Implementing Transformational Enterprise-Wide Agents: A Microsoft Case Study
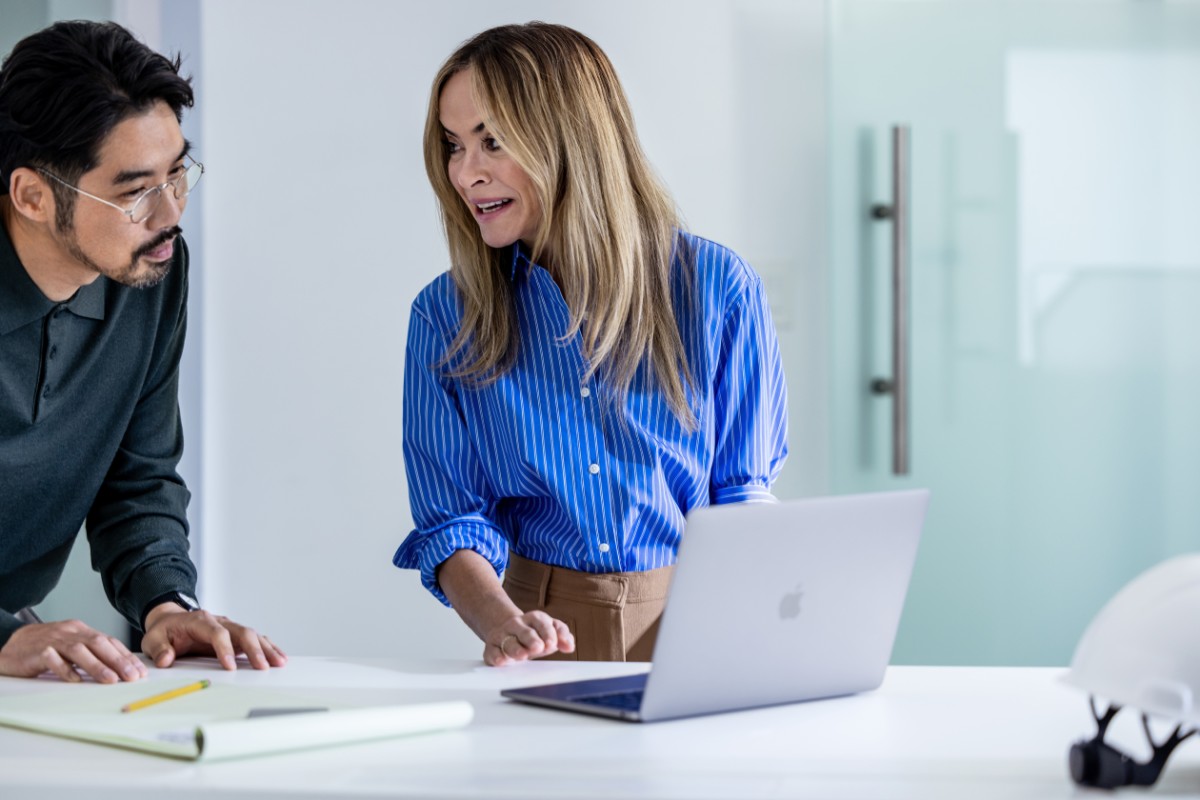
The Rise of AI Agents: Best Practices for Enterprise Deployment
In recent months, AI agents have emerged as essential tools for organizations striving to innovate rapidly while also ensuring security and governance. Businesses are increasingly recognizing the importance of AI in enhancing operational efficiency and improving employee experiences.
Microsoft’s Journey with AI Agents
Microsoft is actively exploring the application of AI agents, particularly with the rollout of their Employee Self-Service (ESS) agent. This initiative has provided valuable insights into securely building, deploying, and evaluating the success of AI agents across large organizations.
Developing the Employee Self-Service Agent
The creation of Microsoft’s self-service agent traces back to the launch of the HRWeb SharePoint site in 2013. Initially designed to centralize HR policies and benefits information, this platform laid the groundwork for a chatbot introduced in 2021 to streamline HR inquiries. With the integration of generative AI, the ESS agent can now address HR queries efficiently, offering accurate, context-aware responses and automating various tasks such as policy lookups and time-off requests. This deployment has resulted in significant cost reductions in HR and enhanced employee productivity and satisfaction.
Key Considerations for Deploying AI Agents
The journey of launching an enterprise-wide AI agent revealed five key areas organizations should focus on:
- Purposeful Planning: Define the objectives, potential challenges, and success metrics before development.
- Knowledge Source Selection: Identify and secure the best data pools for the AI agent.
- Security and Compliance: Implement safeguards to ensure responsible AI usage and compliance with regulations.
- Pilot Testing: Build and evaluate a pilot version to gather feedback from end users.
- Scaling Up: Create a strategy for broader adoption and measure the agent’s impact.
Planning with Purpose
Before embarking on the development of an AI agent, it is vital to establish its purpose. This includes understanding:
- The pain points in the current support system
- The demographics and needs of end users
- How generative AI can enhance the resolution process compared to traditional chatbots
Microsoft identified the need for a more efficient support system, leading to the vision of a generative AI agent that could provide higher resolution rates and a superior user experience.
Selecting and Securing Knowledge Sources
Once the purpose is established, the next step is selecting the best knowledge sources. Organizations should focus on:
- Utilizing secure and reliable information sources.
- Implementing role-based access control to prevent unauthorized access.
- Ensuring the information is up-to-date and comprehensive for the target audience.
At Microsoft, leveraging HRWeb as a foundational knowledge source allowed for secure and effective interactions, supported by regular reviews to keep data relevant.
Implementing Data Loss Prevention Policies
Setting up robust data loss prevention policies is critical. Companies should create separate environments for development, testing, and production, which can be managed through tools like Copilot Studio. Ensuring compliance with security policies allows for safe data interactions while utilizing AI agents.
Ensuring Security and Compliance
After developing the pilot version, it’s vital to undergo comprehensive assessments covering security, compliance, privacy, and responsible AI. Microsoft emphasizes:
- Conducting detailed software development lifecycle assessments.
- Verifying data encryption both in transit and at rest.
- Implementing thorough auditing and logging for interactions.
This multi-faceted approach ensures that all security and compliance requirements are met before the AI agent moves into broader testing and deployment phases.
Building and Testing the Pilot Agent
With suitable knowledge sources and security measures in place, organizations can begin building the AI agent. For scenarios involving sensitive data, the full Copilot Studio is preferable, allowing for customizable responses to ensure accuracy and compliance.
Selecting the Pilot Audience
Choosing an ideal pilot audience is crucial for gathering initial feedback. Engaging a targeted group for A/B testing against existing solutions allows organizations to refine the agent based on real user experiences. At Microsoft, this step was taken by piloting the ESS agent with a focused group of employees to maximize insights and impacts.
Scaling and Measuring Impact
The successful pilot of the ESS agent indicated potential, but expanding it across the enterprise required strategic planning. Microsoft tackled this by analyzing past HR interactions to prioritize essential data sources and views.
Rolling Out at Scale
Rapid scalability was achieved by integrating existing Microsoft Power Platform connectors and optimizing deployment in key regions and teams, such as sales. Organizations should prioritize their deployment strategies to align with overall corporate goals and timelines.
Measuring Success
Finally, it is crucial to track the agent’s performance to demonstrate its value. Key metrics include session counts, engagement rates, customer satisfaction scores, and resolution rates. These insights help assess the ROI of the AI agent and guide future enhancements.
By establishing a concrete framework for deploying AI agents, organizations can enhance operational efficiency while driving positive employee experiences.