Increase in Failure Rates for AI Projects: A Report
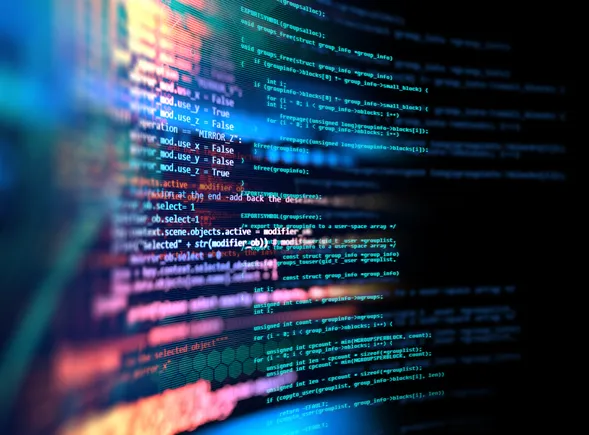
Overview of AI Project Failures in 2023
Increased Failures Across Enterprises
Recent analysis by S&P Global Market Intelligence shows a significant rise in AI project failures this year compared to the previous one. The findings stem from a survey that included over 1,000 respondents across North America and Europe. Among the alarming statistics, a notable 42% of companies have reportedly abandoned most of their AI initiatives, an increase from just 17% last year. Additionally, organizations discarded an average of 46% of their AI prototype projects before they were fully implemented.
Major Challenges Faced
The reasons for these high abandonment rates highlight core challenges in AI initiatives:
- Cost Implications: Many companies find the financial burden of developing and maintaining AI projects overwhelming.
- Data Privacy Risks: Concerns regarding data security and compliance with regulations continue to deter companies from pursuing AI developments.
- Security Risks: Potential vulnerabilities associated with AI technologies make businesses cautious.
According to S&P’s report, while AI adoption is primarily focused on IT operations, it is also being used in customer experience processes and marketing, albeit with uneven success.
Insights into the AI Landscape
Ongoing Struggles in AI Progression
Chief Information Officers (CIOs) express frustration over their organizations’ lagging progress with generative AI technologies. Many acknowledge that their institutions’ experimentation efforts have often not yielded the expected outcomes. The future likely sees an increase in scrapped projects due to these continuing challenges.
Despite these setbacks, most enterprises are increasing their investments in generative AI. A recent Informatica report revealed that two-thirds of companies are grappling with the challenge of transitioning pilot projects into full-scale production.
Successful Strategies for AI Implementation
Businesses that have been successful in their AI endeavors tend to prioritize and customize specific use cases. Decision-makers who focus on selective AI opportunities rather than attempting to pursue every possible avenue are more likely to avoid failures.
Knowing when to deploy AI and when not to is crucial to make the most efficient use of resources. Recognizing the limitations of AI tools can prevent wasted efforts on projects unlikely to succeed.
Understanding the Value of Failure
Interestingly, failures in AI projects should not always be perceived negatively. As noted by Amanda Luther, a managing director at Boston Consulting Group, celebrating these failures can foster a culture of experimentation among employees. Encouraging staff to propose and test their ideas—for better or worse—can promote innovation and lead to meaningful advancements in the long run.
Moreover, Htike Htike Kyaw Soe, a managing director at KPMG U.S., emphasized the importance of embracing the trial-and-error aspect of new technologies. The notion of failure should not instill fear but rather be accepted as part of the process of adapting to and mastering new tools.
The Path Forward
The exercise of trial and error in AI implementation can lead to iterative learning experiences, ultimately yielding improved results over time. As companies continue to explore the complexities of AI integration, fostering an environment that values experimentation—regardless of immediate success—can result in more robust and successful AI applications in the future.
By understanding the challenges and opportunities presented by AI initiatives, organizations can better navigate the evolving landscape of artificial intelligence technology.