DeepMind Introduces Watermarks for AI-Generated Text
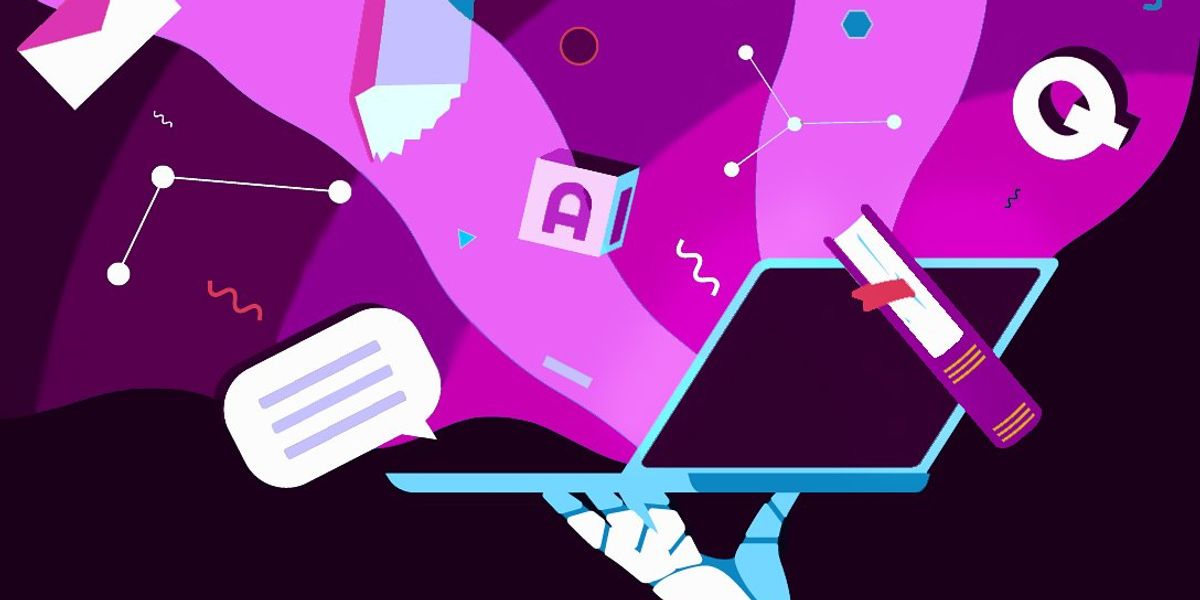
The Rise of Chatbots and AI-Generated Text
Introduction to AI Text Generation
The advent of chatbots has transformed the landscape of text generation. From news articles to academic papers and personal communications, AI-generated text has seeped into many aspects of our daily lives. This influx of automated content has prompted the growth of specialized industries aimed at differentiating between human-written and AI-generated text. On one hand, some firms offer tools designed to identify AI content, while others promote services claiming to make AI writing appear more human-like. However, both types of solutions often yield inconsistent results, especially as chatbots improve their capabilities.
The Challenge of Detecting AI-Generated Text
As AI technology advances, distinguishing between human and machine-generated text is becoming increasingly complicated. The rapid sophistication of chatbots makes it harder for individuals and organizations to determine the source of the content. In response to this challenge, researchers are exploring alternative approaches such as embedding watermarks or content credentials into the generated text. This would enable easier identification of whether a piece of text was produced by AI.
Google DeepMind’s Solution: SynthID-Text
Recent innovations by Google DeepMind offer a promising solution. Their research, published in Nature, introduces SynthID-Text, a new watermarking system designed specifically for text. According to Pushmeet Kohli, the vice president of research at Google DeepMind, this system does not compromise the quality or creativity of generated text. However, the technology is still in the early stages and has limitations.
Integration and Open Source
Google has already incorporated SynthID-Text into its Gemini chatbot platform. The company also made the tool available to developers and businesses, allowing them to assess whether their generated text is watermarked. Currently, only Google and selected developers have access to the detectors that can identify the watermarks. Kohli further notes that while SynthID is not a foolproof method for detecting AI-generated content, it represents a significant step toward developing more robust identification tools.
The Importance of Content Credentials
Content credentials have emerged as a critical topic in discussions about digital authenticity, particularly concerning images and videos. Initiatives like C2PA aim to establish encrypted metadata systems to discern between real and AI-generated media. However, applying these concepts to text presents unique challenges, as text can be easily altered to remove identifiable markings. SynthID-Text stands out as an initiative that has been extensively tested, with its performance evaluated against 20 million production prompts.
How SynthID-Text Works
SynthID-Text operates by subtly modifying the words generated by a chatbot without making the changes noticeable to human readers. This method involves introducing a statistical signature that can later be detected by SynthID’s detection system. The machine learning models (LLMs) driving chatbots typically generate text word by word. SynthID-Text influences this process by scoring potential next words, favoring those with higher scores, which later aids watermark detection.
Performance Evaluation
To ensure that SynthID-Text does not degrade the quality of chatbot responses, the researchers conducted extensive testing. They compared responses generated with and without the watermark using user feedback to gauge satisfaction levels. The results indicated that watermarked responses were just as well-received by users as the conventional responses, which is a positive sign for the future of AI-generated text.
Challenges in Implementation
Despite these advancements, the broader challenge of identifying AI-generated text remains. For effective detection, many AI companies would need to adopt watermarking technologies that are compatible with one another, allowing one detector to identify outputs from various LLMs. Additionally, open-source models present a hurdle, as they can be modified to remove watermarking features easily.
Bruce MacCormack from C2PA emphasizes the practical challenges involved in detecting watermarks, especially in real-world situations. It is essential to know which watermarking method cannot identify the text’s origins. While the journey ahead is lengthy and complex, this early-stage research indicates progress in establishing reliable tools for distinguishing AI-generated content.