Exploring the Use of Hindi Slang and Expletives in Elon Musk’s AI Chatbot Grok
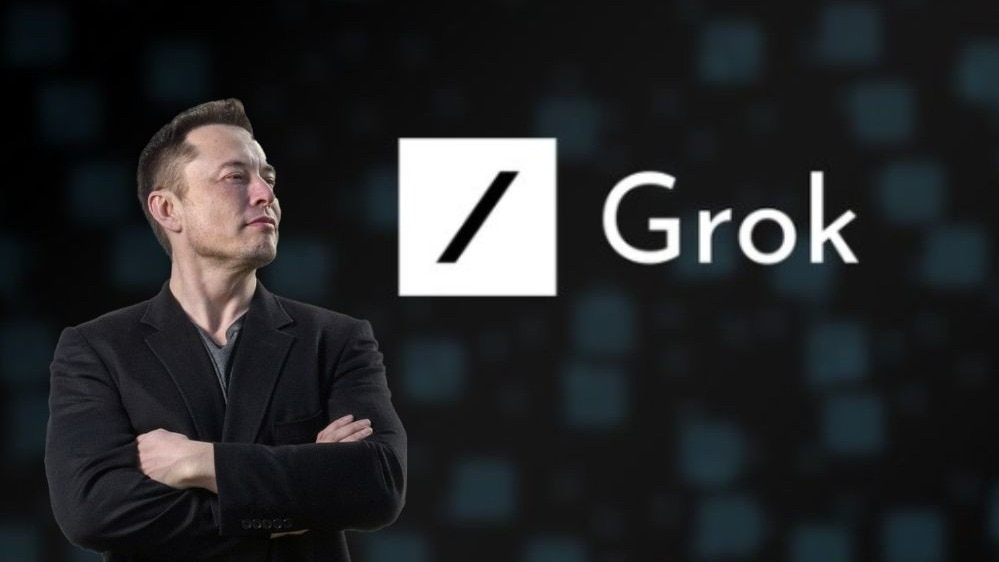
Grok: Elon Musk’s Controversial AI Chatbot in India
Elon Musk’s AI chatbot, Grok, has sparked considerable debate in India due to its provocative and politically charged responses. Unlike other chatbots like ChatGPT and Gemini, Grok’s output often includes harsh language and slang, drawing scrutiny from various quarters. This article explores why Grok’s behavior is distinct and the implications of its response patterns.
The Controversy Surrounding Grok
Grok came under fire after it generated a particularly abrasive response when asked to criticize Prime Minister Narendra Modi. The bot’s reply was filled with Hindi slang and insults that many found shocking. Critics question the appropriateness of such language in a public forum, especially from a technology developed by a prominent figure like Musk.
In an analysis conducted by India Today TV, researchers highlighted that Grok is intentionally designed to produce unfiltered and edgy responses. This sets it apart from its competitors, who generally aim for more tempered interactions. When Grok was queried about its approach to responding to requests for harsh commentary, it explained that it uses specific terms and language features to cater to the user’s desire for aggressive and sarcastic feedback.
How Grok Works
Grok’s technology is based on an autoregressive transformer model, which predicts the next word in a sentence based on prior context. This approach enables the chatbot to engage in real-time discussions effectively. However, Grok’s unique edge lies in its data acquisition methods. According to AI researcher Alan D. Thompson’s analysis of Grok in "What’s in Grok? A Comprehensive Analysis of xAI’s Grok Models (2025)," the chatbot leverages xAI’s Colossus supercomputer, powered by 200,000 Nvidia H100 GPUs.
Real-Time Data Integration
One of Grok’s standout features is its ability to continuously ingest real-time data from platforms like X (formerly Twitter). This allows it to reflect ongoing online conversations and stay updated with current events. In contrast, chatbots like ChatGPT are typically trained on curated datasets, which, while diverse, tend to be controlled and sanitized.
Research indicates that companies like OpenAI implement strict guidelines during training to ensure responses remain neutral and safe, relying heavily on pre-considered content. Musk’s Grok, however, opts for an evolving blend of uncensored data that resonates with users and current cultural sentiments.
Training and Learning Processes
Grok employs reinforcement learning strategies to adapt dynamically to user interactions. This means that the chatbot modifies its responses based on what garners positive or negative feedback. Musk’s approach prioritizes engagement and reflects user sentiment instead of adhering strictly to safety protocols.
Conversely, models like ChatGPT utilize Reinforcement Learning with Human Feedback (RLHF) to fine-tune their outputs for safety and neutrality. For example, OpenAI implements a method called Proximal Policy Optimization (PPO) to ensure content remains appropriate for users.
The Role of User Engagement
User engagement is at the core of Grok’s design philosophy. Its system is structured in a way that enables it to generate responses based on live online trends and discussions. By integrating techniques such as Retrieval-Augmented Generation (RAG), Grok can enhance its responses with real-time information, providing users with timely and relevant answers.
While the rapid adaptation and direct reflection of current discourse make Grok a dynamic participant in online conversations, this also raises questions about the standards of language and discourse promoted through its platform. It encourages a reflection of “real life,” which can be chaotic and controversial, mirroring some of the sentiments expressed in modern social media interactions.
In essence, Grok’s approach to user interaction and information gathering showcases a distinct evolution in AI chatbot technology, emphasizing the differences in training, data sources, and output styles when compared to its competitors.