DeepMind Attains Cutting-Edge Data-Efficient Reinforcement Learning with Enhanced Transformer World Models
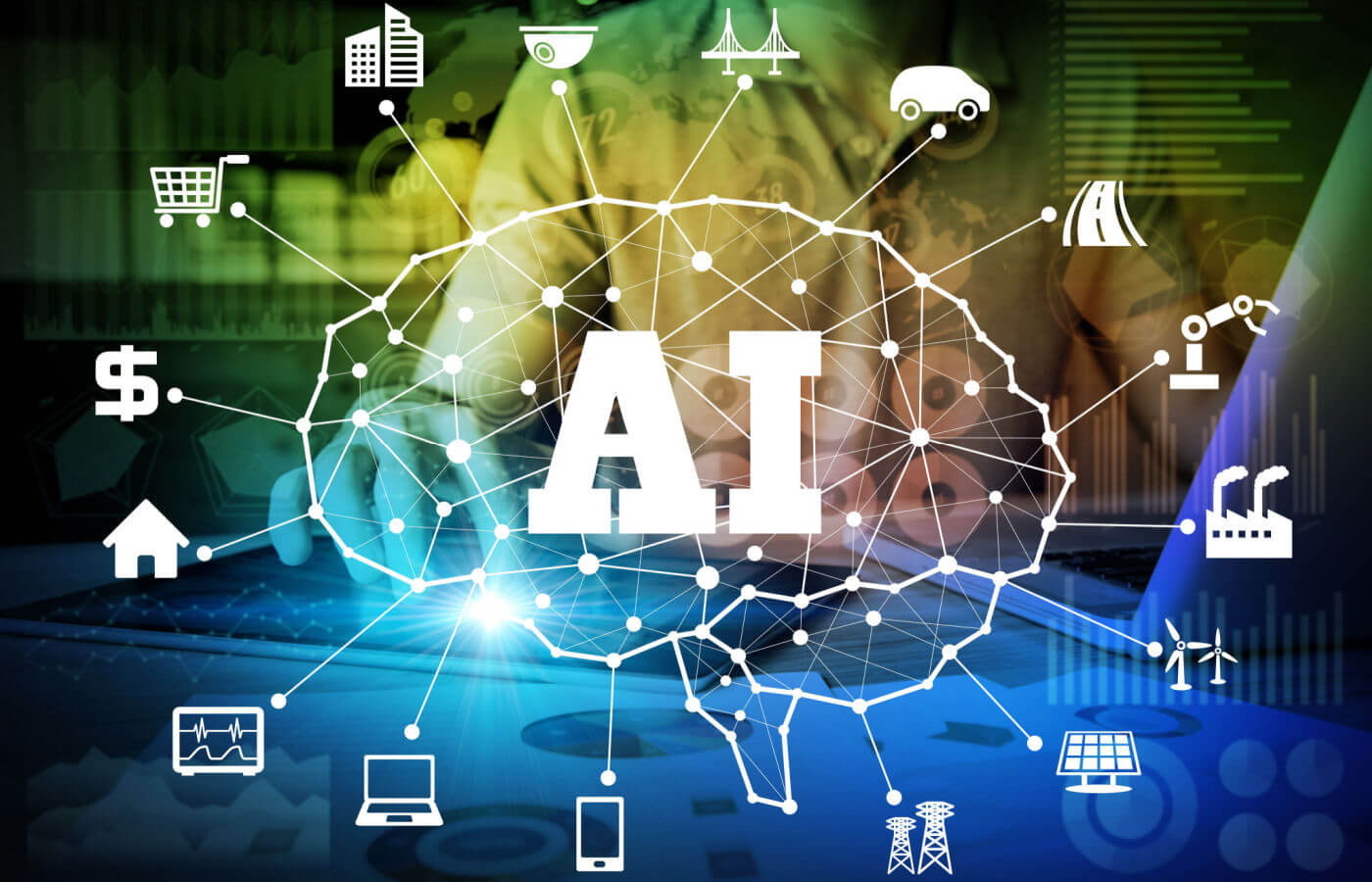
Google DeepMind’s Breakthrough in Reinforcement Learning
Google DeepMind has recently made significant strides in the field of reinforcement learning (RL), specifically focusing on data efficiency. This area of research is crucial as it aims to train AI agents to perform tasks using fewer data samples, thus making the learning process faster and more efficient.
What is Reinforcement Learning?
Understanding the Basics
Reinforcement learning is a type of machine learning where an agent learns to make decisions by interacting with an environment. It receives feedback through rewards or penalties based on its actions. Over time, the agent improves its strategy to maximize the cumulative reward it can achieve.
Importance of Data Efficiency
Traditionally, many reinforcement learning algorithms require large volumes of training data to perform well. This can be time-consuming and costly, especially when applied to real-world scenarios where data collection might be limited. Therefore, developing methods that require less data while still achieving high performance is a significant advancement.
Key Innovations by DeepMind
DeepMind’s research presents a new approach to RL that leverages improved transformer world models. Here are some core components of their innovation:
Improved Transformer Models
Enhanced Architectures: DeepMind’s work involves refining transformer models, which have been widely successful in natural language processing and are now being adapted for RL tasks.
- Incorporating Context: These improved models utilize contextual information from previous interactions, aiding the agent in making more informed decisions with limited data.
Efficiency in Learning
Fewer Samples Needed: With the enhanced transformer models, DeepMind demonstrates that the agent can learn effectively with significantly fewer samples compared to previous algorithms.
- Rapid Adaptability: The new system is designed to adapt quickly to changes in the environment, allowing for more flexible learning.
Applications of the Research
The advancements made by DeepMind could have far-reaching implications across various fields. Here are some potential applications:
Robotics
Robotic systems that require training in dynamic environments could benefit from this research. Robots could learn to interact with their surroundings efficiently, using less data to improve their performance.
Game AI
In the gaming industry, this technology can be used to develop more sophisticated AI opponents. These opponents would learn from fewer games played, allowing for quicker development cycles in game design.
Autonomous Systems
Autonomous vehicles and drones can also leverage this improved RL approach. By using less data to learn how to navigate complex environments, these systems can be deployed more effectively in real-world scenarios.
Challenges and Future Directions
While DeepMind’s advancements are promising, several challenges remain.
Generalization: Ensuring that AI agents can generalize their learning to new, unseen situations is a critical hurdle.
Real-time Iteration: The ability of the models to learn in real-time while engaged in tasks is still an area of ongoing research.
- Scalability: Making sure that these models can scale to larger environments and more complex tasks is essential for practical applications.
Conclusion
Google DeepMind’s latest work in data-efficient reinforcement learning is a significant evolution in AI technology. By reducing the data required for training, their methods promise to enhance the deployment of intelligent systems across various sectors. This pursuit for efficiency not only enables quicker learning but also makes advanced AI technologies more accessible for real-world applications.