Researchers Unveil Jumprelu Sparse Autoencoders from Google DeepMind: Achieving Cutting-Edge Reconstruction Fidelity
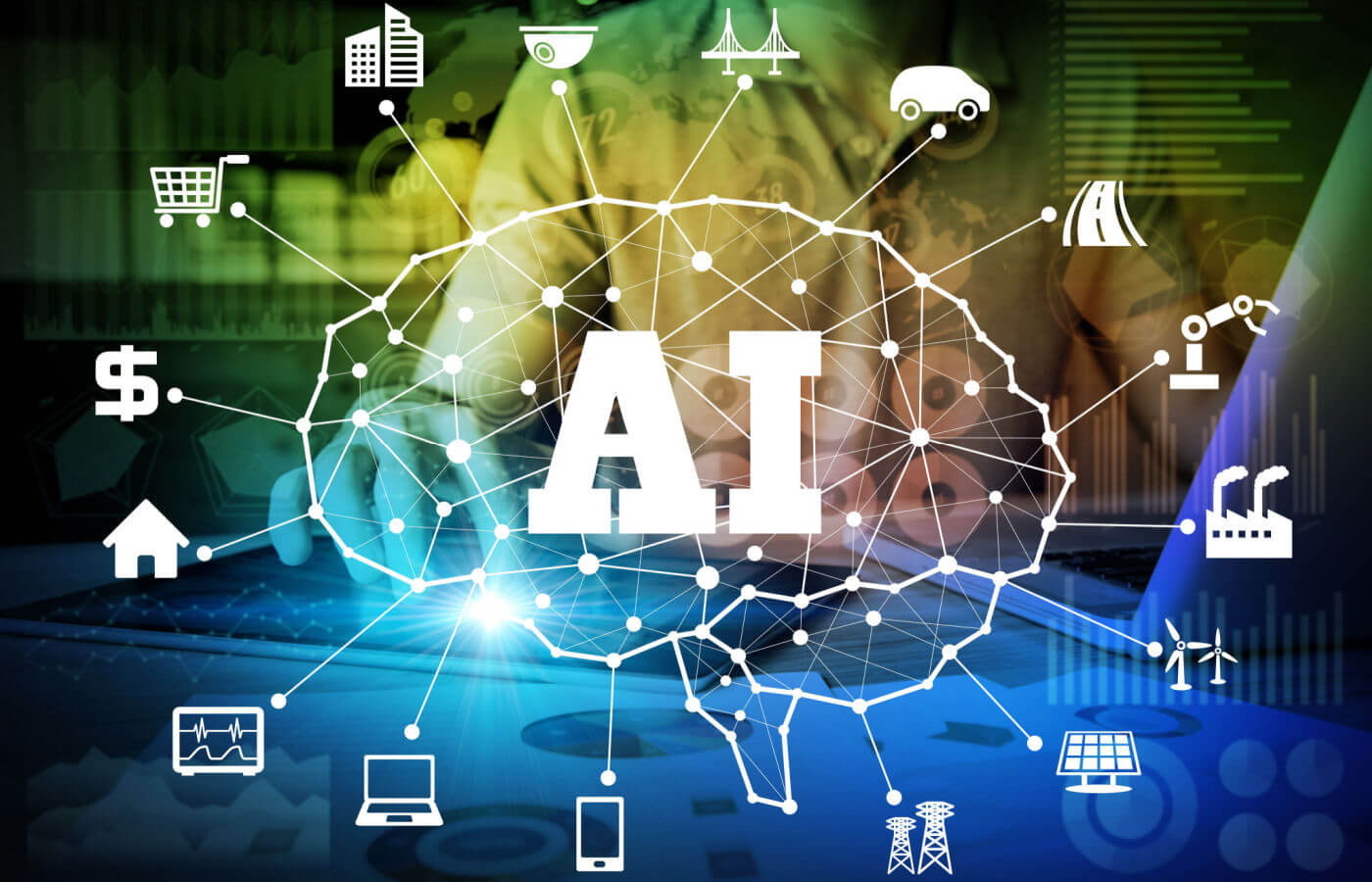
Google DeepMind’s Jumprelu Sparse Autoencoders
Google DeepMind has recently unveiled a groundbreaking innovation in the field of artificial intelligence known as Jumprelu Sparse Autoencoders. This new model stands out for its exceptional ability to achieve state-of-the-art reconstruction fidelity, marking a significant advancement in the capabilities of sparse autoencoders.
Understanding Sparse Autoencoders
Sparse autoencoders are a type of neural network architecture primarily used for learning efficient representations of data. They consist of an encoder that compresses input data into a compact latent space and a decoder that reconstructs the original data from this compressed representation. This technique is particularly useful in various applications such as image processing, signal recovery, and dimensionality reduction.
Key Features of Sparse Autoencoders
- Sparsity: This principle means that only a small number of neurons activate at any given time, making the model more efficient and capable of capturing significant features of the data.
- Reconstruction: The main goal of sparse autoencoders is to minimize the difference between the original and reconstructed data.
- High Performance: These models are known for their ability to produce high-quality outputs, which is crucial in applications where detail retention is essential.
Jumprelu Activation Function
One of the significant innovations introduced with Jumprelu Sparse Autoencoders is the Jumprelu activation function. This new activation approach enhances the model’s performance by allowing for improved gradient flow during the training process. By effectively managing the sparse activations, Jumprelu retains essential features while discarding irrelevant information, resulting in better performance during the reconstruction phase.
Advantages of Jumprelu
- Improved Gradient Flow: The Jumprelu function smoothens the optimization landscape, which helps in achieving faster convergence during training.
- Better Generalization: This function contributes to the model’s ability to perform well on new, unseen data, enhancing the model’s reliability in real-world applications.
Applications of Jumprelu Sparse Autoencoders
Jumprelu Sparse Autoencoders can be applied in a variety of fields, demonstrating their versatility and robustness in different scenarios:
- Image Compression: By effectively capturing critical visual information, these models can significantly reduce image file sizes without compromising quality.
- Anomaly Detection: In industrial settings and cybersecurity, they can identify unusual patterns or outliers in data, allowing for proactive measures.
- Data Imputation: These models can fill in missing values in datasets, improving the quality and usability of incomplete data.
Performance Metrics
In extensive evaluations, Jumprelu Sparse Autoencoders have shown remarkable results, achieving higher reconstruction fidelity than many existing models. Key performance metrics include:
- Mean Squared Error (MSE): A lower MSE indicates better reconstruction quality.
- Peak Signal-to-Noise Ratio (PSNR): A higher PSNR signifies less distortion in the reconstructed output compared to the original input.
- Structural Similarity Index Measure (SSIM): This metric evaluates perceived changes in structural information, which Jumprelu models have been shown to optimize well.
Future Implications
The launch of Jumprelu Sparse Autoencoders by Google DeepMind represents a pivotal moment in the development of AI models capable of complex data representation and processing. As industries increasingly rely on high-quality data representations, the advancements brought by Jumprelu could lead to enhanced performance in a myriad of applications, from healthcare to entertainment, showcasing the potential impact of AI on future technological innovations.
In summary, Jumprelu Sparse Autoencoders exemplify the cutting edge of AI technology, pushing the boundaries of what is possible in data reconstruction and representation. Their efficient design and groundbreaking activation function open up avenues for further research and application, indicating a promising trajectory for the future of artificial intelligence.