DeepMind Advances Weather Forecasting with Remarkable Accuracy
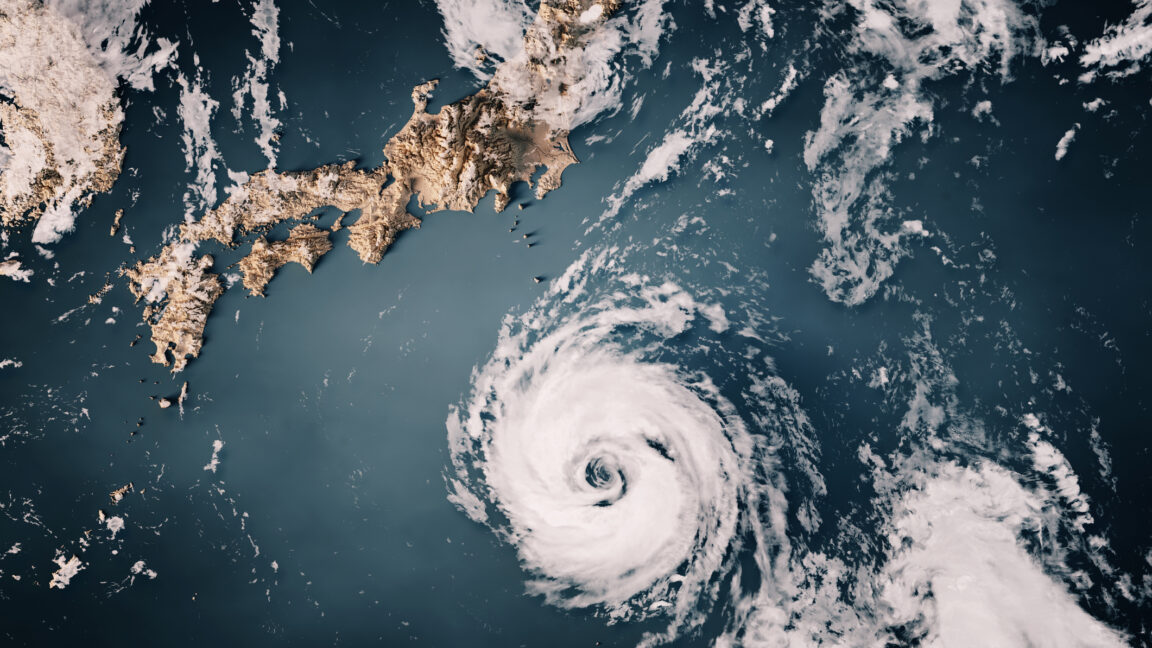
AI in Weather Forecasting
Recent advancements suggest that AI systems have begun to rival traditional computing methods for creating weather forecasts. However, there is a trade-off: while AI tends to penalize deviations in its training, this leads to less precise predictions over longer time frames. As you look further ahead, forecasts get “blurry,” making it harder to pinpoint specifics like storm paths, which may become wider and less defined.
The Appeal of AI
Despite these challenges, the use of AI for weather forecasting presents a compelling option. Conventional atmospheric models are remarkably accurate but require extensive computational resources. The European Centre for Medium-Range Weather Forecasts is widely regarded as a leading example of such effective modeling.
In a new development, Google’s DeepMind has introduced an AI system called GenCast that claims to outperform the European model in terms of forecasts that extend to at least a week, and often even longer. GenCast merges the computational techniques used by atmospheric scientists with diffusion models utilized in generative AI, achieving high-resolution forecasts while significantly cutting down on computational requirements.
Understanding Ensemble Forecasting
Traditional weather forecasting methods have two primary advantages over AI systems. Firstly, they are grounded in atmospheric physics, which utilizes concrete scientific principles to determine weather behavior based on real-world data. Secondly, these models generally run in ensembles, where multiple simulations are performed. This method captures the inherent unpredictability of the weather, providing crucial insights into forecast uncertainty.
Efforts to combine traditional weather modeling with AI have been made in the past. For example, a Google initiative involved an atmospheric model that utilized a grid-based system but relied on AI for predictive analytics within each grid cell. While this approach demonstrated improved computational efficiency, it resulted in relatively large grid sizes and lower resolution.
DeepMind’s approach with GenCast skips some of the traditional physical modeling in favor of the ability to operate an ensemble forecast system.
GenCast’s Mechanism
GenCast employs diffusion models, which are trained to adapt existing weather data by starting with a blend of original weather patterns and variations infused with noise. The goal is to generate variations that closely resemble the original patterns. Once trained, the system can take pure noise as an input and manipulate it into realistic weather data based on current atmospheric conditions and historical trends. The model progresses by taking 12-hour steps, meaning forecasts for later days will rely on past conditions and earlier forecasts.
This method allows for generating ensemble forecasts by introducing different initial noise patterns, leading to diverse weather outputs. This mimics the uncertainty assessments found in traditional weather models.
GenCast analyzes six surface weather parameters, six atmospheric states, and data from 13 altitudes that track air pressure. The resolution of each grid square is 0.2 degrees, providing finer details than the European model. DeepMind asserts that a single instance of GenCast can run for up to 15 days in just eight minutes using Google’s tensor processing systems.
Performance Evaluation
DeepMind’s research indicates that GenCast significantly surpasses traditional weather models. According to a benchmark assessment, GenCast proved more accurate than the European model in 97% of tests, which measured various output values over differing timelines. The confidence ratings derived from the ensemble were generally valid and useful.
Historically, AI weather forecasting systems have struggled with extreme weather predictions due to limited training data on rare events. However, GenCast performed well in predicting unusual temperature and air pressure events (with occurrences below 1%).
Advanced Testing and Results
GenCast also underwent rigorous testing to evaluate its applicability, including predicting the paths of tropical cyclones. For the first four days of these forecasts, GenCast outperformed the European model, maintaining its superior accuracy for about a week. An intriguing test involved using GenCast to forecast wind power potential based on data from the Global Powerplant Database. This forecast showed that GenCast outperformed traditional models by 20% in the first two days and consistently maintained an advantage for up to a week.
DeepMind acknowledges that while GenCast shows remarkable results, further investigations will delve into understanding why performance may diminish gradually after a week. Today’s findings solidify the potential of combining traditional forecasting models with AI elements to enhance accuracy, hinting at a future where innovative forecasting approaches can coexist.