AI Leaders Claim Cancer Solutions: The Truth Behind the Promises
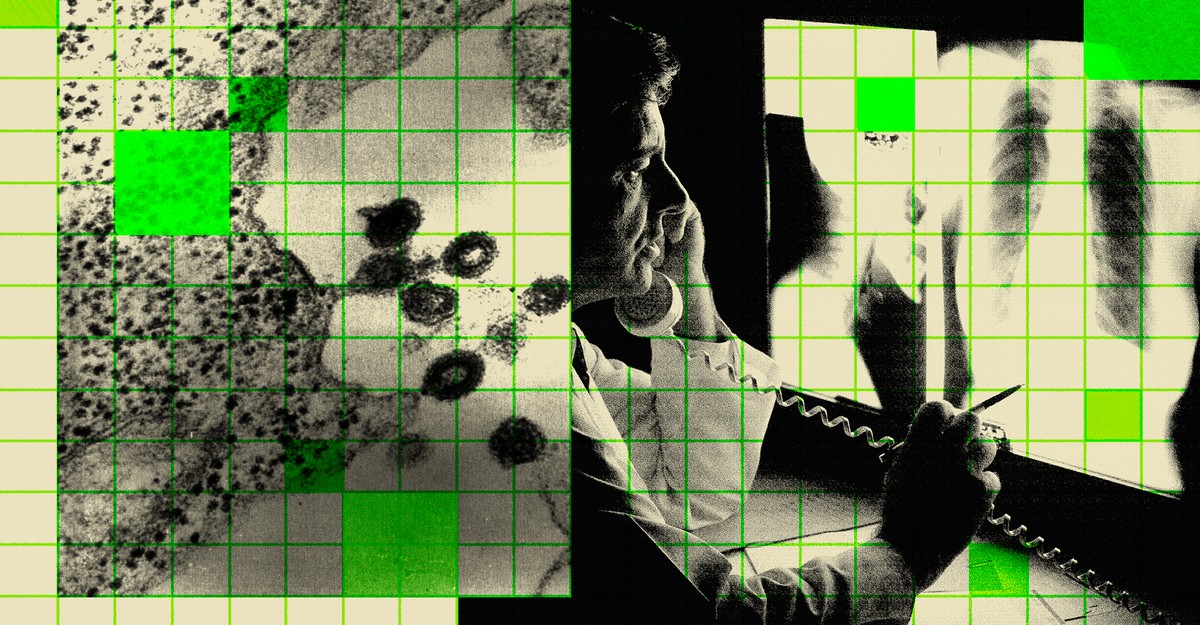
The Role of Generative AI in Healthcare Research
Introduction to AI in Medicine
Generative AI has recently taken center stage in discussions surrounding the future of healthcare and disease treatment. Many leaders in Silicon Valley have expressed optimism about AI’s potential to revolutionize medical research and claims that it could help eliminate diseases in a matter of years. Demis Hassabis, CEO of Google DeepMind and Nobel laureate, anticipates that AI could address significant scientific challenges, including curing various diseases within a decade. Similarly, Sam Altman, CEO of OpenAI, has stated that AI is expected to facilitate unprecedented rates of disease cures.
The Current Landscape of AI in Life Sciences
While the excitement around generative AI is palpable, it’s essential to understand the technology’s actual capabilities and limitations. The application of AI in healthcare is primarily occurring in two ways:
Information Processing: One type of generative AI functions like an advanced search engine, helping researchers analyze scientific literature and generate useful reports. Current models, such as ChatGPT and Google’s research products, allow scientists to streamline their work by quickly summarizing vast amounts of information.
- Biological Analysis: The second type focuses on analyzing biological data, like the three-dimensional structures of proteins. Tools like AlphaFold and other generative AI systems help identify patterns in data, which can expedite drug discovery and development.
Limitations of AI in Drug Development
Despite the hopeful rhetoric, experts warn about the overestimation of AI’s immediate impact on healthcare. For example, any promising drug suggested by AI would still need extensive laboratory tests and clinical trials before it can be deemed safe and effective. Alex Zhavoronkov, CEO of Insilico Medicine, explains that while AI can help design drugs, the actual process is lengthy, with various regulatory hurdles to overcome.
While some companies, such as Insilico, have successfully utilized AI to develop drugs that cleared initial human trials, the conversation often overlooks how long it takes to bring a drug to market or validate a treatment.
AI’s Achievements and Future Potential
Generative AI has already made contributions to science that cannot be overlooked. For instance, employments of AI at companies like Pfizer have led to identifying potential targets to combat diseases like breast and prostate cancer. Nevertheless, experts concur that while AI may facilitate significant advances in research, its role might be more about enhancing existing human research rather than providing groundbreaking discoveries.
Key Applications of AI
Drug Design: AI can help scientists efficiently analyze drugs’ molecular structures and their interactions. This process can dramatically narrow down the number of configurations researchers need to investigate, streamlining the pursuit of new treatments.
- Knowledge Recommendations: AI tools can also recommend knowledge and insights derived from existing literature, aiding researchers in generating hypotheses and building their studies.
The Partnership of Human and Artificial Intelligence
An important aspect of using AI effectively in research is the human element. Good scientists must validate AI outputs to ensure accuracy. Andrea Califano from Columbia University shared an instance where an AI tool provided incorrect references for a research paper, illustrating how caution is necessary when using such technology.
Moreover, innovative models like Google’s "AI co-scientist" aim to enhance the research process by generating and assessing hypotheses similar to a team of scientists. This approach highlights the potential of AI to assist human researchers rather than replace them, serving as a tool that can speed up scientific progress.
Challenges in Utilizing AI in Science
The most significant challenges include the need for high-quality training data and the limitation of AI systems in providing original ideas. Experts emphasize that AI must rely on properly curated data, as its intelligence is only as good as the data it processes. José Penadés from Imperial College London noted that while AI could generate hypotheses fast, verifying and validating these ideas remains where human expertise is irreplaceable.
Ultimately, the path forward for integrating AI into life sciences will likely require humans to ask the right questions, manage data wisely, and perform the essential experimental validation that AI cannot yet accomplish. As scientists and AI systems collaborate more intensely, generative AI’s role in healthcare will likely evolve, potentially leading to significant advancements in disease treatment and prevention.