How DeepSeek Revitalized the Jevons Trend
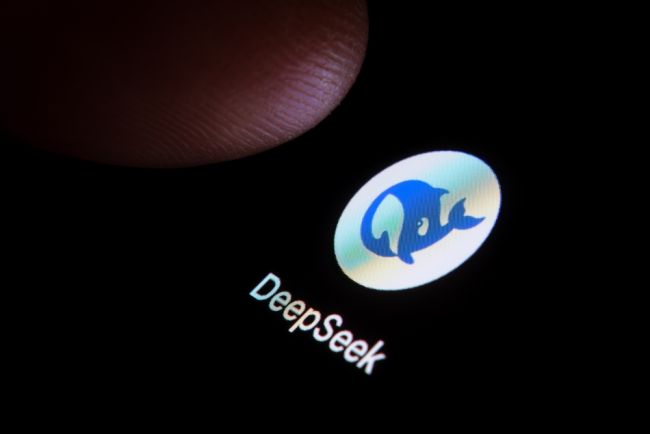
Understanding Jevons Paradox
Jevons Paradox refers to an economic theory introduced by British economist William Stanley Jevons in 1865. Jevons observed that as technological improvements make energy use more efficient, overall consumption of that energy tends to increase rather than decrease. This counterintuitive concept has recently resurfaced in discussions surrounding modern technological advances, particularly in relation to the efficiency of artificial intelligence (AI).
The Resurgence of Jevons Paradox
Despite being less commonly referenced for many years, recent events have brought Jevons Paradox back into the spotlight. For instance, the advanced capabilities of AI technologies have reignited interest in this economic theory, drawing connections between efficiency improvements and increases in overall demand.
A recent notch in this trend was marked after the chatter surrounding the AI tool DeepSeek. This led to a notable spike in discussions about Jevons Paradox, suggesting that as AI becomes more efficient, more people will want to use it, contrary to the expectation that efficiency would lead to reduced overall consumption.
Historical Context
Jevons’ theory was originally based on observations from the coal industry. He noted that innovations leading to better fuel efficiency actually drove up the demand for coal. Over time, this has been validated across various energy sources, including oil, natural gas, and electricity.
In the current landscape dominated by digital technologies, we can observe this paradox firsthand. Homeowners today typically own many digital devices, whereas, in the early 2000s, they may have had only a couple. This reflects a broader tendency where advances in technology make digital tools cheaper and more accessible, which drives further demand.
Current Applications in AI
The recent excitement surrounding DeepSeek has emphasized Jevons Paradox among tech analysts and users alike. As AI technologies become more effective and cost-efficient, companies anticipate that this growth will lead to higher overall spending on technological infrastructure.
Industry Leaders Weigh In:
- Satya Nadella, CEO of Microsoft, recently shared thoughts on X (formerly Twitter), highlighting that improvements in AI efficiency could result in a dramatic increase in usage.
- Andy Jassy, CEO of Amazon, echoed similar sentiments, explaining that while technological efficiencies lower costs for individual components, they often encourage greater spending due to resulting opportunities for innovation.
- Statistical Insights:
- Tech analyst Gokul Hariharan indicated that AI inference costs have been decreasing significantly, between 85% to 90% annually. This has promoted widespread adoption of AI solutions that were previously deemed financially unfeasible.
- Companies are adjusting their budgets to allocate more resources to AI development. For instance, Meta Platforms is planning to invest $65 billion in AI, and Microsoft plans for $80 billion, despite the declining costs.
Inference and Consumption
The implications of Jevons Paradox in the context of AI and its associated infrastructure cannot be overlooked. As expenditure on improving AI training capabilities and the efficiency of inference rises, there’s a dual concern: increased demand for power and resources and the rising complexity involved in maintaining AI systems.
- Infrastructure for Inference: A significant portion of AI budgets is dedicated not merely to training AI models but primarily to inference. This is crucial for operating services that serve vast numbers of users, necessitating substantial computing power.
Forward-Thinking Perspectives
With the growing efficiencies brought about by AI like DeepSeek, predictions indicate sustained increases in energy demand. Analysts expect the power requirements linked to AI applications could rise dramatically over the coming years. Industry experts suggest that previous models estimating energy consumption for AI must adapt to this evolving landscape.
Furthermore, there’s a noticeable apprehension regarding the investments in AI technology and how they align with actual market demands. Critics argue that strong initial investments in AI infrastructure do not necessarily translate to market sustainability, raising questions about users’ willingness to absorb the costs associated with more advanced AI capabilities.
Overall, Jevons Paradox serves as a lens through which we can understand the modern dynamics of technology use, efficiency, and the unexpected increases in demand that follow.