Google DeepMind Launches Omni×R: A Holistic Evaluation Framework for Assessing Reasoning Abilities of Omni-Modality Language Models Utilizing Text, Audio, Image, and Video Inputs
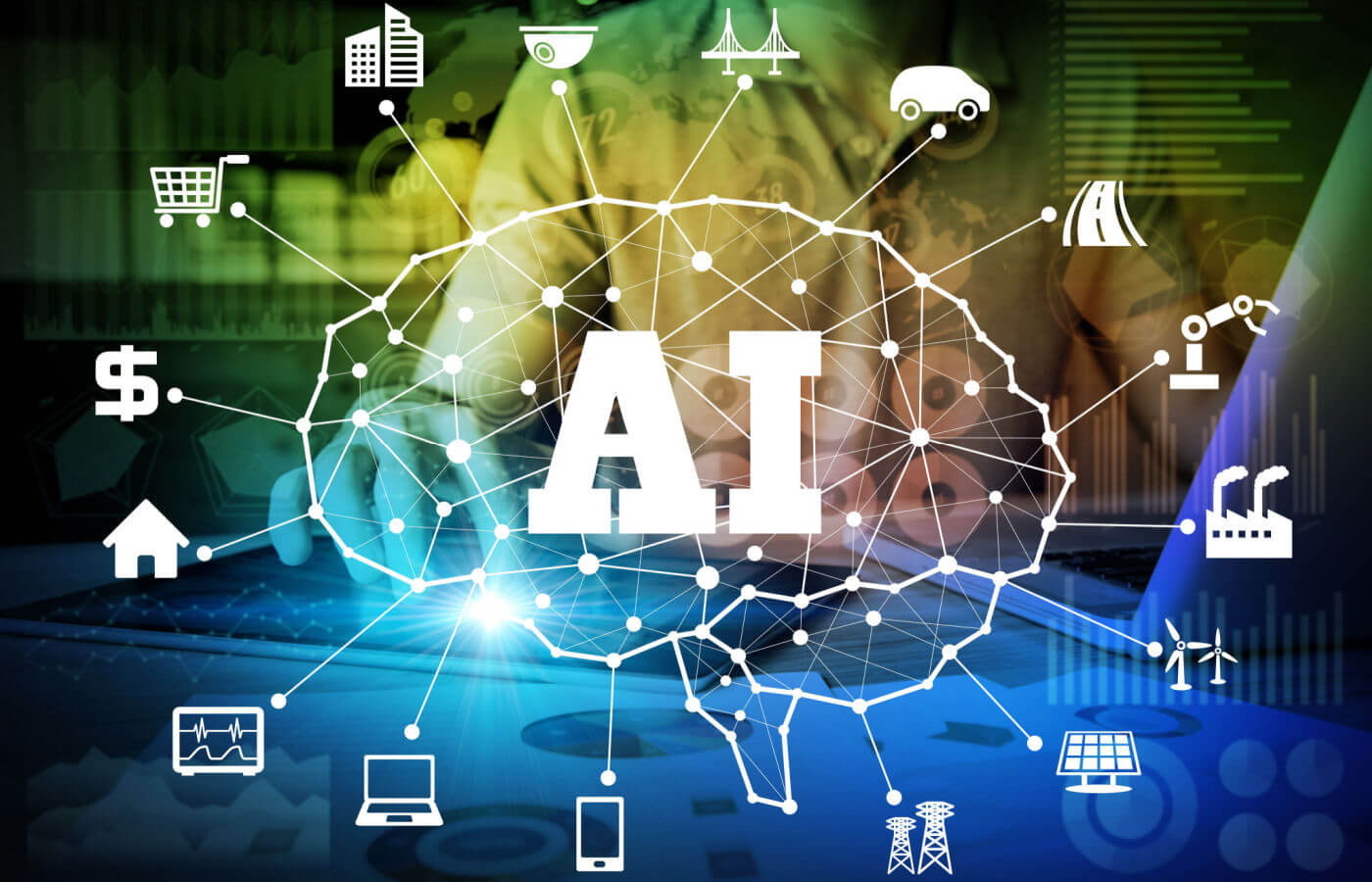
Google DeepMind Unveils Omni×R: A New Evaluation Framework for Language Models
Introduction to Omni×R
Google DeepMind has launched an innovative framework known as Omni×R. This framework is designed to evaluate the reasoning capabilities of omni-modality language models. These models can process and understand various types of inputs, including text, audio, images, and videos. With the demand for advanced AI systems growing, Omni×R aims to set a benchmark for measuring how well these models perform across multiple modalities.
The Importance of Multi-Modal Learning
What is Multi-Modal Learning?
Multi-modal learning refers to the ability of AI systems to process and integrate data from different sources. For example, an AI that can analyze an image and also understand descriptive text about it exhibits multi-modal capabilities. This type of learning is crucial for tasks that require a broader understanding of context, such as in AI applications in healthcare, education, and entertainment.
Benefits of Multi-Modal Models
- Improved Context Understanding: By combining information from various inputs, AI can provide more accurate insights and responses.
- Enhanced User Experience: Multi-modal models can interact with users in a more natural and engaging way, accommodating various preferences for input.
- Broader Application Range: These models can be used in diverse fields, such as virtual reality, robotics, and autonomous vehicles.
Features of Omni×R
Comprehensive Evaluation
Omni×R provides a structured approach to assess the reasoning capabilities of omni-modal language models. It includes criteria for evaluating performance metrics in:
- Text comprehension
- Audio understanding
- Image recognition
- Video analysis
By offering a comprehensive evaluation, researchers and developers can identify strengths and weaknesses in their models, helping them to enhance performance effectively.
Benchmarking Across Modalities
The framework allows for benchmarking across different modalities, meaning it can compare how well a model handles various types of data inputs. This feature is particularly significant in understanding how seamlessly a model can switch between different sources of information.
Importance for Developers and Researchers
For developers and researchers in the field of artificial intelligence, using Omni×R means they can:
- Align Models with Industry Standards: By adhering to established benchmarks, developers can ensure their models meet industry expectations.
- Optimize Performance: The insights gained from the evaluations can guide improvements, enhancing model performance over time.
- Enhance Collaboration: A standardized evaluation framework promotes collaboration among researchers by providing a common ground for comparison and discussion.
Implications for the Future of AI
The introduction of Omni×R marks a significant step forward in the world of AI. As omni-modality models grow increasingly complex, having a reliable evaluation framework can drive innovation and further development. It sets the foundation for future advancements, ensuring that language models can effectively process data from multiple sources and deliver smarter, more contextualized responses.
Conclusion
With the growing sophistication of AI technologies, frameworks like Omni×R become essential. By establishing clear benchmarks, it not only aids in assessing current models but also paves the way for future breakthroughs in artificial intelligence across a variety of fields.