Insights on Eureka’s Inference-Time Scaling: Current Status and Future Directions
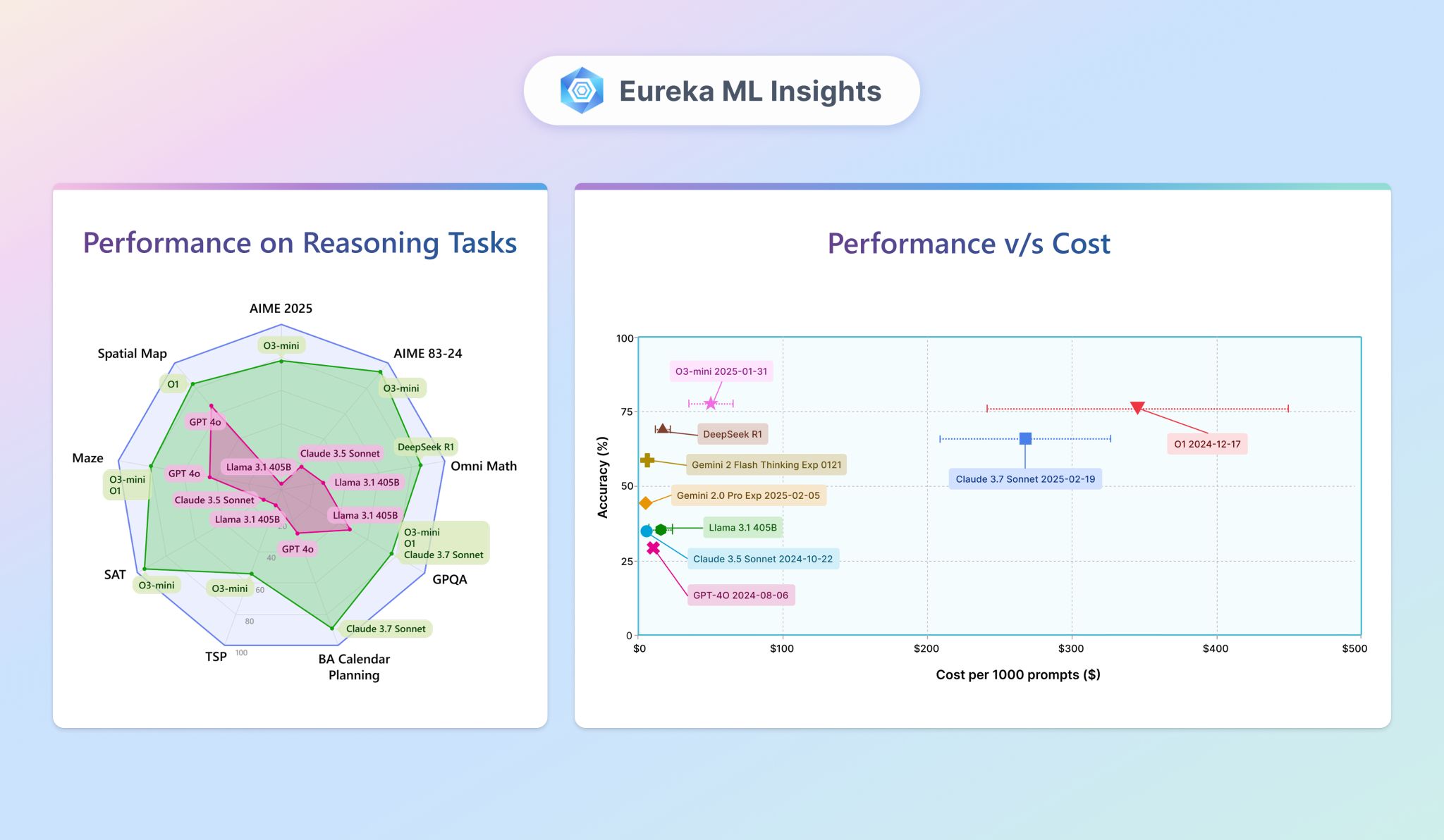
Understanding Reasoning Capabilities in AI Models
The recent "Eureka report on inference-time scaling" explores the reasoning capabilities of large models in Artificial Intelligence (AI). It investigates whether these advanced models can handle complex reasoning tasks beyond simple math, how they compare to traditional autoregressive models, and what potential improvements can still be made in reasoning abilities.
Key Research Questions
The study seeks to answer several pivotal questions:
- Do advanced reasoning models excel in areas beyond math?
- How do they stack up against conventional autoregressive models?
- What is the untapped potential in reasoning tasks?
- Do longer Chain of Thought (CoT) scratchpads lead to better accuracy?
To delve deeper, the study uses a series of experiments on eight different reasoning tasks, analyzed across nine cutting-edge models.
Diverse Complex Reasoning Tasks
The AI models were evaluated on tasks including:
- Mathematical Reasoning: Benchmarked by AIME 2025, AIME 1983-2024, and OmniMATH.
- Scientific Reasoning: Benchmarked by GPQA.
- Planning and Scheduling: Benchmarked by BA Calendar.
- NP-Hard Algorithmic Reasoning: Encompassing Satisfiability (3SAT) and Traveling Salesman Path Optimization (TSP).
- Spatial Understanding: Assessed through maze-solving problems.
The test subjects included both conventional models such as GPT-4o and Gemini 2.0 Pro and advanced reasoning models like Claude 3.7 Sonnet and DeepSeek R1.
Experiment Approach
The research employed two scaling techniques to evaluate the models:
- Parallel Scaling: Making multiple independent calls to a model and combining the results using methods like average or majority vote.
- Sequential Scaling: The model solves the problem, receives feedback if incorrect, and continues until the context budget is exhausted.
Findings of the Study
Finding 1: Performance Discrepancies
There is a notable performance gap between conventional models and those trained for inference-time compute (reasoning models). These reasoning models often outperform their traditional counterparts by more than 50% in accuracy on mathematical tasks. Improvements are also seen in algorithmic challenges and planning tasks. However, advancements in spatial understanding and scientific reasoning are not as substantial, yet still display significant gains.
Finding 2: Variable Effectiveness Across Domains
The effectiveness of inference-time scaling differs significantly across domains. For instance, while reasoning models achieved over 90% accuracy in Physics, they lagged in Biology and Chemistry. Model accuracy tends to decrease as task complexity rises, particularly in algorithmic challenges.
Finding 3: Token Usage vs. Accuracy
More tokens don’t always equate to higher accuracy. The analysis revealed that longer responses could lead to decreased precision. There is considerable variability in token usage among models with similar accuracy, which implies that shorter, more focused responses might be more effective.
Finding 4: Cost Variability
The variations in token usage introduce cost unpredictability for developers and users. The study discovered that this variability could lead to fluctuations in operational costs by up to 40% for reasoning models.
Finding 5: Untapped Potential for Improvement
There is still potential to enhance both conventional and reasoning-based models. By testing multiple runs and verifying with a "perfect" verifier, the research showed that better inference paths could be derived, suggesting room for further fine-tuning and enhancements.
Finding 6: Feedback Efficiency
Current reasoning models improve more swiftly with feedback compared to traditional models, especially in complex tasks. The experiments indicated that models like O1 became more proficient much faster when receiving input after initial attempts, showcasing a notable edge over conventional models like GPT-4o.
Implications for the Future
This research highlights the emerging capabilities of advanced reasoning models and sheds light on the framework for future AI developments. The findings suggest that there are exciting avenues for improvement and exploration in the realm of complex reasoning, which could have significant implications for various applications in AI.