Clinical Trials for AI-Engineered Medications Expected in 2025
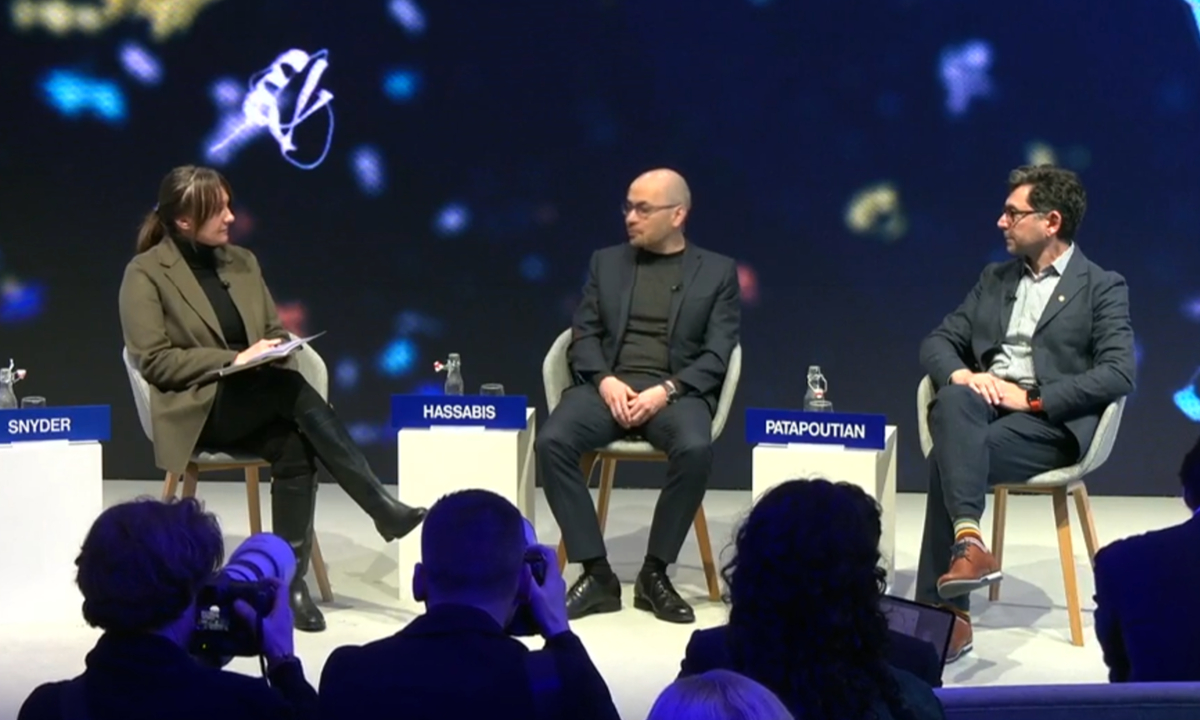
Demis Hassabis, the CEO of Google DeepMind and a Nobel Prize winner, recently spoke at the World Economic Forum in Davos, Switzerland. He shared exciting news about the future of pharmaceuticals, indicating that drugs developed with the help of artificial intelligence (AI) could begin clinical trials by the end of this year. This development comes from Isomorphic Labs, a for-profit branch established by Google’s parent company, Alphabet, in 2021, which aims to transform the drug discovery process using AI.
Hassabis emphasized that AI is much broader than the well-known large language models, extending into various scientific applications, including the groundbreaking AlphaFold. This AI model, which he co-developed, is capable of predicting the three-dimensional structures of proteins, addressing a significant challenge in biology that has persisted for decades.
Advancements in Protein Research
During the panel discussion, Ardem Patapoutian, a neuroscience professor and Nobel laureate, praised AlphaFold as “one of the most remarkable advancements in science.” He noted that previously it could take years for researchers to determine protein structures, but with AlphaFold, scientists can simply input a protein sequence to get its structure instantly.
AlphaFold has now predicted structures for approximately 200 million proteins, a task that would have traditionally required an estimated billion years of research. The upcoming AlphaFold3 version is anticipated to further enhance capabilities by analyzing how proteins interact with other proteins, ligands, and DNA/RNA, advancing our understanding of biological processes.
AI’s Role in Understanding the Brain
Patapoutian pointed out AI’s promise in unlocking the complexities of brain function. The current technology allows predictions in simpler organisms, yet comprehending more complicated brains poses a greater challenge. Despite extensive research, understanding how the brain works remains elusive, which is why many neuroscientists are excited about AI’s potential.
Patapoutian explained that predicting behavior in more complicated brains is still an unsolved puzzle in neuroscience. For simpler organisms, like the C. elegans worm with only 300 neurons, predictions may be feasible, but the complex networks of higher organisms present significant difficulties. He believes that the insights gained from AI will eventually lead to improved understanding of behavior, thought processes, intelligence, and consciousness.
The Evolution of AI and Drug Discovery
Hassabis mentioned that AI has indeed come full circle. The original inspiration of AI arose from concepts of how the brain functions, and now AI can contribute to deciphering that very complexity. He also discussed the future direction of AlphaFold3, particularly in relation to how genetic mutations can affect protein structures and functions. This understanding could pave the way for personalized medicine tailored to individual metabolic responses.
Future of Biological Research
Looking towards the future, Hassabis has a vision of creating a “virtual cell” simulation that could revolutionize biological research. Currently, determining a protein’s structure typically involves extracting it from a cell, which disrupts our understanding of its natural environment. By visualizing proteins within a whole cell, researchers can gain more detailed insights about their functions and locations.
Patapoutian stressed the value of this comprehensive view, highlighting that it could reveal specific activities occurring at the ends of neurons where proteins might be localized. This deeper understanding could lead to significant advancements in biology.
Data and the Path to Artificial General Intelligence
Questions arose regarding the data sources for training AI models. Hassabis explained that in addition to relying on public datasets, companies specializing in data generation can fill existing gaps. They also generate their synthetic data, and their goal is to develop algorithms that require minimal data to train. This approach mimics human learning—where we often learn effectively from just a few examples.
When asked about the timeline for achieving artificial general intelligence (AGI), Hassabis indicated that while some forecasts may be overly optimistic, significant breakthroughs are needed first. He identified two critical factors for AGI: developing reasoning and planning abilities akin to human thought processes and achieving true creativity, rather than simply replicating existing artistic styles or ideas. Hassabis believes AGI could be realized within the next five to ten years, provided these advancements occur.