DeepSeek Introduces DeepSeek-Prover-V2: Enhancing Neural Theorem Proving with Recursive Proof Search and an Innovative Benchmark
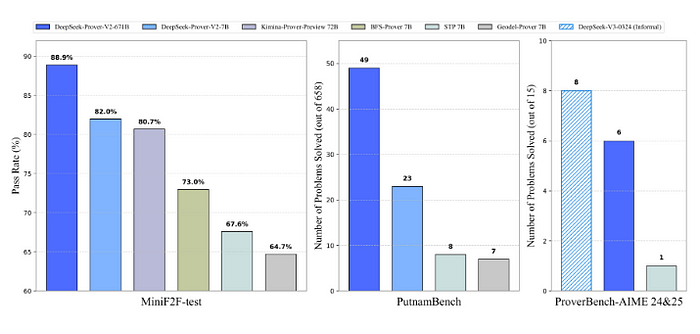
Introduction to DeepSeek-Prover-V2
DeepSeek AI recently unveiled DeepSeek-Prover-V2, an innovative open-source large language model tailored specifically for formal theorem proving within the Lean 4 framework. This advanced version stands on the shoulders of earlier iterations, introducing a unique recursive theorem-proving pipeline that harnesses the robustness of the DeepSeek-V3 model to generate its own high-quality initialization data. Notably, the new model exhibits state-of-the-art performance in neural theorem proving tasks and is accompanied by ProverBench, a fresh benchmark designed to assess mathematical reasoning capabilities.
Key Features of DeepSeek-Prover-V2
Cold-Start Training Procedure
One of the key advancements in DeepSeek-Prover-V2 is its distinct cold-start training process. This mechanism kicks off by using the highly capable DeepSeek-V3 model to break down challenging mathematical theorems into simpler, more manageable parts. Concurrently, it formalizes these higher-level proof steps in Lean 4, establishing a structured framework of sub-problems.
Efficient Proof Search
To effectively navigate the complex proof search for each subgoal, researchers utilized a smaller 7-billion-parameter model. After successfully proving each decomposed step of a complicated theorem, the model constructs a complete, step-by-step formal proof, coupled with its corresponding chain-of-thought reasoning from DeepSeek-V3. This methodology enables the model to learn from a synthesized dataset that combines informal mathematical reasoning with formal proofs, creating a potent foundation for later reinforcement learning stages.
Model Training and Performance
Building on the synthetic cold-start data, the DeepSeek team gathered a selection of challenging problems which the smaller 7B prover model could not fully solve. These problems had their subgoals individually addressed, allowing the team to assemble a complete proof for the original problem. This formal proof is paired with DeepSeek-V3’s reasoning outline, creating a holistic training example that interweaves informal reasoning with formalized arguments.
Fine-Tuning and Reinforcement Learning
The prover model is initially fine-tuned on the synthetic dataset and is further refined through a reinforcement learning phase. During this phase, it receives binary feedback—correct or incorrect—as a reward signal. This iterative process sharpens the model’s skill in connecting informal mathematical insights with the meticulous construction of formal proofs.
Achievements and Benchmarks
DeepSeek-Prover-V2–671B is the model featuring an astounding 671 billion parameters. Its performance metrics are laudable, achieving an impressive 88.9% pass rate on the MiniF2F test and successfully solving 49 out of 658 problems from PutnamBench. The proofs generated for the miniF2F dataset are publicly accessible, allowing researchers and users to review and analyze them.
Introduction of ProverBench
Alongside the new model, DeepSeek AI has rolled out ProverBench, a benchmarking dataset that consists of 325 problems. This benchmark serves to provide a thorough evaluation of mathematical reasoning skills at various difficulty levels.
Diversity in Problem Types
ProverBench incorporates 15 problems from recent American Invitational Mathematics Examination (AIME) contests (AIME 24 and 25), presenting authentic high-school-level challenges. The remaining 310 problems are crafted from curated textbook examples and educational tutorials, ensuring a diverse range of formalized mathematical issues across different domains.
Model Variations and Accessibility
DeepSeek AI is offering DeepSeek-Prover-V2 in two sizes to accommodate varying computational needs: a smaller 7B parameter version and a larger 671B parameter model. The larger DeepSeek-Prover-V2–671B leverages the strong foundation of DeepSeek-V3-Base, while the smaller model, DeepSeek-Prover-V2–7B, draws from DeepSeek-Prover-V1.5-Base, extending its context capability to 32,000 tokens. This enhancement allows it to manage longer and more intricate reasoning sequences effectively.
The rollout of DeepSeek-Prover-V2, paired with the ProverBench benchmark, signifies an important advancement in neural theorem proving, fostering the development and assessment of more sophisticated AI systems designed for formal mathematical tasks.