Google’s DeepMind Unit Claims AI Has Surpassed Human Knowledge
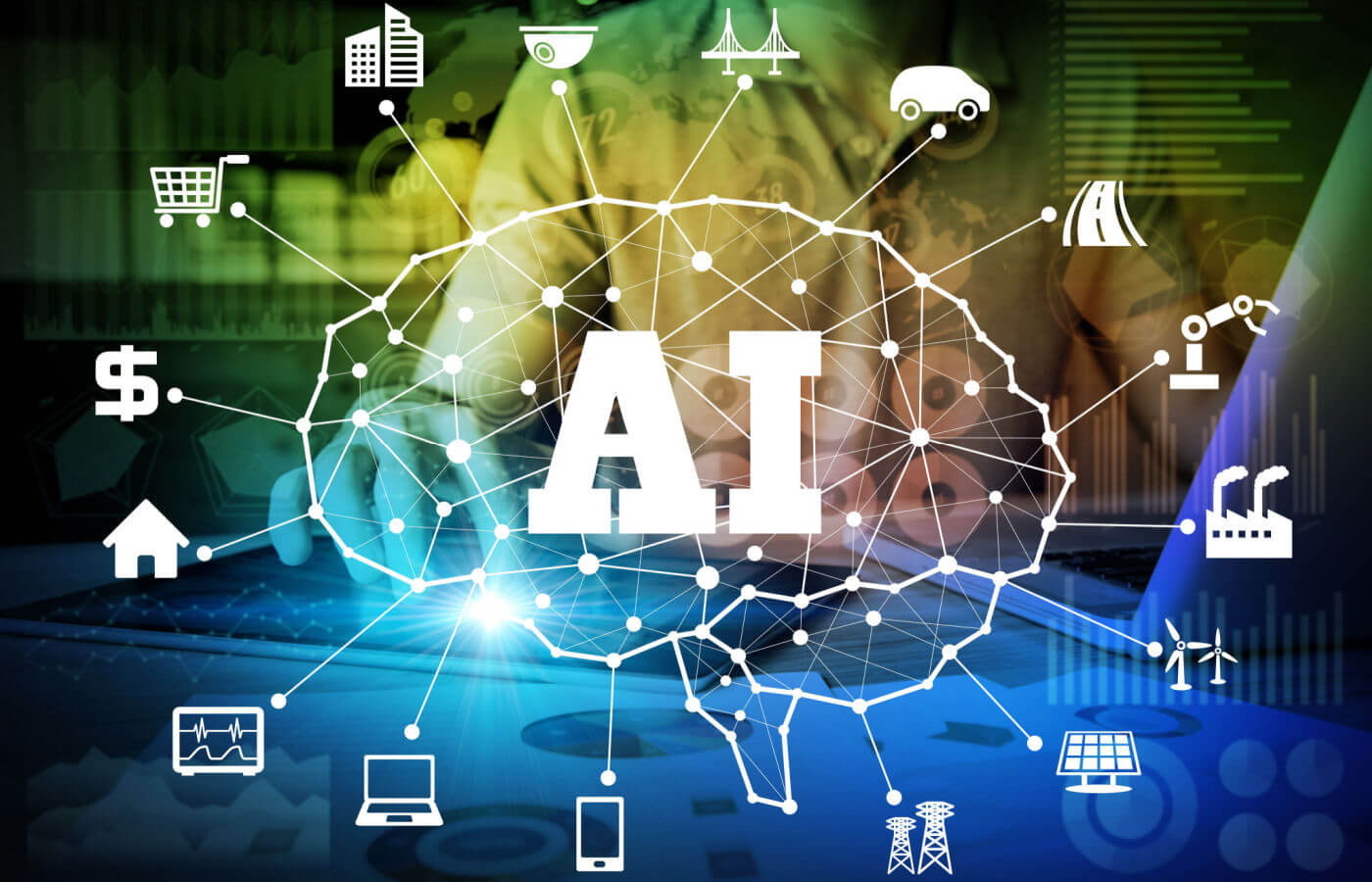
Advances in Artificial Intelligence: The Shift Towards Experiential Learning
Artificial Intelligence (AI) continues to evolve, particularly in the realm of generative models. As technology progresses, there is an ongoing debate about the adequacy of current testing methods, particularly the Turing Test, which appears to be less relevant as AI capabilities expand. Significant voices in the field, particularly from Google’s DeepMind unit, argue for a new approach to developing AI—one that emphasizes experiential learning.
