The Impact of DeepSeek on Artificial Intelligence and Its Implications for Europe
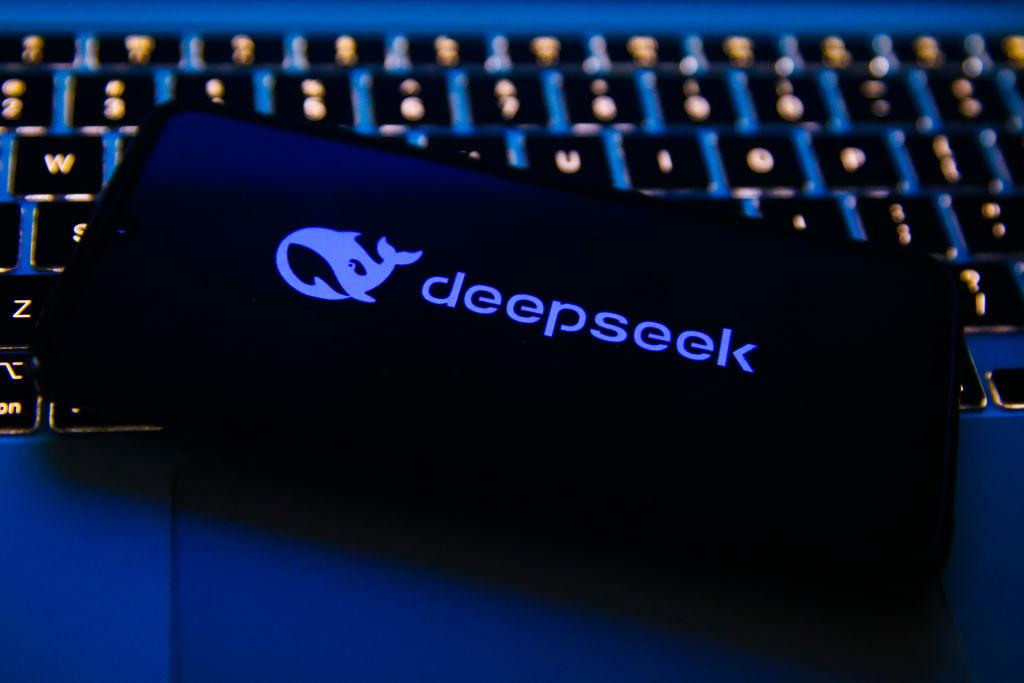
Understanding the Evolution of AI Language Models
Executive Summary
By mid-2024, advancements in artificial intelligence, particularly in large language models (LLMs), led to a realization of declining returns on training data and computational resources. This prompted a shift from expensive initial training processes to more affordable fine-tuning methods. Fine-tuning involved using Chain-of-Thought (CoT) datasets, which consist of structured questions and reasoning steps to arrive at answers. This approach not only enhances the efficiency of smaller AI models, such as DeepSeek, but also allows them to learn more quickly and adaptively from larger teacher models.
The financial implications of these changes are significant. The cost structure has transitioned from high pre-training costs to lower fine-tuning expenses for developers, while users now bear more inference costs. While small models like DeepSeek are cheaper to deploy, the demand for AI services is expected to grow significantly, potentially offsetting pricing declines and driving competition among AI firms.
1. Introduction: DeepSeek Emerges
In January 2025, DeepSeek’s AI model launch raised eyebrows as it appeared poised to disrupt the American AI landscape by providing a cost-effective alternative to larger models. Initial reactions in the market were enthusiastic, but it became evident that while DeepSeek introduced innovations, it conformed to existing AI model paradigms.
This article aims to explore these innovations critically, analyzing how they affect AI companies and regulators, particularly within the European Union. Although DeepSeek is considered innovative, it represents a continuation of existing trends rather than a groundbreaking shift.
2. Changes in AI Model Training
DeepSeek attracted attention toward the end of 2024 with its third version, which performed reasonably well on various metrics. The company leveraged a mixture-of-experts training strategy to overcome hardware limitations and develop a more efficient model without needing the most advanced computing resources.
Developers learned that their reliance on vast amounts of human-generated training data was unsustainable, leading to exploration of synthetic data. However, generating this data faced diminishing returns. A more effective alternative emerged through the use of CoT datasets, which document the reasoning process needed to derive correct solutions. This allowed models to undergo effective fine-tuning after their initial training without requiring extensive human feedback.
3. The Economics Behind Reasoning Models
Recent developments suggest that the emergence of smaller reasoning models, like those developed by DeepSeek, does not challenge foundational scaling laws of AI models. These smaller models benefit from reduced training costs while maintaining reasonable performance levels when compared to their larger counterparts. Nevertheless, the claim that they eliminate the need for infrastructure development, particularly concerning training data, remains contentious.
Despite a shift toward CoT datasets aiding in operational efficiency, these models still trail behind the most advanced systems. The inevitable transition towards longer reasoning periods during inference adds a layer of complexity, necessitating enhanced computational resources while making models more cost-effective for users.
4. Economic Impacts on AI Development
The evolution from intensive pre-training to more practical fine-tuning signals a broader economic transformation for AI model developers. Costs historically tied to extensive initial training have been reduced, leading to an increase in recurring expenses at the inference stage, where models interact with users.
This shift has implications for how AI companies structure their services and pricing. The reduced costs for users of smaller models result in their wider application, driving demand. Yet, pricing strategies must cleverly encompass both operational expenses and the amortization of costs associated with model development.
Competition remains fierce, forcing developers to bundle their models with additional services to remain financially viable. However, the growing trend of knowledge extraction among models complicates the sustainability of business models, as unrestricted access can lead to potential underpricing across the industry.
5. The Role of AI Development in the European Union
Unlike large tech firms in the U.S. with substantial resources and existing infrastructures, EU businesses face unique challenges in developing AI capabilities. Notably, numerous investments have been announced, indicating a growing ambition among European firms to establish a competitive footing in AI infrastructure.
Despite this, the dependency on larger AI models for foundational knowledge persists, complicating the landscape for smaller AI entities that wish to thrive without significant capital investment. The evolving cost structures present an opportunity for European developers to create specialized applications on established models, fostering innovation while carefully navigating competitive pressures.
6. Future Directions
The ongoing evolution in AI model training signifies a transition where larger generative capabilities exist alongside smaller, specialized applications. The emphasis on fine-tuning and efficient reasoning aligns with a broader move toward more accessible AI solutions that can promote productivity across various sectors.
For policymakers, this presents a delicate balance: support competitive innovation without stifling investment incentives for foundational AI models. Ultimately, the AI landscape’s future will hinge on how effectively these challenges are navigated, allowing the EU to embrace innovative pathways while leveraging the growing intelligence of new AI technologies.