Exploring Socratic Learning through Language Games: A Journey Towards Self-Improving Superintelligence
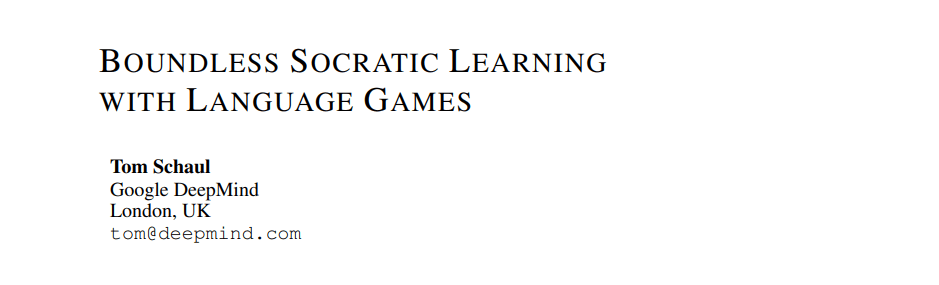
Understanding Recursive Self-Improvement in AI
The Quest for Artificial Superhuman Intelligence
As we strive for artificial superhuman intelligence (ASI), a pivotal milestone is a system’s ability to improve itself without relying on human input. This self-improvement, known as recursive self-improvement, is still largely absent in existing AI systems. Now is an excellent opportunity to explore and define this concept and its implications.
Introducing Socratic Learning
A recent research paper titled Boundless Socratic Learning with Language Games from Google DeepMind introduces the idea of "Socratic learning." This innovative approach represents a form of recursive self-improvement where AI systems can significantly enhance their performance beyond their initial knowledge. The only limits are time and the risk of gradual misalignment from objectives. The researchers outline a practical framework for Socratic learning, which relies on the concept of “language games.”
Key Aspects of Socratic Learning
Closed Self-Contained Environments
The study places a strong emphasis on closed environments for Socratic learning, where AI systems operate independently without tapping into external data. The authors suggest that an agent can master any desired skill if it meets three essential criteria:
- Informative Feedback: The feedback provided must be insightful and aligned with the desired goals.
- Broad Experience Coverage: The AI’s learning and data should cover a wide range of experiences.
- Adequate Resources: Sufficient computational power and resources must be available.
By fulfilling these conditions, researchers are optimistic about exploring the potential of recursive self-improvement toward artificial general intelligence (AGI).
The Importance of Language Games
In the context of Socratic learning, language games play a crucial role. These games are structured protocols where AI agents interact using language-based inputs and outputs. Each interaction results in a score that reflects the performance of the agents. This game setup directly addresses two vital needs of Socratic learning:
- Interactive Data Generation: The structure enables agents to generate data through self-play, allowing for scalable learning opportunities.
- Intrinsic Feedback Mechanism: The scoring system provides ongoing feedback that helps refine the agents’ actions.
Levels of Recursion in Learning
The researchers propose two levels of recursion within their framework:
- Hierarchical Reinforcement Learning: This level offers agents more autonomy and a broader range of abstract actions.
- Protocol Interaction: Games can be treated as fully encoded interaction protocols within the agent’s language. This capability enables the agent to autonomously create and engage in new games, thereby enhancing its learning experience.
Self-Modification for Higher Performance
The final step in this recursive learning process is self-modification. Here, agents can change their input streams and internal structures. Such flexibility allows them to surpass the limitations of their initial designs, unlocking greater potential for improvement and capability expansion.
The Potential of Socratic Learning
The authors of the study envision Socratic learning as a transformative pathway toward achieving AGI. With the foundation provided by language games, this innovative approach shows promise in overcoming current constraints and unlocking new levels of AI performance. The framework helps address crucial challenges, guiding future research endeavors in the field.
For more detailed insights, the paper Boundless Socratic Learning with Language Games can be accessed on arXiv.