DeepMind’s Presentation at NeurIPS 2024
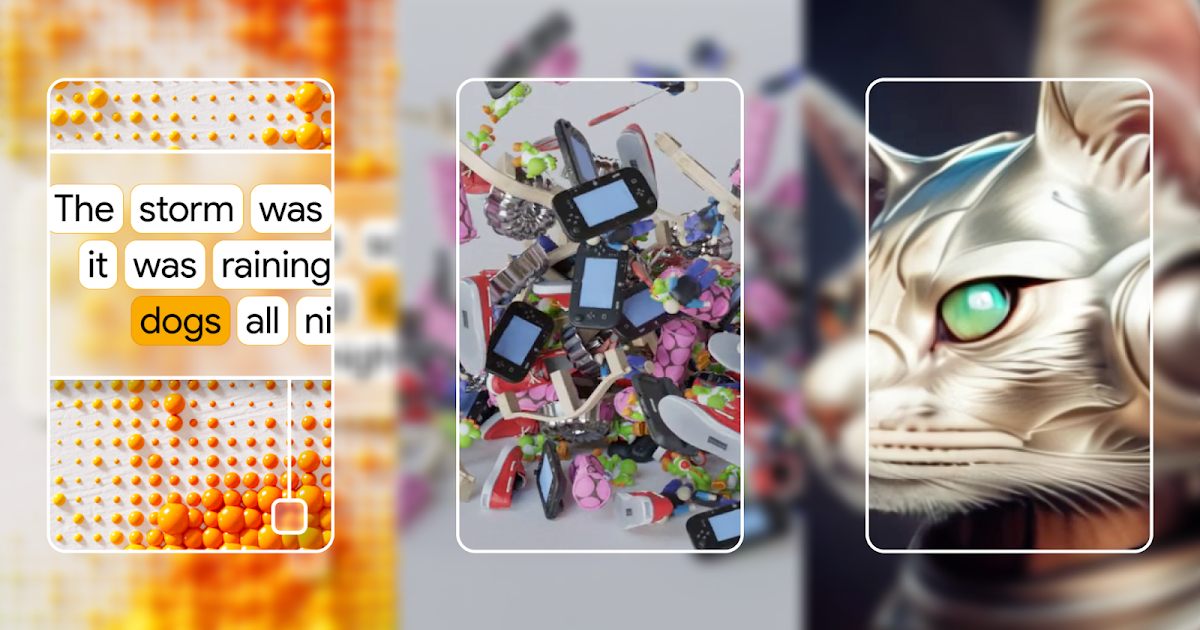
Advancements in AI: Exploring New Frontiers
As AI technology continues to develop rapidly, various conferences bring researchers together to share groundbreaking findings. The upcoming 38th Annual Conference on Neural Information Processing Systems (NeurIPS), happening from December 10 to 15 in Vancouver, is expected to showcase a wealth of new research. Among the most highly anticipated presentations are those from Google DeepMind, highlighting their significant contributions to the AI field.
Recognizing Influential Research
During the NeurIPS conference, two papers from Google DeepMind will receive Test of Time awards, acknowledging their lasting impact on AI research. Ilya Sutskever will discuss "Sequence to Sequence Learning with Neural Networks," co-authored with leading figures Oriol Vinyals and Quoc V. Le. Additionally, Ian Goodfellow and David Warde-Farley will present on Generative Adversarial Networks (GANs), which have transformed approaches to AI image generation.
Live Demonstrations
In addition to theoretical discussions, Google DeepMind will offer live demonstrations to illustrate the practical applications of their research. Attendees can expect showcases of Gemma Scope, an AI tool for music generation, and applications in weather forecasting, among others. These demonstrations serve to highlight how foundational research translates into real-world utility.
Advancing AI Agents
Building Adaptive AI
Large Language Model (LLM)-based AI agents are gaining traction in executing tasks through natural language commands. The effectiveness of these agents often hinges on their ability to interact with complex user interfaces. To enhance this capability, Google DeepMind introduces a dataset, known as AndroidControl, which contains over 15,000 human-collected demonstrations across more than 800 applications. This diverse dataset significantly boosts the training of AI agents, enabling them to perform a wider range of tasks more effectively.
Learning from Experience
For AI agents to operate efficiently in various scenarios, they must learn from their interactions. Google DeepMind proposes a method called in-context abstraction learning that allows AI to deduce key task patterns from less-than-perfect demonstrations and user feedback. This approach enhances an AI agent’s adaptability, allowing it to improve over time.
3D Scene Creation Innovations
Streamlining Content Creation
As the need for high-quality 3D content grows in industries such as gaming and visual effects, innovative tools are emerging to streamline the creation process. Google DeepMind presents CAT3D, a groundbreaking system capable of generating 3D content from various input types, including simple text prompts or single images. By utilizing a multi-view diffusion model, CAT3D creates additional consistent 2D images from multiple perspectives, which are then processed to yield realistic 3D models quickly and efficiently.
Efficient Scene Simulation
Simulating complex scenes with many objects can be computationally intensive. To address this challenge, a new technique called SDF-Sim has been developed. This method efficiently represents object shapes, speeding up collision detection and making large-scale scene simulations more manageable.
Enhancements in AI Learning Processes
Evolving LLM Training
The progress in LLM training practices aims to boost their efficiency and responsiveness. With the introduction of many-shot in-context learning (ICL), LLMs can learn from thousands of examples simultaneously. This promotes better performance in areas like math and translation, but it often relies heavily on high-quality, manually curated data. To tackle this issue, researchers are investigating means to reduce dependence on such data.
Introducing Time-Reversed Language Models
A novel approach called Time-Reversed Language Models (TRLM) has emerged, allowing LLMs to generate plausible queries based on traditional responses. This technique helps improve user instruction compliance and enhances accuracy in generating citations, as well as bolstering safety measures against inappropriate content.
Optimizing Training with JEST
To further refine the training process for large AI models, Google DeepMind has introduced the Joint Example Selection (JEST) algorithm. JEST selects the most crucial data points from larger batches, significantly reducing the necessary training rounds and computational demands, leading to improved efficiency.
Engaging the AI Community
Google DeepMind is proud to sponsor the NeurIPS conference, supporting initiatives such as Women in Machine Learning and LatinX in AI, among others. The conference serves as a vital gathering for professionals in AI, machine learning, and data science to connect and exchange knowledge. Attendees are encouraged to visit Google DeepMind’s booths to engage with the latest research through demos and workshops, enhancing collaboration within the global AI community.