Alibaba Unveils Open Source Qwen3, Surpassing OpenAI’s O1
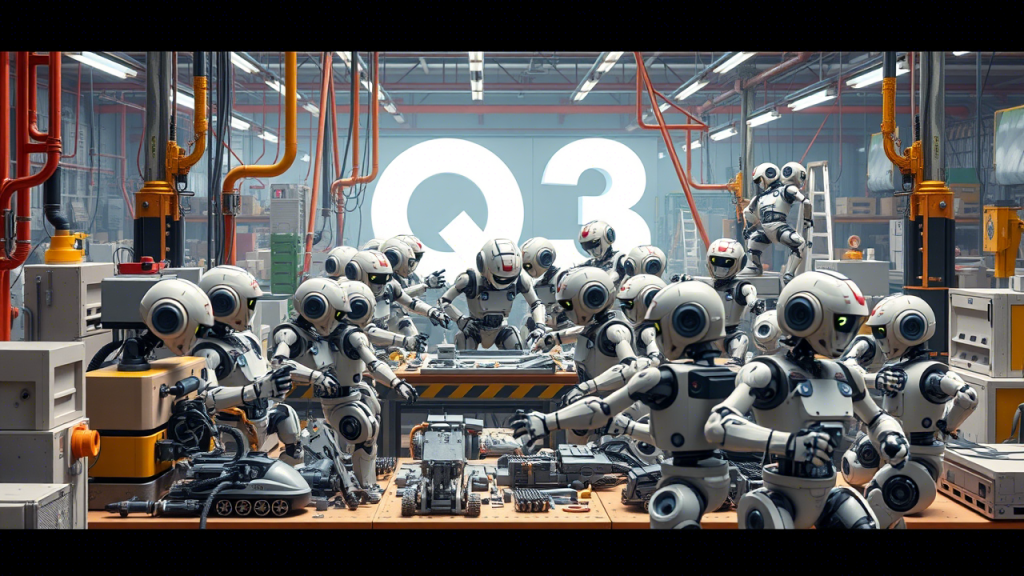
Alibaba’s Qwen3: A Major Leap in AI Language Models
Alibaba’s Qwen team has recently unveiled the Qwen3, a groundbreaking series of open-source large language multimodal models. These models are showing promising results, rivaling proprietary models from well-known players such as OpenAI and Google.
Overview of Qwen3 Models
The Qwen3 series consists of eight models, which include two mixture-of-experts (MoE) models and six dense models. The innovative mixture-of-experts approach integrates various specialized model types into a single framework. Only the relevant models are activated based on specific tasks, which enhances efficiency in resource use. This method gained popularity thanks to companies like the French AI startup, Mistral.
The standout model in this series is the Qwen3-235B with the codename A22B. This model has demonstrated superior performance compared to notable competitors, including DeepSeek’s R1 and OpenAI’s o1, in independent benchmark tests. It achieves remarkable results in areas such as software engineering and mathematics, even nearing the capabilities of the cutting-edge Google Gemini 2.5-Pro.
Key Features of Qwen3
Advanced Reasoning Capabilities
One of the most notable features of Qwen3 is its capacity for hybrid or dynamic reasoning. This allows users to switch between quick, accurate responses and more complex, time-consuming reasoning processes. Users can activate a "Thinking Mode" via a simple toggle on the Qwen Chat interface or by entering specific prompts during model deployment. This flexibility caters to various query complexities, making it especially useful for detailed inquiries in sciences and engineering.
Multilingual Support
Qwen3 significantly expands its multilingual capabilities, supporting 119 languages and dialects from various major language families. This development broadens the potential applications of these models globally, facilitating their use in diverse linguistic environments.
Model Architecture and Training
The Qwen3 models are built on an enhanced training framework that marks a considerable advancement from their predecessors. The training dataset has been drastically increased to around 36 trillion tokens, sourced from web crawls and other documents. This extensive data collection allows the Qwen models to perform proportionally better than previous versions, even when the latter were much larger.
The training process consists of a rigorous three-stage pretraining followed by a four-stage post-training refinement. This method is designed to bolster both the hybrid reasoning capabilities and overall model performance.
Deployment and Accessibility
Qwen3 models offer users a range of deployment options. They can be integrated into various frameworks, including SGLang and vLLM, which provide OpenAI-compatible endpoints. For local installations, tools like Ollama and MLX are recommended, allowing users to utilize Qwen models directly.
Additionally, the Qwen-Agent toolkit simplifies operations involving tool-calling functionalities, enhancing usability for developers working with these advanced AI models.
Implications for Enterprises
For decision-makers in the tech industry, the introduction of Qwen3 marks a change in how they can leverage AI. Existing OpenAI-compatible endpoints can be connected to Qwen3 in a matter of hours, significantly reducing implementation time. Also, various MoE model checkpoints provide notable reasoning prowess at a fraction of the cost typically associated with larger models.
Moreover, the Apache 2.0 license accompanying Qwen3 allows organizations to use the models freely for commercial purposes, though they should investigate any legal implications that may arise from utilizing a model developed by a China-based firm.
Future Directions with Qwen
The Qwen team is not resting on its laurels. Future plans involve scaling up both data and model sizes, developing even more robust reasoning capabilities, and expanding the types of tasks that models can undertake effectively. The overarching goal is to approach Artificial General Intelligence (AGI), gradually building towards providing even more sophisticated AI solutions.
Ongoing competitions in the AI landscape underscore the significant demand for increasingly capable, efficient, and accessible models. The advancements seen in Qwen3 enhance its potential to meet these growing needs, making it a valuable tool for researchers, developers, and enterprises aiming to innovate with cutting-edge AI technology.