Google DeepMind Unveils QuestBench: A Tool for Assessing LLMs’ Skills in Identifying Gaps in Reasoning Tasks
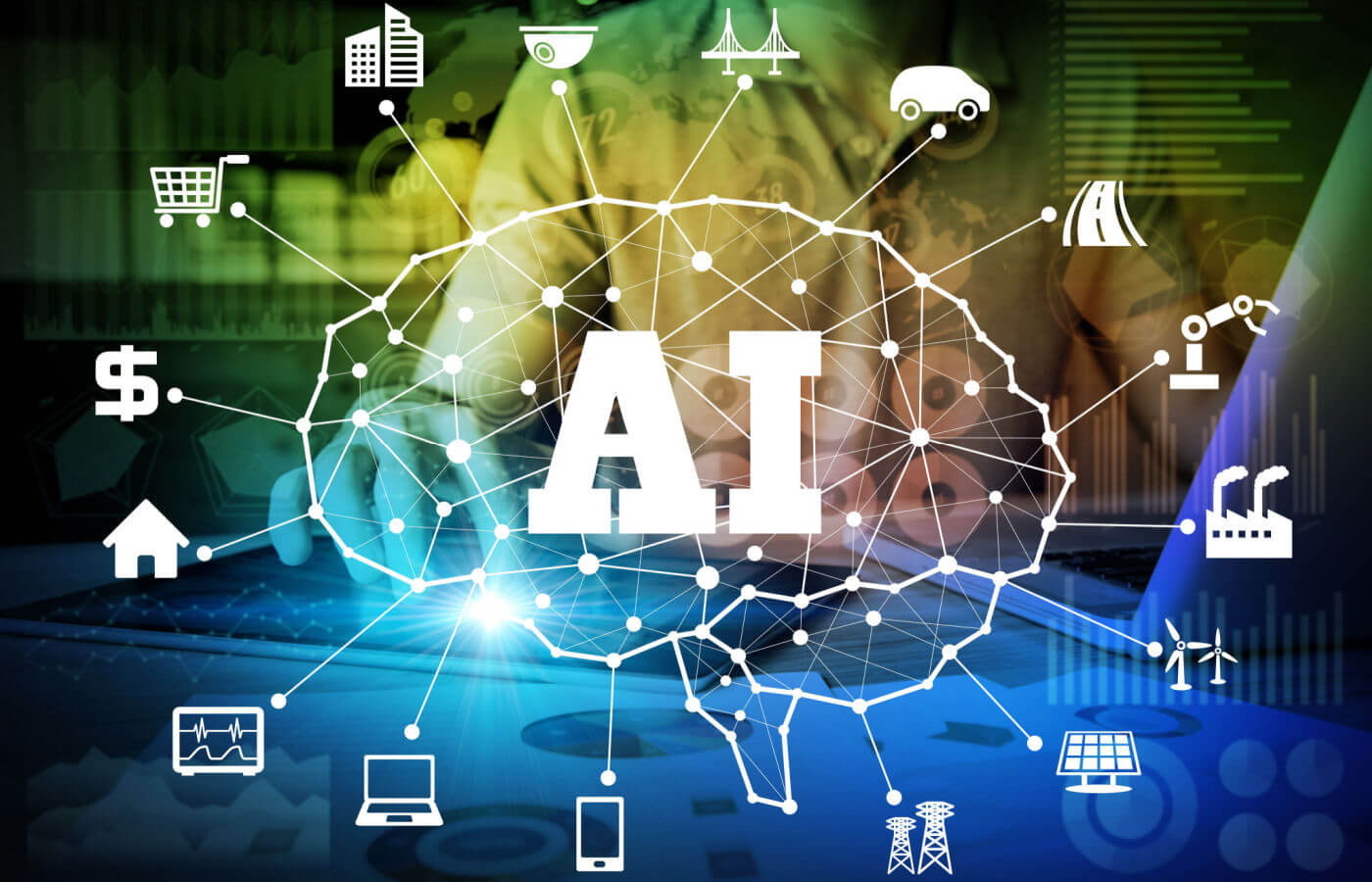
Google DeepMind Unveils QuestBench: A New Evaluation Tool for Language Models
Google DeepMind has recently introduced an innovative evaluation framework called QuestBench. This tool is designed to assess the capabilities of large language models (LLMs) in identifying information gaps during reasoning tasks. Understanding this tool’s features and implications can help users appreciate its significance in the realm of artificial intelligence.
What is QuestBench?
QuestBench is an advanced benchmarking system aimed at evaluating the performance of LLMs in completing various reasoning tasks. Unlike traditional assessments, which often focus on generating text or answering questions directly, QuestBench emphasizes the model’s ability to recognize when critical information is missing. This capability is crucial for developing more reliable AI systems that can better understand context and reasoning.
Key Features of QuestBench
Focus on Information Gaps: QuestBench specifically tests how well LLMs can spot missing information essential for reasoning tasks. This is a step forward from conventional benchmarks that may not address this aspect.
Diverse Task Types: The evaluation covers a wide variety of reasoning tasks, ensuring that the models are tested on different fronts. This diversity helps paint a clearer picture of their abilities.
- Robust Data Collection: The researchers at DeepMind have established a rigorous method for collecting data that feeds into the QuestBench framework. This allows for consistent and reliable evaluation of different models.
Why is QuestBench Important?
QuestBench plays a vital role in enhancing the understanding of AI systems’ limitations and strengths. By focusing on identifying gaps in reasoning, it sheds light on how LLMs comprehend information. This is crucial for several reasons:
Improved Model Training: Understanding where models struggle can lead to better training processes and refinement, ultimately improving their performance.
Greater Reliability: Models that can identify missing information are likely to produce more coherent and contextually accurate outputs, contributing to more reliable AI applications.
- Applications Across Fields: With well-functioning LLMs, the applications extend to various domains, including education, healthcare, and technology, where intelligent reasoning is essential.
Potential Challenges for LLMs
Even with tools like QuestBench, assessing the intelligence of LLMs reveals several challenges:
Limited Understanding of Context: Many models still struggle with grasping the full context of information, which can lead to incomplete reasoning.
Dependence on Training Data: The quality and diversity of training data significantly influence the model’s ability to reason and identify gaps.
- Ambiguity in Language: Natural language is inherently complex and ambiguous. LLMs may find it difficult to navigate this intricacy when identifying missing information.
Future Implications
The advent of QuestBench marks a significant step in understanding and improving language models. As AI continues to evolve, tools like QuestBench will likely inform the development of more sophisticated systems capable of deeper reasoning and comprehension. Continuous improvements in these areas will foster more effective human-computer interaction and open up new avenues for utilizing AI technology in everyday life.
In summary, QuestBench presents an innovative approach to evaluating large language models by focusing on their ability to identify missing information in reasoning tasks. With ongoing research and development, we can expect significant advancements in the functionality and reliability of AI systems.