DeepMind AI Achieves Superior Weather Prediction Accuracy
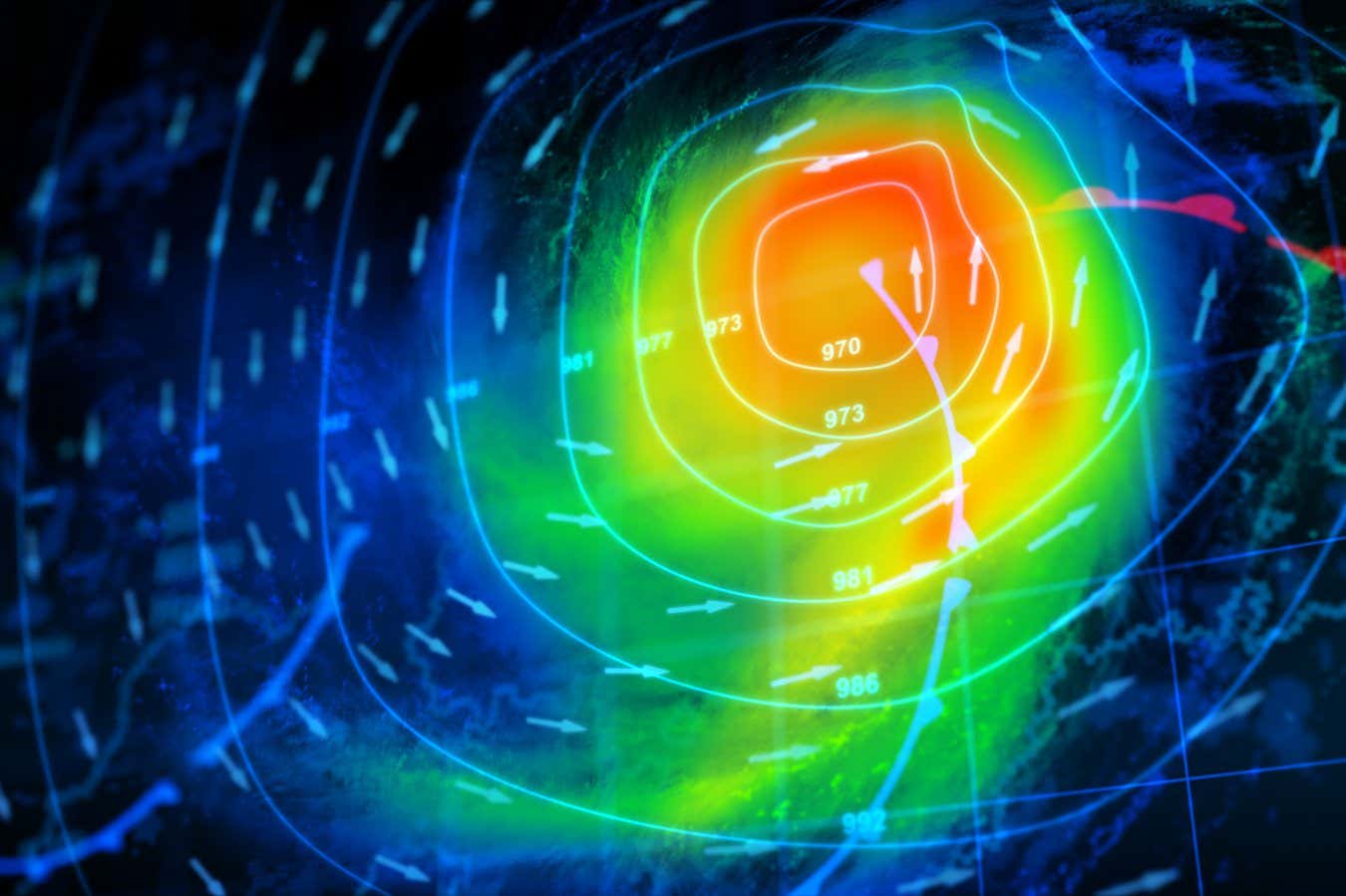
Advancements in Weather Forecasting: The Role of AI
Weather forecasting has always relied on complex physics-based models executed on powerful supercomputers. However, advancements in artificial intelligence (AI) are now promising to revolutionize this field. Google DeepMind has launched GenCast, its latest AI model aimed at enhancing the accuracy and speed of weather predictions.
Overview of GenCast
GenCast is part of DeepMind’s ongoing initiative to improve weather forecasting through AI technology. It was developed using four decades of historical weather data from the European Centre for Medium-Range Weather Forecasts (ECMWF). This data, stored in the ERA5 archive, encompassed measurements of temperature, wind speed, and pressure across various altitudes globally.
Training and Testing
- Training Data: The GenCast model utilized data up until 2018 for its training purposes, focusing on long-term climate patterns.
- Testing Period: Data from 2019 was employed to validate GenCast’s predictions against actual weather outcomes. The AI outperformed the traditional ECMWF’s ENS forecast 97.4% of the time, and even achieved a remarkable 99.8% accuracy when predicting forecasts beyond 36 hours.
DeepMind’s previous developments have also shown consistent success in weather forecasting, including an AI that surpassed traditional high-resolution 10-day forecasts by over 90% in accuracy.
Traditional vs. AI-Based Weather Forecasts
The existing methods for weather forecasting heavily depend on simulations that require immense computational resources. Forecasters typically run numerous simulations using slightly varied inputs—known as ensemble forecasting—to capture a range of potential weather outcomes.
Challenges with Traditional Methods
- High Computational Demand: These deterministic models consume significant energy and require highly sophisticated computing systems to function.
- Complexity: As predictive models increase in complexity and scale, so do the requirements for computing power and energy use.
The Benefits of AI in Weather Forecasting
AI models like GenCast indicate a shift towards a more efficient approach in weather forecasting. GenCast utilizes an ensemble of 50 potential scenarios, with each scenario processed in just 8 minutes using specialized Google Cloud TPU v5 chips.
Key Features
- Resolution: GenCast operates at a resolution of approximately 28 square kilometers, while the ECMWF’s updated ENS has improved to 9 square kilometers.
- Efficiency: By focusing on AI-based predictions, GenCast aims to create forecasts more rapidly and at a reduced computational cost compared to traditional methods.
Perspectives from Experts
Experts in the field highlight the potential and limitations of AI-driven weather forecasting.
- Ilan Price from DeepMind suggests that this method doesn’t necessarily require increasing data granularity or calculation intensity to obtain more accurate predictions.
- David Schultz from the University of Manchester emphasizes that AI models rely on extensive training data from traditional physics-based forecasts. He asserts that while AI tools like GenCast show promise, they cannot exist independently of previous efforts in numerical weather predictions.
Future Considerations
Experts like Sergey Frolov from NOAA stress that for AI models to progress, there must be improvements in training data quality. The current reliance on operational centers like ECMWF to provide high-fidelity data continues to be crucial for AI’s advancement in weather predictions.
Meanwhile, Kieran Hunt of the University of Reading acknowledges the combination of traditional forecasting and AI is bound to enhance the overall prediction quality. He believes using ensemble methods, as demonstrated during extreme weather events like the 40°C temperature observed in the UK in 2022, can improve accuracy; AI can help recognize forecasts that may seem outliers at first but align as the event draws nearer.
In summary, AI has the potential to significantly improve weather forecasting, offering faster and more cost-effective solutions. While these advancements promise remarkable benefits, the integration of AI-based and traditional methods appears essential for optimizing prediction accuracy.