DeepSeek Disrupts AI Field: The Next Breakthrough in AI May Rely on Enhanced Computational Power at Inference Instead of Increased Data
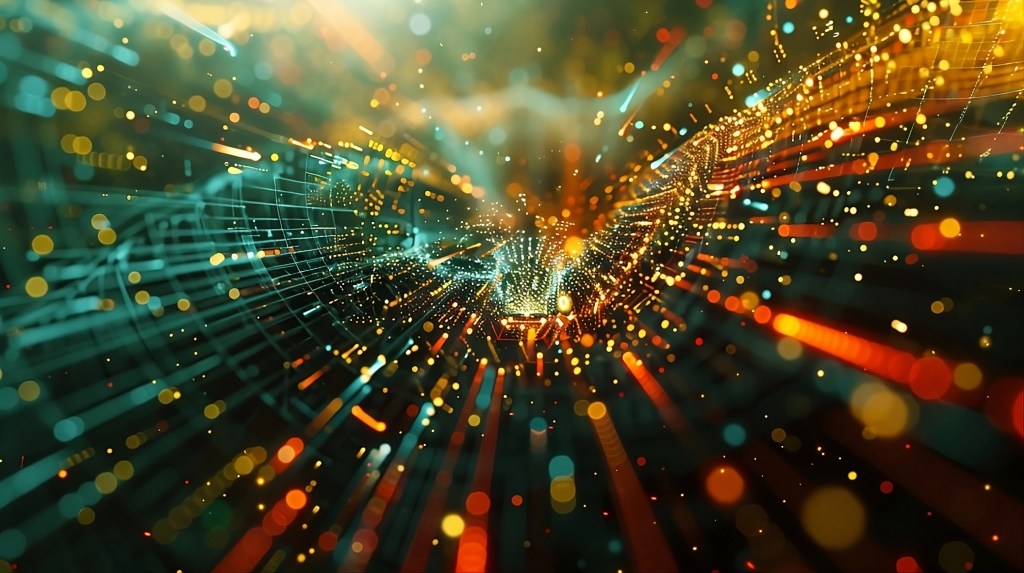
Recent Changes in the AI Landscape
The world of artificial intelligence (AI) is changing quickly. In early 2025, a breakthrough from DeepSeek, a Chinese AI lab, created a stir in the AI market. The introduction of their new model led to a 17% drop in Nvidia’s stock, along with declines in other tech shares related to AI data centers. This shift in the market is primarily due to DeepSeek’s ability to offer high-performance models at lower costs than U.S. competitors. This development has sparked discussions about what it means for AI data centers and the future of machine learning.
The Context of DeepSeek’s Disruption
One of the main factors driving the changes in the AI sector is the scarcity of available training data. Many leading AI laboratories have already utilized most public data from the internet for model training. This limitation is starting to slow down advancements in pre-training AI models. Consequently, companies are increasingly focusing on a new approach called "test-time compute" (TTC). This method allows models to engage in reasoning before providing responses, potentially improving overall performance. Experts believe that TTC could lead to advancements similar to those achieved through extensive pre-training.
Key Shifts in the AI Industry
The rise of new players like DeepSeek and the emphasis on TTC signify major changes in the competitive landscape of AI. Here’s a look at some important trends that are emerging:
1. Hardware Evolution
From Large Training Clusters to TTC Focus: As AI companies pivot towards TTC, there will likely be a shift in their hardware needs. Instead of just investing in massive GPU clusters aimed at training, companies will likely increase their spending on infrastructure suitable for inference. This change will also complicate capacity planning, as inference workloads can be more unpredictable than traditional training workloads.
- Increased Demand for Inference-Optimized Hardware: The emphasis on TTC may lead to a boost in demand for hardware designed specifically for low-latency inference, such as application-specific integrated circuits (ASICs). General-purpose GPUs, which are widely used for both training and inference, may take a backseat as specialized chip providers gain traction.
2. Cloud Platforms and Their Role
Quality of Service (QoS) as a Differentiator: As AI integration in businesses increases, challenges such as inconsistent response times and difficulties in handling concurrent requests may harm model adoption. Cloud platforms that can offer reliable service and address these issues will have a competitive edge.
- Increased Cloud Spending: Improved efficiency in AI training and inference could lead to a paradox where better performance drives higher consumption of cloud resources. Instead of reducing demand for AI hardware, efficient models could encourage more developers to adopt sophisticated reasoning models.
3. Foundation Model Providers
- Impact on Proprietary Models: The entrance of cost-efficient companies like DeepSeek challenges the traditional positioning of established AI labs. As new models emerge, proprietary training techniques may lose their effectiveness, fostering a new wave of innovation in existing labs.
4. Enterprise AI Adoption
Security and Privacy Concerns: Given DeepSeek’s roots in China, concerns about security and privacy make many companies hesitant to adopt its products. Many organizations in Western markets are reportedly blocking DeepSeek applications due to these worries.
- Focus on Specialized Applications: Businesses are increasingly turning to customized AI applications that serve specific needs. Techniques like retrieval-augmented generation and domain-specific optimizations are being utilized to adapt generalized models to specialized tasks. As improvements in TTC reduce the urgency for vast amounts of pre-training data, new opportunities for specialized application developers are likely to emerge.
The Future of AI in Business
As the emphasis on TTC grows, companies focused on enhancing inference capabilities will be crucial in optimizing AI performance. This shift may confirm that advancements in AI need not solely rely on vast data but can also stem from improving existing frameworks. Furthermore, established players may be encouraged to adopt newer methods to keep pace with these developments.
Overall, the rise of DeepSeek and similar models suggests a dynamic future for AI, where innovation may come from less traditional sources, fundamentally altering business practices and strategies across the board.