ByteDance Enhances AI Reasoning with DeepSeek through Intern-Led Open-Source Initiative
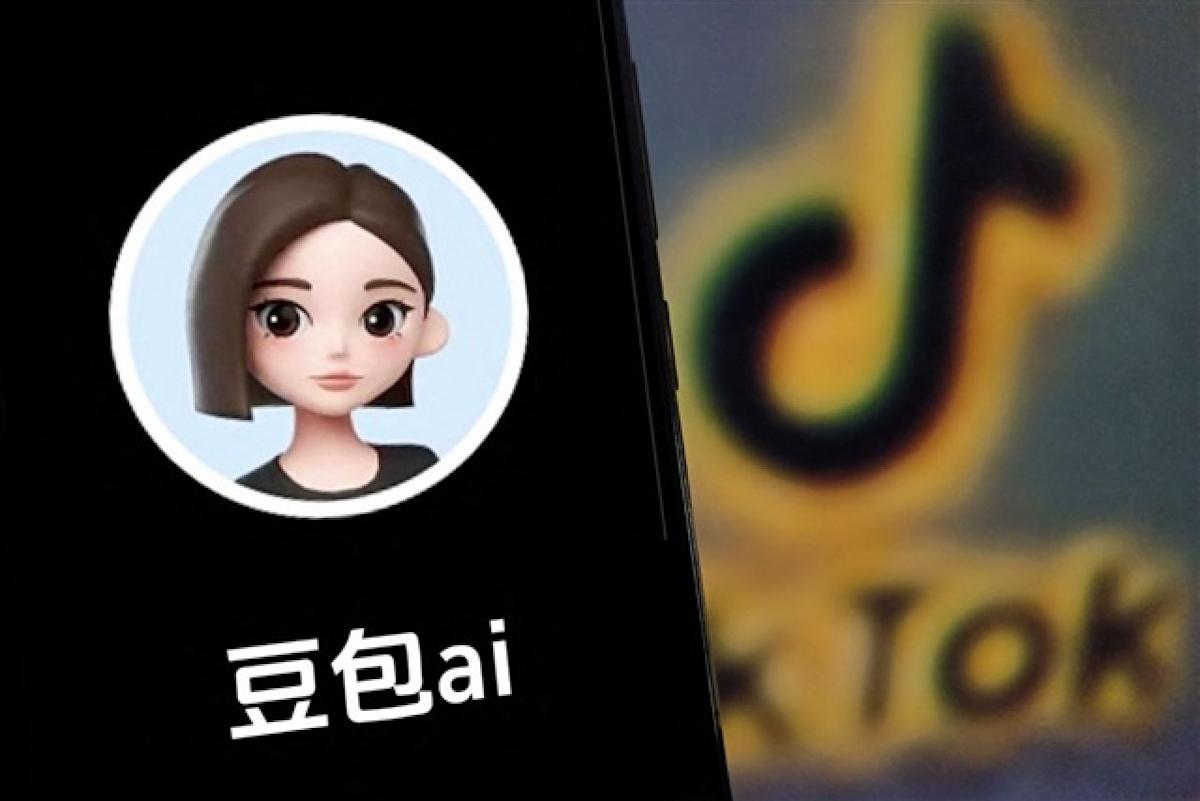
ByteDance Introduces DAPO: A New AI Training System
ByteDance, the parent company of TikTok, has made significant strides in the field of artificial intelligence (AI). Recently, they launched DAPO, a new system designed to enhance the capabilities of large language models (LLMs) in complex reasoning tasks. This innovative system builds upon the work of DeepSeek, a previous AI model focused on training reasoning algorithms.
What is DAPO?
DAPO stands for Decoupled Clip and Dynamic Sampling Policy Optimisation. It employs a scalable reinforcement learning algorithm that is aimed at improving the complex reasoning functions of LLMs. According to a research paper by ByteDance in collaboration with Tsinghua University’s Institute for AI Industry Research, DAPO achieves tasks like self-verification and iterative refinement more efficiently.
The DAPO system has shown notable success, outperforming DeepSeek’s R1 reasoning model. The algorithm recorded an impressive score of 50 points on the American Invitational Mathematics Examination (AIME) 2024, using Alibaba Group’s Qwen2.5-32B base model. In comparison, DeepSeek’s R1 model secured only 47 points while utilizing the same foundational model.
Key Features of DAPO
- Efficiency: DAPO achieved superior results with 50% fewer training steps than the previous models.
- Scalability: The algorithm is designed to be scalable, making it applicable for a wide range of AI applications.
- Collaborative Research: The project showcases a collaborative effort in the AI community, offering insights into training methodologies.
Comparison with DeepSeek
Notably, the findings revealed that DAPO provides better performance compared to DeepSeek’s training techniques, specifically the group relative policy optimisation (GRPO). This feedback was emphasized by Philipp Schmid, an engineer from Google DeepMind, who acknowledged DAPO as a significant step forward in reinforcement learning.
However, the study’s authors highlighted that when applying GRPO, they lagged behind DeepSeek by 17 points on the AIME, indicating that certain critical training data may have been overlooked in the original R1 research. They have also suggested four new techniques aimed at further improving performance over DeepSeek.
Industry Reactions
The introduction of DAPO has been met with approval from various academics and industry professionals. Arpit Sharma, the head of the ecosystem at Aethir, noted the importance of such transparency and collaboration among researchers. On the other hand, some experts raised questions regarding the relevance of comparing training steps, with Nvidia’s senior research scientist, Vitaly Kurin, suggesting that fewer training steps don’t necessarily indicate a reduction in overall training time.
The Team Behind DAPO
The DAPO project is spearheaded by Yu Qiying, an intern at ByteDance and a doctoral candidate at Tsinghua University, along with Tsinghua undergraduates and PhD candidates. This development aligns with ByteDance’s strategy to attract talented AI professionals early in their careers. They have recently announced a recruitment campaign targeting research interns with a passion for technology, particularly from universities like Tsinghua and others worldwide.
Future Directions
ByteDance continues to strengthen its focus on AI development, as demonstrated in a recent internal meeting led by co-leaders Zhu Wenjia and Wu Yonghui, both formerly with Google. Their discussions included plans to explore the limits of AI intelligence and to enhance open-source initiatives, showcasing ByteDance’s commitment to innovation in the artificial intelligence sector.
ByteDance’s advancements with DAPO represent a significant milestone in AI research, promoting new methods and fostering collaboration within the industry while drawing attention from other tech giants and academic institutions alike.