Achieving India’s DeepSeek Vision: Adopting the Right Approach and Mindset
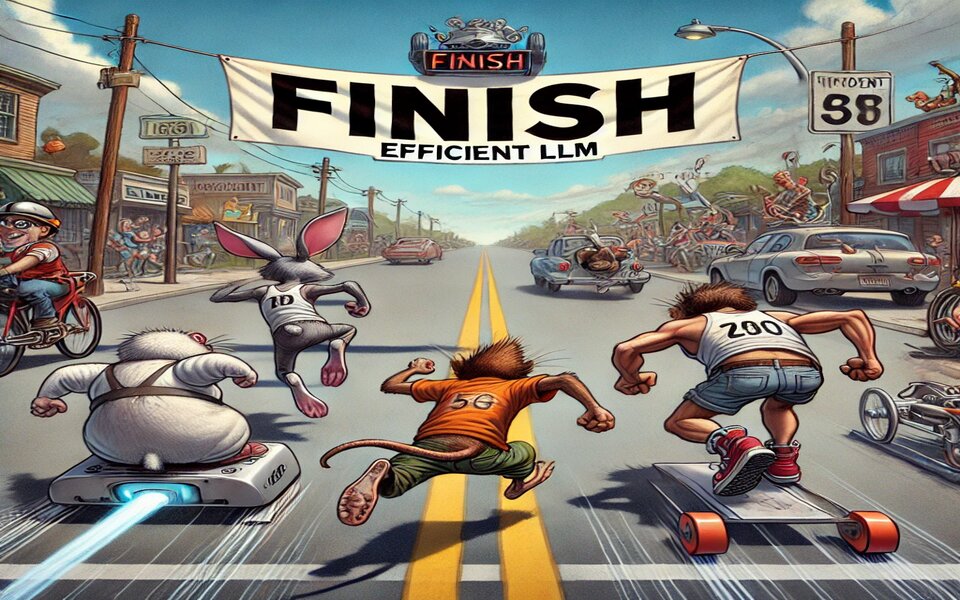
The Evolution and Implications of Large Language Models
Overview of Large Language Models
Recent analyses reveal that a staggering 33,000 large language models (LLMs) have been developed. A pivotal moment in the journey of LLMs occurred on January 10, 2025, termed the DeepSeek moment. This event surprised major tech companies including OpenAI, Google, Meta, and Anthropic. Initially, these companies dismissed the innovation, attributing it to advancements in China, but later acknowledged its significance.
A question has since emerged: Should India create its own foundation model? The Indian government, through the Ministry of Electronics and Information Technology (MeitY), aims to have its own foundation model by the end of 2025. However, the lack of widespread enthusiasm raises questions: Are we too late to appreciate the changes brought about by DeepSeek, which, in turn, has also faced disruptions?
Understanding AI Progress
The history of artificial intelligence (AI) is marked by periods of rapid advancement followed by stagnation, often referred to as "AI winters." Currently, we are in a fascinating phase where AI models are becoming increasingly efficient at a rapid pace. Efficiency in AI is primarily determined by two key factors:
1. Cost of Compute and Storage
The cost of generating a million tokens of output has plummeted from over $60 for OpenAI’s ChatGPT 3 series to approximately $0.14 with DeepSeek in just two years. This significant reduction is noteworthy; however, it should not be mistaken for an overall decrease in the computational resources required. NVIDIA’s CEO, Jensen Huang, indicated that the computing demands have surged by a factor of 100. Hence, lower costs per output unit may not necessarily translate to overall expense reductions.
2. Cost of Accuracy
DeepSeek has specifically targeted the cost of accuracy, making strides in reducing computing costs. Experts assert that model accuracy can be enhanced through three methods: pre-training, post-training, and time-test scaling. Previous models mainly focused on costly pre-training, which demanded extensive data and computational resources. In contrast, DeepSeek’s approach integrates post-training techniques, allowing for iterations that refine output quality without significantly inflating costs. Time-test scaling is still in a testing phase.
DeepSeek’s Strategy
DeepSeek optimizes costs through several key strategies:
The Foundational Aspect of DeepSeek
The inception of the first GPT model in 2017 laid the groundwork for subsequent advancements, with its release in 2018 based on 7,000 books. This sparked interest from Lian Wenfeng, a Chinese algorithmic trader and founder of High-Flyer Quantitative Investment Management. With a solid educational background in electronic information and communication engineering, Lian’s expertise in algorithms positioned him as a pioneer in leveraging technology for AI advancements.
India’s Prospects
India has a formidable advantage, thanks to its robust pipeline of STEM graduates. Nonetheless, there are concerns about whether these graduates pursue careers in science and math.
1. Seizing Opportunity Costs
Lian Wenfeng systematically collected financial data for algorithmic trading before establishing High-Flyer AI to advance AI research. By 2019, he had acquired 10,000 A100 GPUs before U.S. sanctions against China, setting the stage for DeepSeek’s development.
In India, the IndiaAI mission plans to procure 18,000 GPUs under a $1.3 billion initiative to foster AI research and development.
2. Leveraging Local Talent
One of DeepSeek’s secrets to success was effectively engaging local talent. Lian employed graduates from diverse backgrounds, including humanities, to reverse-engineer advanced LLMs. This approach fostered innovation through a collaborative mindset.
In contrast, while India has one of the largest pools of AI talent, reliance on the concept of jugaad (a flexible approach to problem-solving) may hinder more systematic solutions to existing data challenges.
Moving Forward
While the complete costs associated with DeepSeek remain unclear, its meticulous planning led to substantial advancements in accuracy and efficiency. For Indian LLM developers, DeepSeek serves as a vital case study in enhancing model training techniques by exploring reinforcement learning and other innovative methods.
According to Nasscom’s report, India already has over 17 LLMs in operation. By optimizing existing foundational models, India can aim for a comprehensive, multi-functional AI model suitable for varied applications.
The true potential lies in harnessing India’s linguistic diversity and unique societal factors, which could lead to rich data insights and experimentation. With AI evolving rapidly, capturing and democratizing this data will be essential to keep pace with the global AI landscape.