DeepMind Researchers Introduce RT-Affordance: A Hierarchical Approach Utilizing Affordances as Intermediate Representations for Policy Development
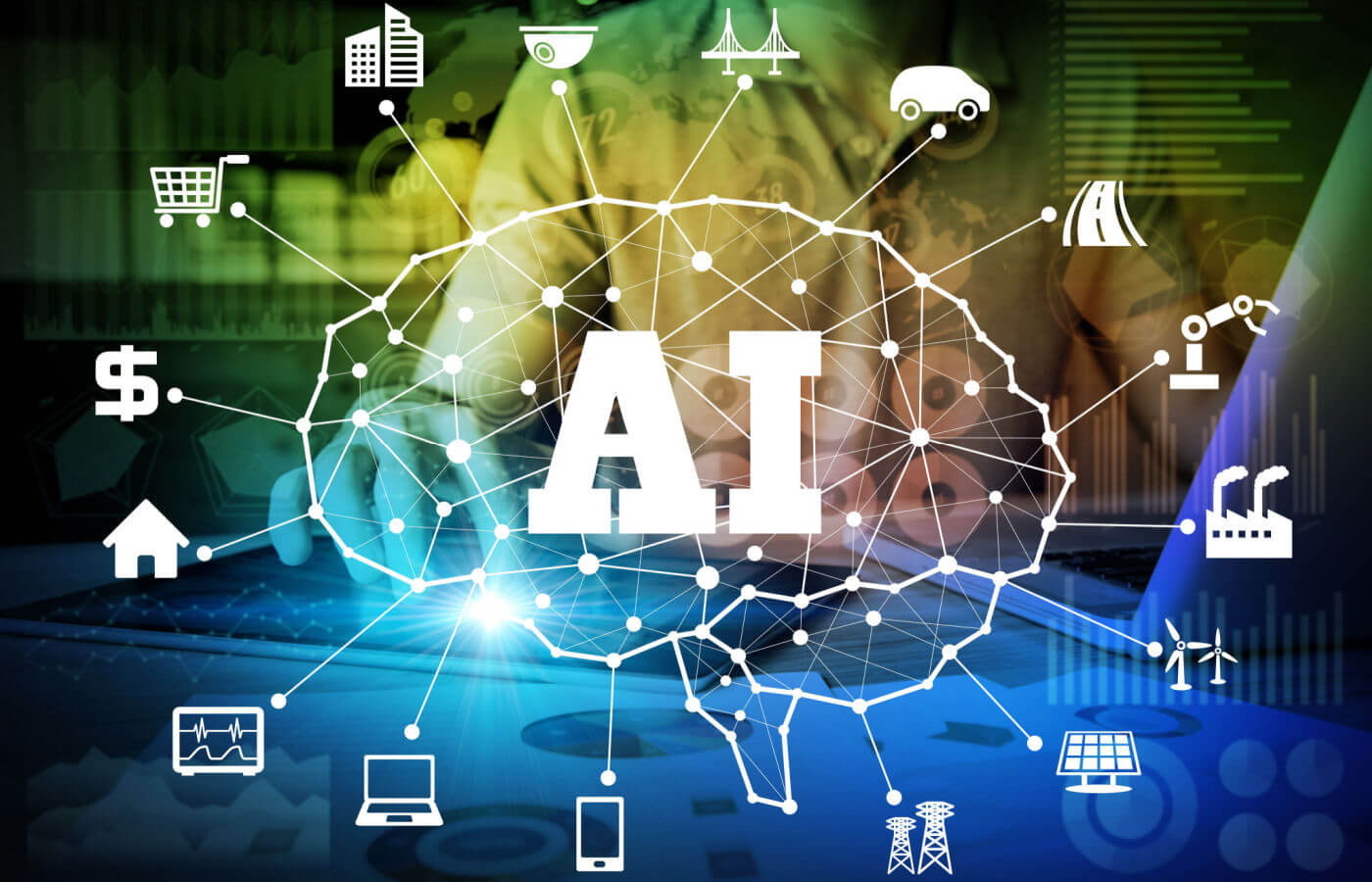
Understanding RT-Affordance: A New Hierarchical Approach
Introduction to Affordances in AI
In the field of artificial intelligence (AI), the concept of affordances plays a crucial role. Affordances are essentially the possibilities for action that an object provides to an agent. For instance, a chair affords sitting, while a door affords opening or closing. Understanding these affordances in a structured way can significantly enhance how AI systems interact with their environments.
The Hierarchical Method: RT-Affordance
Recently, researchers from Google DeepMind introduced a new technique called RT-Affordance. This method employs a hierarchical framework that utilizes affordances as a key element in shaping policies for decision-making in AI. By viewing actions through the lens of affordances, the RT-Affordance method provides a more nuanced understanding of how agents can interact with their surroundings.
Key Components of RT-Affordance
Hierarchical Structure: RT-Affordance operates on multiple levels, allowing for a deeper interpretation of actions. This structure enables AI systems to consider not just immediate actions but also the broader implications of those actions across different contexts.
- Affordances as Intermediate Representations: The method treats affordances as intermediaries in the decision-making process. This means that before an action is taken, the system identifies relevant affordances that guide the next steps, creating a more informed and adaptable interaction with the environment.
Benefits of RT-Affordance
Implementing RT-Affordance offers several advantages in various applications of AI:
Improved Accuracy: By leveraging affordances, AI systems can make better-informed decisions that reflect a more sophisticated understanding of their surroundings.
Enhanced Learning Efficiency: The hierarchical approach allows for more efficient learning. Rather than starting from scratch with every interaction, the system can build on previous experiences by recognizing patterns in affordances.
Adaptability: In dynamic environments, RT-Affordance provides AI systems the ability to adapt to new situations by allowing them to reevaluate actions based on the current affordances present in the environment.
- Complex Task Execution: The use of a hierarchical framework facilitates the execution of complex tasks, as systems can break down actions into manageable components based on affordances.
Practical Applications of RT-Affordance
The implications of RT-Affordance extend across various domains, including:
Robotics: Robots can benefit from a better understanding of how to interact with physical objects, allowing for more intuitive manipulation in tasks like assembly or navigation.
Autonomous Vehicles: In the context of self-driving cars, recognizing affordances can help these vehicles make safer and more effective driving decisions by better interpreting road signals and pedestrian behavior.
- Virtual Assistants: AI-driven virtual assistants can enhance their interaction quality by comprehensively understanding user commands through the lens of affordances, allowing for tailored responses that take into account the specific context.
Conclusion
RT-Affordance represents an exciting advancement in AI, bridging the gap between theoretical concepts and practical applications. By fostering a deeper understanding of actions through a hierarchical representation of affordances, this method brings forth a new paradigm in how intelligent systems perceive and engage with the world. As research continues to evolve, the potential impact of RT-Affordance on AI technology and its applications remains vast and profound.