Google Gemini Model Introduces Adjustable Reasoning Feature
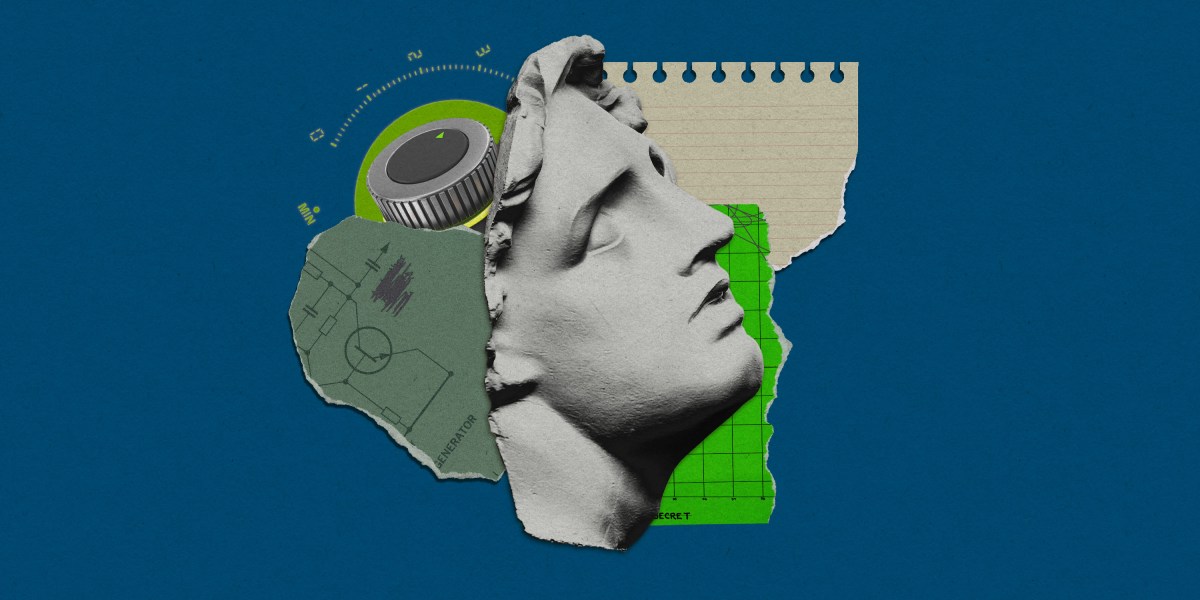
The Rise of Reasoning Models in AI
Introduction to Reasoning Models
In recent times, there has been a significant push towards enhancing artificial intelligence (AI) through the development of reasoning models. Jack Rae, a principal research scientist at DeepMind, emphasizes the importance of “thinking” in these models. These systems are designed to solve problems logically, dedicating substantial time to reach conclusions. The introduction of the DeepSeek R1 model earlier this year marked a milestone in this field. Reasoning models are appealing for AI companies as they allow improvements to existing models rather than necessitating the creation of entirely new ones.
Cost Implications of Reasoning Models
Running Costs
The processing requirements for reasoning models often lead to increased operational expenses. For instance, leaderboards tracking reasoning models indicate that completing a single task can exceed $200. Despite the high costs, the potential benefits include superior performance in managing complex tasks, such as debugging code or compiling extensive information from various documents. The additional time and resources invested may yield better results for challenging inquiries.
Iterative Approach
Koray Kavukcuoglu, Chief Technical Officer at Google DeepMind, highlights the value of iterating through different hypotheses during the reasoning process. This method aims to improve the likelihood of arriving at the correct answer.
Challenges with Overthinking
The Pitfalls of Excessive Reasoning
However, not all outcomes from increased reasoning time are favorable. Tulsee Doshi, leading the product team at Gemini, points out that the Gemini Flash 2.5 model can “overthink” for more straightforward prompts. In such cases, the model’s excessive evaluation does not enhance its output quality, leading to inefficiencies.
Environmental Concerns
The environmental impact is another serious consideration. When AI models extend their processing duration unnecessarily, it results in higher energy consumption, exacerbating their carbon footprint. Nathan Habib, an engineer at Hugging Face, expresses concern about the tendency of companies to apply reasoning models indiscriminately. In some instances, reasoning is not suited to the problem being addressed.
Performance Variability
Mixed Results Across Different Tasks
The performance improvements of reasoning models may be clear for specific functions. According to Habib, while these models demonstrate undeniable gains in certain areas, they may fall short in many typical AI applications. He illustrates this with an example of an organic chemistry problem where a leading reasoning model began with promising responses but quickly devolved into repetitive and unproductive outputs. Kate Olszewska, who evaluates Gemini models, notes that Google’s models can also encounter issues like repetitive looping.
Innovations to Address Current Limitations
Reasoning Dial Feature
To mitigate the challenges associated with overthinking, Google has introduced a feature called the “reasoning dial,” currently available for developers rather than the general consumer version of Gemini. This allows developers to control the amount of computational power allocated to a task. By adjusting this dial, developers can optimize the reasoning process, reducing unnecessary computation for simpler tasks. However, it is important to note that outputs generated when the reasoning feature is activated are approximately six times more costly.
The Future of Reasoning Models
As reasoning models continue to evolve, striking a balance between efficiency and performance is vital. Finding the right applications for these models can lead to significant advancements in AI capabilities, but it requires careful consideration to avoid unnecessary complexity and high operational costs. The focus will need to shift towards understanding when reasoning is beneficial and when it may hinder performance, ensuring that AI technologies are both effective and sustainable.