DeepMind’s Latest AI Model Achieves Top Performance in Weather Forecasting
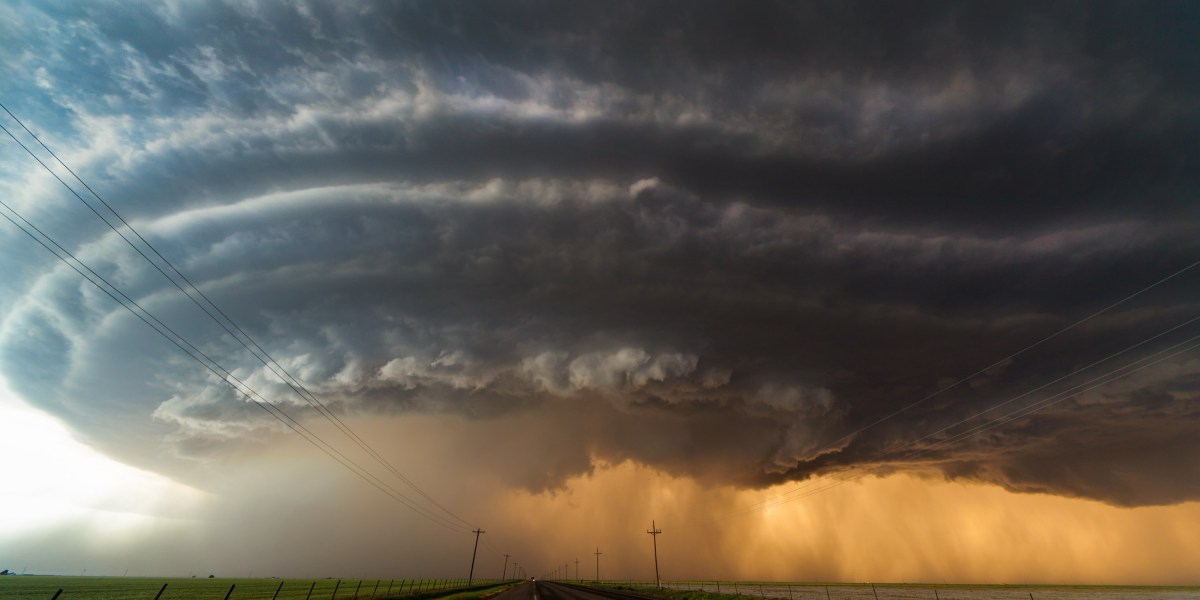
The Role of AI in Modern Weather Forecasting
Introduction to AI in Weather Prediction
Several major technology companies are now leveraging artificial intelligence (AI) to enhance weather forecasting. Notably, Google DeepMind is among the leaders in this field, but it’s not alone. Other companies such as Nvidia and Huawei are also making significant contributions.
Advancements in Weather Models
FourCastNet by Nvidia
In 2022, Nvidia introduced a model called FourCastNet. This AI system utilizes extensive data sets to make weather predictions, showcasing the potential for AI to revolutionize how we understand and forecast the weather.
Huawei’s Pangu-Weather Model
In 2023, Huawei unveiled its Pangu-Weather model, which has a long training history based on 39 years of weather data. This model generates deterministic forecasts, which provide a specific prediction rather than a range. For instance, it might predict tomorrow’s temperature to be exactly 30 °F or specify an expected rainfall of 0.7 inches.
Understanding GenCast
GenCast, another model, takes a different approach. It produces probabilistic forecasts, indicating the likelihood of various weather scenarios. For example, it could say there is a 40% chance that temperatures will drop to 30 °F or a 60% chance of receiving 0.7 inches of rainfall. This type of forecasting provides valuable insights, helping decision-makers understand potential weather events and plan accordingly.
The Role of Traditional Meteorology
Despite the advancements brought by AI models like GenCast and Pangu-Weather, conventional meteorology remains crucial. These AI models rely heavily on historical weather data, which makes them vulnerable to inaccuracies in long-term forecasting, especially in light of climate change and its unpredictable effects.
Data Sources and Modeling Limitations
Importance of ERA5 Dataset
GenCast uses a dataset known as ERA5, which encompasses hourly estimates of various atmospheric conditions dating back to 1940. According to Aaron Hill, an assistant professor at the School of Meteorology at the University of Oklahoma, ERA5 is rooted in a physics-based model that ensures reliability.
Challenges in Atmospheric Variables
Many atmospheric variables are not directly observable, which means meteorologists often depend on physical equations to make educated estimates. These estimates are then combined with available observational data and input into models like GenCast. Ilan Price, a researcher at DeepMind and one of GenCast’s developers, highlights that models trained on older data will likely underperform as conditions change. For instance, a model that was developed using data up to 2018 is expected to be less accurate in 2024 compared to a model trained with data available up to 2023.
Future Directions for AI in Weather Forecasting
DeepMind is currently investigating more practical applications of AI in weather forecasting. Future plans include directly testing predictions based solely on observational data such as wind and humidity readings. This approach could enhance the accuracy and reliability of forecasts even further.
Conclusion
The integration of AI into weather forecasting is reshaping how we predict climate-related events. With advancements from leading tech firms, the future of meteorology looks promising, combining traditional methods with innovative AI technologies. The adaptability and continued development of AI models may ultimately lead to more accurate and helpful weather predictions for various applications.