases significant differences during both pre-training and post-training stages. In pre-training, large datasets—including text from various sources—are incorporated, leading to the model learning general data patterns. Post-training fine-tunes the model for specific user queries. Notably, Deepseek-R1 employs a method called long Chain-of-Thought, which conducts logical queries in sequential steps, improving its problem-solving ability.
Implications for AI Development
Deepseek’s innovative approaches have not only enhanced its models but have also set a precedent for future AI developments. The models invite collaboration and suggestions from the global developer community by being open-source, allowing improvements and adaptations to the underlying technology.
In the evolving field of AI, Deepseek’s advances—such as the MoE architecture, MLA strategy, and comprehensive training frameworks—have challenged the status quo of AI model training and efficiency. The development of robust, cost-effective model training could propel further breakthroughs in intelligent systems and applications.