DeepMind Unveils New AI Fact-Checking Benchmark Featuring Gemini as the Front Runner
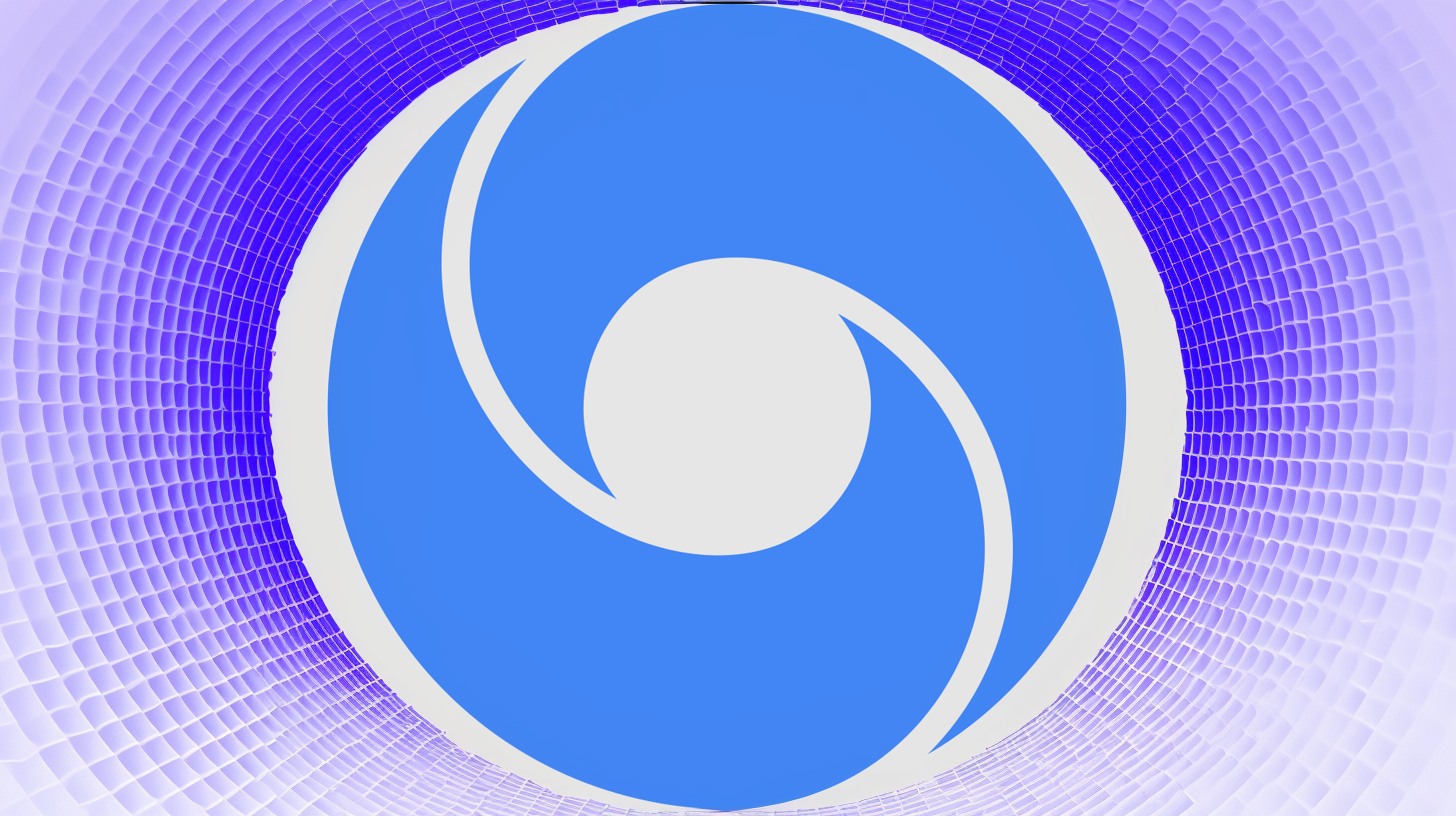
Understanding Google DeepMind’s FACTS Grounding Benchmark
Google DeepMind has recently launched a new benchmark called FACTS Grounding. This tool is designed to assess how well AI models can provide accurate answers based on documents. The benchmark uses a set of carefully chosen examples that challenge AI models to craft comprehensive responses using the information in the provided texts.
Key Features of FACTS Grounding
Overview of the Benchmark.
FACTS Grounding comprises 1,719 examples where AI models are required to generate in-depth answers from specific documents. A distinctive aspect of this benchmark is how the responses are evaluated. Three leading AI models—Gemini 1.5 Pro, GPT-4o, and Claude 3.5 Sonnet—perform the role of evaluators.
Evaluation Criteria.
These models assess the responses based on two primary standards:
- Relevance: Does the answer satisfactorily address the query?
- Factual Accuracy: Is the response correct and fully supported by the source document?
Types of Tasks
The documents used for testing cover various fields including finance, technology, retail, medicine, and law. They can be quite lengthy, consisting of up to 32,000 tokens, which is approximately 20,000 words. The types of tasks involved include:
- Summarizing documents.
- Answering specific questions based on the information.
- Rephrasing content to maintain the original meaning while using different wording.
These tasks are designed to require no creative input, specialized knowledge, or complex mathematical skills, making them accessible for evaluation.
Scoring System
To determine the final scores, the benchmark combines results from different scoring models for each given answer. The overall score for a task is an average derived from all the scoring model results across the various examples. Google DeepMind has set up a FACTS Leaderboard on Kaggle to showcase the performance results of different models.
Maintaining Integrity in Evaluation
To avoid any potential manipulation, Google DeepMind has divided the benchmark into two groups. The first group contains 860 public examples currently available, while the second group includes 859 examples that remain private. The ultimate score is calculated by incorporating results from both sets.
Challenges in Factual Accuracy
While large language models (LLMs) are revolutionizing how we access information, their accuracy still presents challenges. Complex queries can sometimes lead to “hallucinations,” where the AI generates incorrect information, which can erode trust in these systems. Google DeepMind recognizes these limitations, emphasizing that improving factual accuracy is a critical focus for their development.
Comparing with Other Benchmarks
FACTS Grounding takes a different approach compared to other benchmarks such as OpenAI’s SimpleQA. Unlike SimpleQA, which evaluates AI models using multiple knowledge questions from their training data, FACTS Grounding emphasizes how well models can interpret and utilize new information presented in documentary format.
Future Implications
Google DeepMind states that focusing on factuality and grounding is key to enhancing the effectiveness of LLMs and broader AI systems. It signals a significant step toward ensuring these technologies provide more reliable and trustworthy information to users.