Exploring Researchers’ Strategies to Enhance the Efficiency of AI-Powered Systems
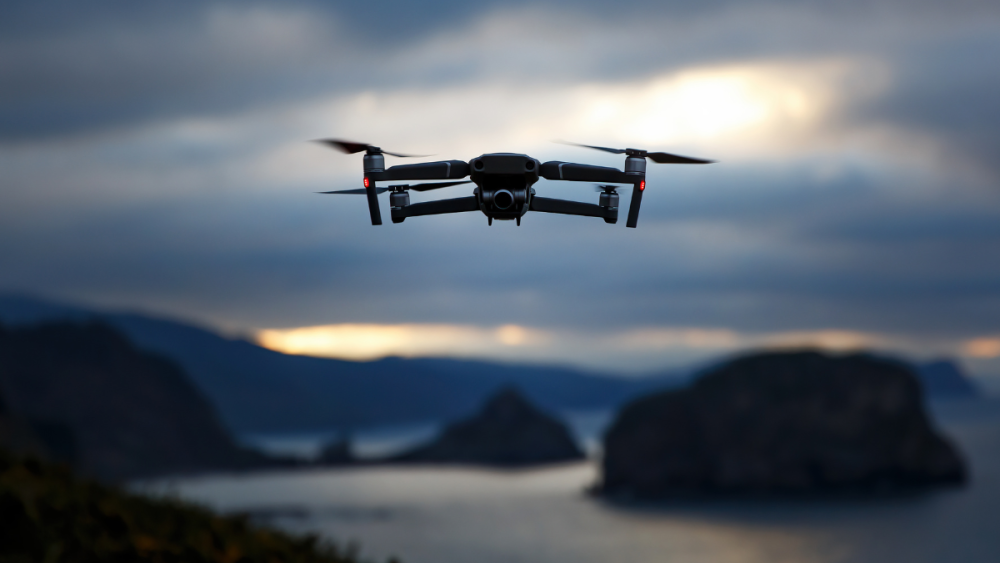
Enhancing AI Efficiency at Penn State
Introduction to Selective Learning
At Penn State University, researchers are innovating in the field of artificial intelligence (AI) to improve the efficiency of unmanned autonomous vehicles (UAVs). They are using a method known as "selective learning," which focuses on collecting only the essential data required to solve a problem. This strategy is different from traditional approaches, which often gather extensive data sets and sort through them later to find relevant information.
Insights from Human Biology
Soundar Kumara, a professor of Industrial Engineering at Penn State and the leader of this research, likens their approach to the “selective attention” feature of the human brain. Like our brains, which prioritize critical information to save energy and resources, Kumara’s team designed algorithms that minimize the data requirement for AI systems, making them more efficient. This research is crucial because many AI applications, including UAVs, have limitations regarding computing power, energy consumption, storage space, and data transmission.
Efficiency in AI-Powered Systems
The team’s novel methodology can lead to substantial reductions in the resources needed for AI operations. In their findings, they reported that using this selective learning technique could decrease the computing resources needed by UAVs by as much as 435 times. This efficiency is vital for a wide range of applications such as wearable electronics and various industrial equipment.
What Are AI-Powered Vehicles?
AI-powered vehicles, particularly UAVs, are designed to navigate autonomously by using data collected from onboard sensors. These vehicles can be used in various scenarios, from search and rescue missions to infrastructure inspections and even extraterrestrial exploration. For example, UAVs can operate in environments unsafe for humans, allowing them to gather data and complete tasks that would otherwise be challenging.
Sensor Technology
UAVs utilize diverse sensors, including standard cameras, multi-spectral cameras, gyroscopes, and accelerometers. These sensors generate vast amounts of data. The selective learning approach developed at Penn State has been tested with data gathered from various mechanical components, such as motors and turbines, showcasing its versatility.
Data and Energy Consumption in AI Systems
Data usage in UAVs varies significantly based on the specific vehicle and application. As established by the Shannon-Nyquist sampling theorem, the sampling rate must be set at least twice that of the highest frequency present to ensure no information loss. UAVs typically require high sampling frequencies, which can generate thousands to millions of data points every second.
For instance, an aircraft can have around 300 different sensors, resulting in several gigabytes of data generated per flight. The research indicates that using the team’s approach can lead to a considerable reduction of energy needed—up to 435 times less—resulting in longer battery life and lighter vehicular designs.
Making UAVs More Efficient
To enhance energy and data efficiency of UAVs, a Penn State collective developed advanced neural network architectures. Ankur Verma, a doctoral graduate, was pivotal in combining data collection and model training processes. This integration results in a 10-fold reduction in data requirements and a staggering 435-fold cut in necessary computational power.
As a result, these models are lighter and quicker while consuming less energy, crucial for applications ranging from satellites to drones. The team’s approach allows for AI advancement in resource-constrained environments.
Future Directions
The research team at Penn State has successfully applied their findings in various sectors, including industrial equipment monitoring and activity recognition in wearable devices. They aim to commercialize their technology through a company named Lightscline, co-founded by team members, to bring this innovative approach to industries such as space and defense.
The initial exploration of this strategy is supported by funding from the U.S. National Science Foundation’s I-Corps program, aiming to assess its market viability.
By leveraging selective learning, Penn State researchers are paving the way for more effective and efficient AI systems, transforming the capabilities of UAVs and potentially other autonomous technologies in the future.