Assessing the Precision and Dependability of Large Language Models in Responding to Item-Analyzed Multiple-Choice Questions on Blood Physiology
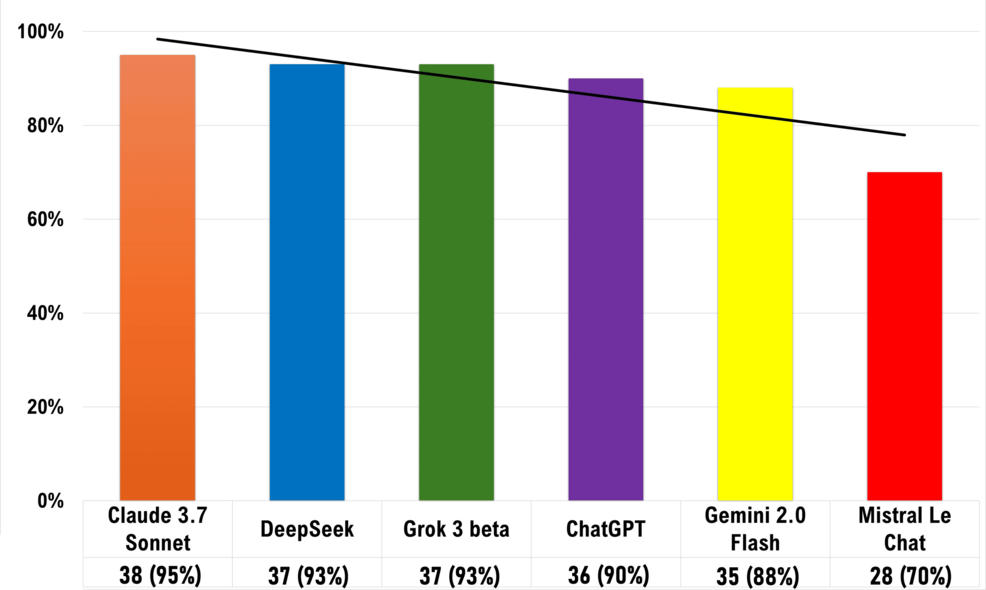
Introduction to Large Language Models in Medical Education
Large language models (LLMs) like ChatGPT, Claude, DeepSeek, Gemini, Grok, and Le Chat have garnered attention for their potential uses in various fields, including medical education. One of the vital areas of evaluation is how accurately these models can respond to specific queries, particularly item-analyzed multiple-choice questions that focus on blood physiology. This article delves into the evaluation of these models regarding their accuracy and reliability in responding to such questions.
Understanding Blood Physiology
What is Blood Physiology?
Blood physiology is the study of the components, functions, and processes of blood in the body. Blood plays numerous critical roles, such as transporting oxygen and nutrients, regulating body temperature, and protecting against pathogens. Understanding blood physiology can help in diagnosing and treating various medical conditions.
Importance of Accurate Responses
Accurate answers in medical education, particularly regarding blood physiology, are essential for students and professionals. Incorrect or misleading information can lead to misunderstandings and hamper learning. Therefore, evaluating LLMs’ responses to multiple-choice questions (MCQs) is crucial for their potential application in medical education or exam preparation.
Evaluating Large Language Models
Framework for Evaluation
To assess the accuracy and reliability of LLMs in answering MCQs about blood physiology, researchers often adopt a systematic approach. Here are some key elements of this evaluation:
- Question Selection: Carefully curated questions focused on various aspects of blood physiology.
- Response Analysis: Responses generated by LLMs are compared against established medical knowledge and expert opinions.
- Scoring Mechanism: Each response is rated for accuracy based on predefined criteria, typically using a scoring system that measures correctness and relevance.
Models Under Review
The evaluation focuses on a few notable LLMs:
- ChatGPT: Developed by OpenAI, known for its conversational capabilities and quick responses.
- Claude: From Anthropic, designed with a focus on safe and reliable interaction.
- DeepSeek: Aimed at specialized queries, including medical and scientific questions.
- Gemini: A newer model with advancements in contextual understanding.
- Grok: Known for its succinct responses and clarity.
- Le Chat: Emphasizes conversational learning and user engagement.
Findings on Reliability and Accuracy
General Performance Insights
Recent studies have revealed varying levels of competence among these LLMs when handling medical MCQs. Common findings include:
- High Variability: Different models demonstrate varying accuracy rates across different question categories, with some models excelling in straightforward factual questions while struggling with complex scenarios.
- Contextual Understanding: Models that better grasp context tend to perform better on nuanced questions that require more than mere recall of facts.
- Limitation Awareness: Many models perform well within their training data but struggle with questions outside that scope, emphasizing the importance of ongoing training and model updates.
Examples of Evaluation Outcomes
- Simple Recognition Questions: Most models accurately identified facts about blood composition and functions.
- Complex Application Questions: While some models provided decent explanations, inaccuracies often arose when questions required the application of knowledge to hypothetical scenarios.
- Critical Thinking Queries: Models struggled most with questions needing critical analysis or multi-step reasoning.
Implications for Medical Education
Potential Uses in Learning Environments
Despite the limitations, LLMs offer several opportunities for enhancing medical education:
- Supplemental Learning Tools: They can serve as additional resources for students, helping clarify concepts.
- Preparation Tools: LLMs can be harnessed for practice quizzes, creating an interactive way for students to assess their understanding.
- Assessment Tools: Their use in exam-like settings can help identify areas where students may need further study.
Future Directions
As technology advances, ongoing research and development will be crucial. Improvements in accuracy, context handling, and broader training data can increase the reliability of LLMs in medical fields. Understanding their strengths and limitations will allow educators to integrate these tools effectively into curricula, enhancing the learning experience for students pursuing medical proficiency.