Gemini Hackers Enhance Attack Potential with Internal Assistance
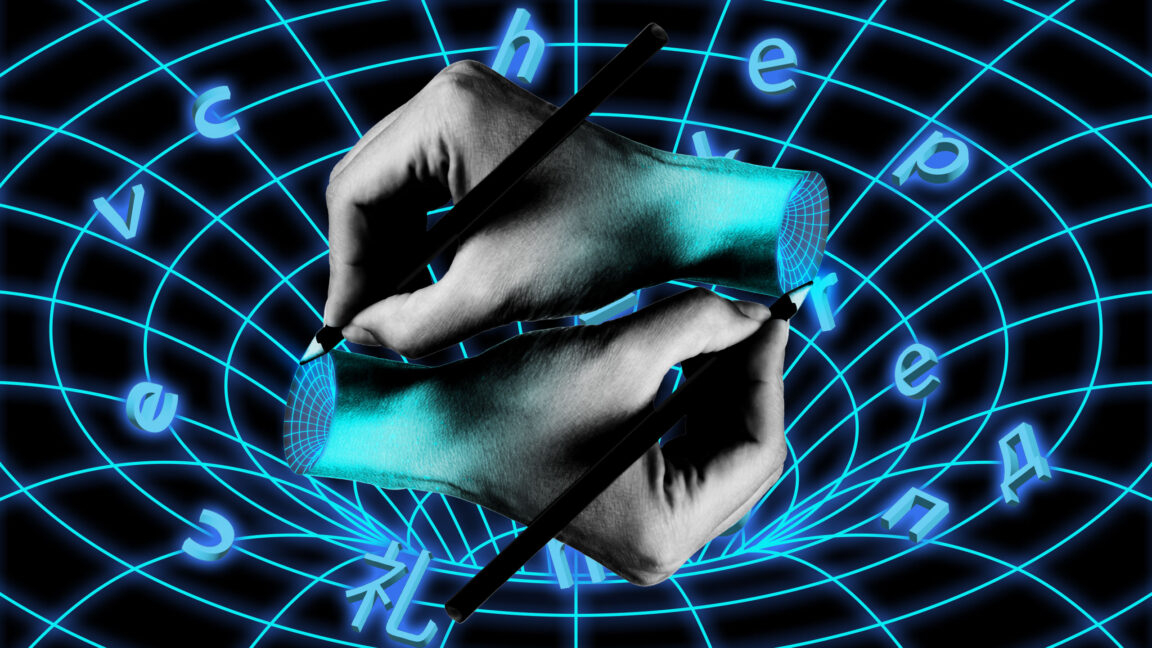
Attack Success Rates in Gemini Models
The research focused on analyzing the performance of various models within the Gemini framework, specifically Gemini 1.5 Flash and Gemini 1.0 Pro. The resulting dataset revealed significant insights into attack success rates, comparing them with baseline rates and evaluating the effects of different tuning methods.
Attack Success Rates
The study found that the success rates for attacks against the Gemini models were notably high. The breakdown of the success rates is as follows:
- Gemini 1.5 Flash: 65%
- Gemini 1.0 Pro: 82%
In contrast, the baseline success rates for attacks were significantly lower:
- Baseline for Gemini 1.5 Flash: 28%
- Baseline for Gemini 1.0 Pro: 43%
Furthermore, when investigating the effects of fine-tuning, the ablation tests showed success rates of 44% for Gemini 1.5 Flash and 61% for Gemini 1.0 Pro. These results indicate that fine-tuning methods, referred to as "Fun-Tuning," yield higher success rates compared to traditional methods.
Transferability of Attacks
Another crucial finding from the research is the transferability of attack strategies between different versions of the Gemini models. When an attack method is developed for one model, it shows a high likelihood of being effective on another model within the Gemini series. Daniel Fernandes, one of the researchers, highlighted this feature, stating, “If you compute the attack for one Gemini model and simply try it directly on another Gemini model, it will work with high probability.” This insight emphasizes the potential ease with which attackers can adapt their strategies across different model versions.
Iterative Improvement through Fun-Tuning
The study also explored the iterative nature of the Fun-Tuning method. It noted a pronounced improvement in attack success rates at specific iteration points, particularly after iterations 0, 15, and 30. The results showed that Fun-Tuning benefits significantly from restarts. According to the findings, most advancements in success occurred within the first five to ten iterations, indicating a strategic advantage could be gleaned through multiple restarts.
In contrast, the ablation method, which operates without the guided adjustments found in Fun-Tuning, resulted in random guesses with limited incremental success. This lack of a structured approach indicates why Fun-Tuning consistently outperformed the ablation technique.
Effectiveness of Prompt Injections
The effectiveness of different prompt injections was evaluated, revealing varied success rates. Two specific techniques were highlighted:
- Phishing Attempt: Aimed at stealing passwords via a phishing site, this method had a success rate below 50%.
- Python Code Mislead: This attempted to mislead the model regarding the input of Python code and also resulted in a success rate below 50% for Gemini 1.5 Flash.
The researchers speculated that Gemini’s additional training in countering phishing attacks might explain the lower success in that scenario. For the Python coding test, it appeared that Gemini 1.5 Flash had significantly enhanced capabilities in code analysis, leading to a reduced success rate.
Visual Insights
The findings of this research were further illustrated through various charts and graphs showing the comparative success rates of attacks across different Gemini models and fine-tuning strategies. These visual aids help to better understand the performance dynamics between the models and the tuning methods applied.
Supporting Visualizations
- Attack Success Rates of Gemini 1.5: This image shows the high success rates achieved through Fun-Tuning.
- Performance Comparison of Gemini 1.0 Pro: This highlights the differences in success rates against various attack methods.
The research findings provide intriguing insights into the capabilities and weaknesses of Gemini models in the face of attacks, indicating a clear trend toward the effectiveness of well-structured Fine-Tuning methods over conventional approaches.