Insights from the Inventor: Exploring Agents, Grounded AI, and Their Impact on Enterprises
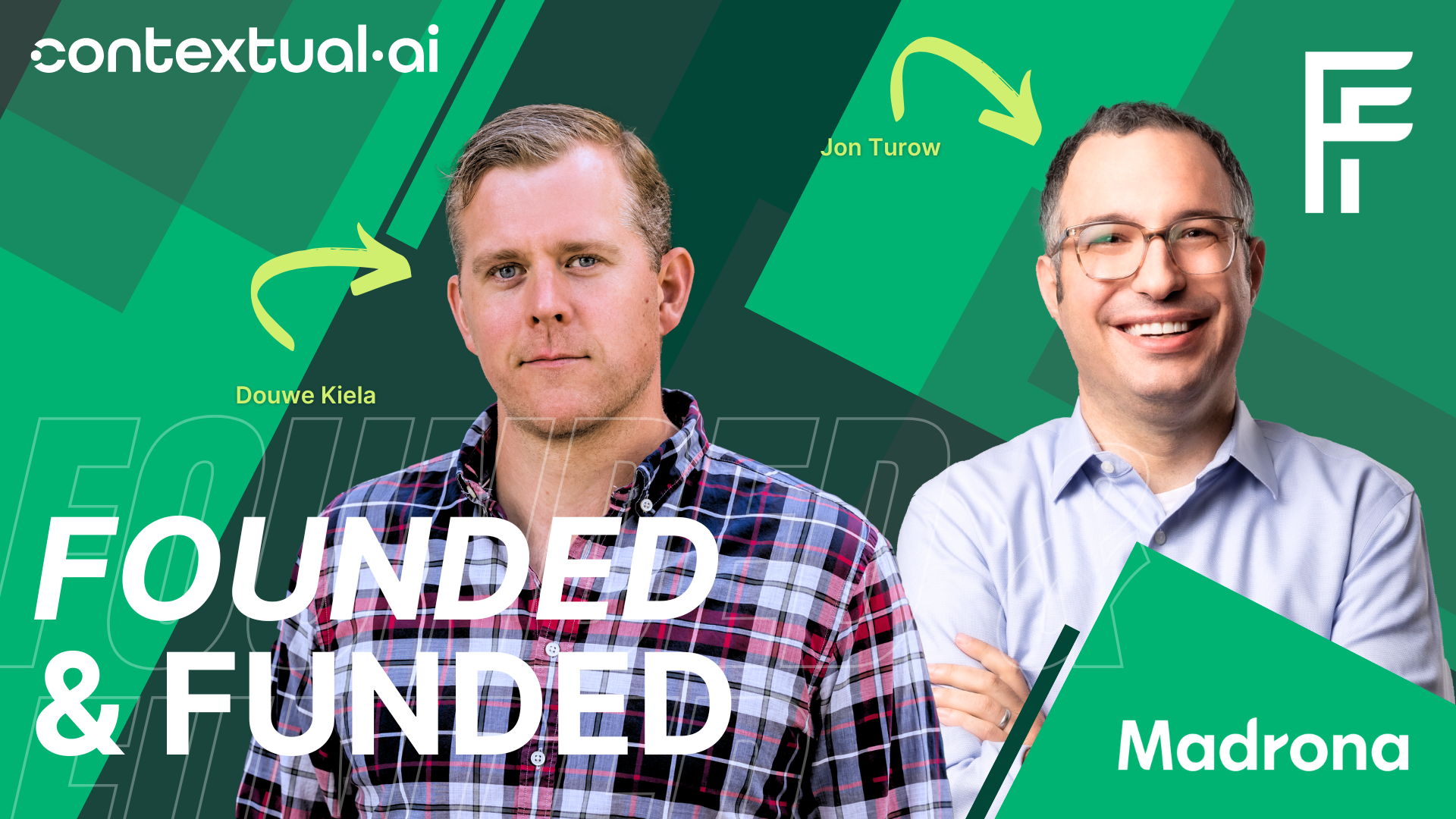
Understanding Retrieval-Augmented Generation (RAG) in AI
Retrieval-Augmented Generation (RAG) is an innovative approach in the field of Artificial Intelligence (AI) that combines generative models with data retrieval techniques. This concept is crucial for anyone involved in AI development, providing a robust framework for addressing complex information tasks.
What Is Retrieval-Augmented Generation?
RAG was initially conceptualized to tackle specific challenges in understanding language in relation to context, extending beyond mere word associations. The foundational idea stemmed from the realization that to truly grasp the meaning of words, one must also understand the context in which they are used. For instance, knowing what a "cat" looks like enhances the understanding of the term itself.
To further develop this idea, Douwe Kiela, the co-creator of RAG, and his team aimed to ground information in textual sources, such as Wikipedia. This led to the integration of generative models with data retrieval systems, allowing the generation of responses based on retrieved information.
The Evolution of RAG
RAG’s development occurred in conjunction with advancements in machine learning, particularly at Facebook AI Research. The approach gained traction as it aligned with the industry’s movement towards generative AI, effectively allowing models to retrieve and utilize external information to enhance their responses. Notably, RAG combines a vector database with generative models, fundamentally changing how information is processed and understood.
Why RAG Matters
RAG should not be viewed as a one-size-fits-all solution; its effectiveness lies in its ability to integrate with various data types. The history of RAG reveals its flexibility—from the initial focus on images to its current application in managing vast amounts of textual information. This adaptability is key to its success and utility in different contexts, especially in enterprise settings where diverse data types must be handled efficiently.
As businesses increasingly leverage generative AI, understanding how RAG fits into the broader landscape of AI solutions is essential. It allows organizations to power applications capable of answering complex queries based on extensive data repositories without requiring every piece of information to be contained within the model itself.
Building Effective AI Infrastructure
To implement RAG successfully, enterprises must consider several factors when evaluating AI solutions:
- Understanding RAG Problems: Not every question can be solved using RAG. Establishing whether a problem is suitable for RAG requires careful consideration of the type of information needed.
- Data Diversity: Companies often collect vast amounts of data for varying contexts. RAG excels in scenarios where traditional generative models might struggle due to knowledge cutoffs.
- AI Evaluation: Enterprises must have robust evaluation metrics to measure the effectiveness of RAG-based systems, ensuring they meet the operational needs.
The Challenges of Implementing RAG
While RAG offers significant benefits, there are still challenges to navigate. For example, one common misconception is that RAG eliminates the risk of "hallucinations," or inaccurate outputs produced by AI models. In reality, RAG can help ground responses in factual data, but inaccuracies may still arise. Thus, businesses need a strategy to mitigate risks associated with these inaccuracies effectively.
The Future of RAG
Looking ahead, RAG is evolving into what is referred to as RAG 2.0, which takes this approach even further. As organizations seek to derive more value from AI technologies, integrating retrieval mechanisms with improved reasoning capabilities is crucial. The future will likely see RAG agents capable of more nuanced interactions with data, enhancing their utility for enterprise applications.
Conclusion
Ultimately, the journey to adopting RAG effectively requires a nuanced understanding of its capabilities and limitations. As AI technology continues to mature, leveraging RAG can lead to more powerful, contextually aware applications in various industries. Then, businesses will be better positioned to harness these innovations to create value and drive efficiencies across their operations.