DeepMind Introduces Inference Time Scaling for Diffusion Models
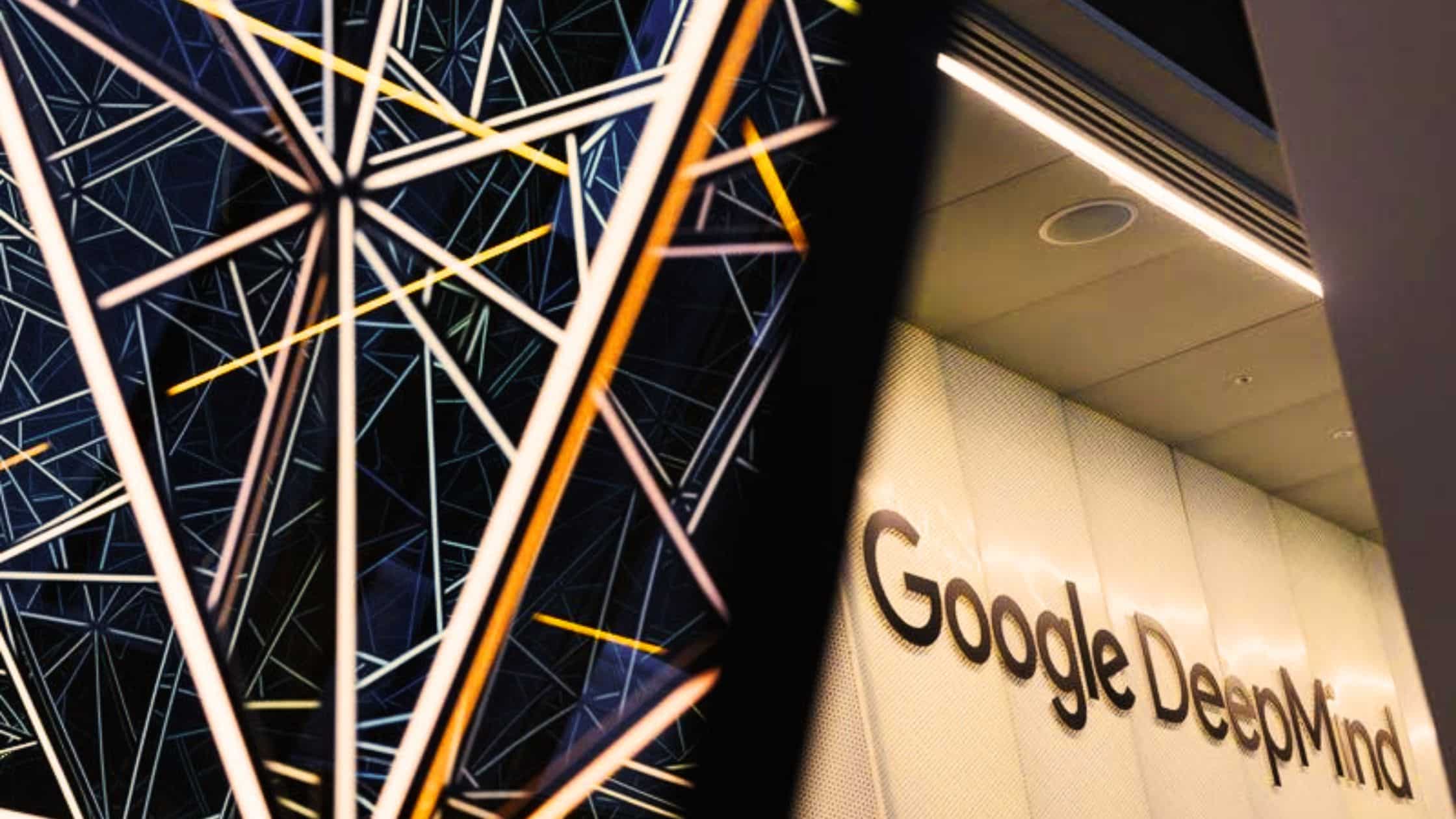
Understanding Inference-Time Scaling in Diffusion Models
Introduction to Google DeepMind’s Research
Google DeepMind, the artificial intelligence (AI) research division of Google, has joined forces with the Massachusetts Institute of Technology (MIT) and New York University (NYU) to explore a novel concept known as inference-time scaling for diffusion models. This collaborative study examines how providing additional computational resources during image generation can enhance the quality of outputs produced by these models.
What Are Diffusion Models?
Diffusion models are AI frameworks that generate images by starting from "pure noise" and progressively refining this noise through multiple denoising steps to produce clean outputs. The researchers in this study focused on how inference-time scaling—an approach that optimizes the use of computational resources—can improve image generation beyond merely increasing denoising steps.
Key Findings of the Research
The study, titled "Inference-Time Scaling for Diffusion Models Beyond Scaling Denoising Steps," highlighted several significant findings:
Improved Sample Quality: The researchers discovered that increasing the computing power during the inference stage led to notable improvements in the quality of the generated samples.
Search for Better Noise: One of the main contributors to the research, Nanye Ma, pointed out that better results can be achieved by enhancing the initial noise conditions. His comments on social media emphasized that investing resources in searching for more favorable noise options can significantly elevate the output quality.
Framework Components: The search framework employed in this study involves two main components: verifiers, which provide feedback on the generated output, and algorithms designed to locate better noise candidates.
- Model Performance Comparison: The research also compared the effectiveness of different inference-time search strategies across various models. Interestingly, smaller models equipped with effective search mechanisms outperformed larger models that lacked such methods. This suggests that the quality of samples can be enhanced without necessarily incurring high training costs by simply allocating more computational resources at inference time.
Inference Time Compute and Its Applications
Inference time compute is a concept that has gained traction, especially within the domain of large language models (LLMs). As evident from past research, such as OpenAI’s reasoning model, allocating additional computational power during inference often yields higher-quality and more contextually relevant responses. The authors of the paper indicated their intent to apply these successful techniques from LLMs to diffusion models.
Saining Xie, another author from the research team, expressed amazement at the scalability of diffusion models during the inference phase. He noted that while models may be trained under fixed computational constraints, they can be compensated during the testing stage to by increasing computational power by up to 1,000 times.
Implications for Future AI Developments
While the study primarily centers on image generation and evaluates models in the context of text-to-image benchmarks, it raises intriguing possibilities for future applications. If the techniques proven successful in this research can be leveraged for video generation, for instance, Google could gain a significant competitive edge over industry peers like OpenAI. For reference, Google’s Veo 2 model has already demonstrated superior performance in video generation compared to OpenAI’s Sora, both in quality and in adherence to prompts.
Final Thoughts
This innovative research from Google DeepMind, MIT, and NYU represents a meaningful advancement in the fields of image generation and AI technology. With the continuous evolution of diffusion models and inference-time scaling, the possibilities for creating high-quality AI-generated content are becoming increasingly promising. As the field progresses, the insights gained from such studies will undoubtedly pave the way for even more significant breakthroughs in AI capabilities.