Gemini 2.0 Enhances Robotics with Multimodal Flexibility
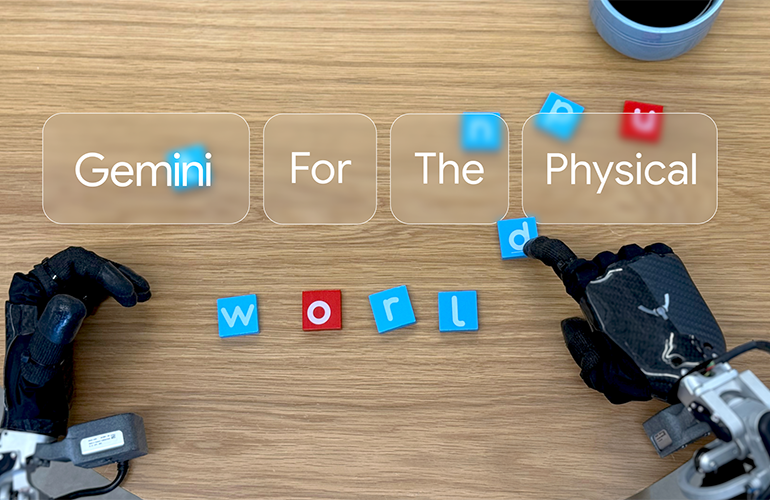
Google’s Advances in Robotics with Gemini 2.0
Earlier this year, NVIDIA introduced Cosmos, a new framework that aims to connect artificial intelligence (AI) with the physical world. The CEO of NVIDIA, Jensen Huang, describes it as the next level of AI focusing on physical applications. Following this, Google has unveiled its own initiative called Gemini 2.0, which adds a new dimension to AI and robotics.
What is Gemini Robotics?
Gemini Robotics represents Google’s latest advancement in large multimodal foundation models. Originally designed to work with text and images, this new model incorporates physical actions as an additional output. The core functionality of Gemini Robotics is to allow robots to see and interpret their surroundings using cameras and sensors. It can understand verbal instructions in a natural language format, enabling it to execute commands through low-level control of robotic systems. This capability makes it a "vision-language-action" (VLA) model.
Training and Capabilities
Google has developed Gemini by merging extensive web-scale data with insights from its in-house robotics systems, such as the bi-arm apparatus known as "ALOHA 2." This dual-layered training allows Gemini to manage highly dynamic tasks, including those not covered during initial training, by leveraging a vast underlying knowledge base. In addition to the primary model, Google introduced Gemini Robotics-ER, which possesses advanced spatial understanding features. This model assists robotics engineers in executing specific programs that benefit from Gemini’s reasoning capabilities.
Supporting Complex Tasks and Dexterous Movements
Gemini Robotics is targeted at general-purpose robots designed to perform various tasks that require human-like decision-making skills and dexterity. It can undertake intricate, multi-step operations, such as folding origami or selectively packing snacks into bags. These activities require not only precise control of fingers and hands but also a contextual understanding of different materials and motion dynamics.
Key Features of Gemini Robotics
Gemini Robotics is built on three essential qualities:
Generality: The ability to handle a diverse range of instructions and objects, even ones not previously encountered, by drawing from its extensive knowledge base.
Interactivity: The capability to engage with users and adapt promptly to changing commands or environmental factors.
- Dexterity: The skill to manipulate objects with the same finesse as humans.
The Gemini-ER model acts as a high-level intellectual processor but does not manage low-level controls directly. Instead, it can integrate with existing robotic controllers to enhance their performance. For instance, it can take 3D sensor data to enhance perception and planning, directing the actions of various robotic systems—be it industrial arms or humanoid robots. This flexible setup allows many types of robots to utilize spatial intelligence effectively.
Ensuring Safety in Robotics
As robots gain more autonomy, safety becomes an increasing concern. Google DeepMind has adopted a layered and holistic approach to safety within its designs. This includes:
- Implementing traditional hardware and software measures to prevent collisions and control force.
- Introducing contextual safety reasoning so that Gemini can identify unsafe instructions and either reject them or suggest safer alternatives.
- Utilizing a data-driven robot constitution inspired by Asimov’s “Three Laws” to guide robotic actions.
- Creating the ASIMOV safety benchmark for evaluating how often robots select safe versus unsafe actions in various scenarios.
The Competitive Landscape
Beyond just Google and NVIDIA, other companies are also advancing in AI and robotics. OpenAI has collaborated with humanoid robot startups to integrate GPT-based systems that can grasp human language and respond physically. Microsoft is experimenting with automating control code generation for drones and robotic arms using ChatGPT. Meanwhile, Tesla’s work on its Optimus humanoid robot benefits from real-world data collected from its self-driving technology. Other notable efforts include Meta’s commitment to developing simulation platforms for embodied AI and Boston Dynamics’ push for higher autonomy in its dexterous robots.
A Legacy of Innovations at Google DeepMind
Google DeepMind has been a leader in the field of reinforcement learning, a technique critical for robotics. For example, it developed the Deep Q-Network (DQN) around 2013, which learned to play video games at a level comparable to humans. The AlphaGo system later combined reinforcement learning with other methods to conquer professional Go players. With ongoing projects like AlphaFold, the company continues to make significant strides in both AI and robotics.