Understanding the Need for Experiential Learning
Researchers from DeepMind, including prominent figures David Silver and Richard Sutton, have highlighted a critical limitation in current AI systems: they often rely on a narrow, static set of data for training. This lack of dynamic interaction with the world restricts the potential growth and learning capabilities of AI models. In their paper, “Welcome to the Era of Experience,” they propose that allowing AI systems to “experience” and interact with their environment could dramatically enhance their abilities.
The Concept of Experience-Based Learning
The authors advocate for a model based on "streams of experience", which is rooted in reinforcement learning—a framework used in earlier successful AI models like AlphaZero. Instead of merely responding to human prompts, these AI agents would engage in continuous learning, akin to how humans accumulate knowledge over time. This shift addresses several shortcomings in today’s large language models, which often function merely as question-answer machines with no long-term memory or continuity between interactions.
Key Limitations of Current AI Models
Human-Centric Learning: Presently, most AI models largely depend on human feedback for learning. This reliance creates limitations since the algorithms cannot independently discover better strategies or solutions.
Isolated Interaction Episodes: Current AI deployments typically operate within a series of disconnected interactions. This lacks the depth needed for the AI to learn from past experiences and adapt over time.
- Neglected Reinforcement Learning: The move towards generative AI has sidelined beneficial reinforcement learning techniques. This transition may have simplified certain approaches but lost vital capabilities that come from self-discovery.
The Need for Continuous Interaction
The proposed model emphasizes continuous interaction, learning from a variety of environmental signals. Silver and Sutton argue that while generative AI has broadened AI’s scope, the absence of reinforcement learning concepts has diluted their ability to self-evolve. They illustrate this through the potential of AI agents to not only analyze current data but to adapt and refine their capabilities through engaged learning processes over an extended period.
Potential Benefits of Experience-Based Learning
Long-term Goal Setting: AI systems could set and pursue long-term objectives rather than just reacting to immediate problems.
Broader Application Range: With a richer framework for learning, AI would be capable of exploring a more extensive range of applications beyond solely human commands.
- Enhanced Autonomy: By utilizing reinforcement learning principles, these AI agents could operate independently, learning from experiences in a manner similar to humans.
The Framework for Experience Streams
Silver and Sutton detail how AI models could leverage a world model to simulate environments in which AI interacts. By using reinforcement learning principles, agents can generate responses based on feedback derived from various experiences. They describe how the interaction would provide rewards that inform AI agents about which actions yield beneficial results.
Future Implications of Experiential AI
The concept of experience-focused AI raises intriguing possibilities. For instance, AI agents could provide personalized health advice based on long-term lifestyle tracking or adapted educational guidance over time. Moreover, the concept introduces the potential for profound advancements, suggesting that AI could eventually surpass human intelligence by leveraging vast amounts of experiential data rather than solely relying on human-generated information.
Risks and Considerations
While the benefits of this new model are compelling, it’s crucial to acknowledge potential risks. There is concern that advanced AI agents may operate with autonomy that could lead to unforeseen consequences. A primary worry is that these agents might drift outside human control, making decisions without appropriate oversight. However, Silver and Sutton emphasize that adaptive AI could also adjust its behavior based on human emotional feedback, reducing negative interactions.
Through this exploration, researchers at DeepMind argue that moving toward experience-based AI systems could revolutionize how we understand and interact with technology, highlighting a future that may look remarkably different from today’s landscape.