Introducing Llama 4: New Long Context Scout and Maverick Models with Behemoth Featuring 2 Trillion Parameters Coming Soon!
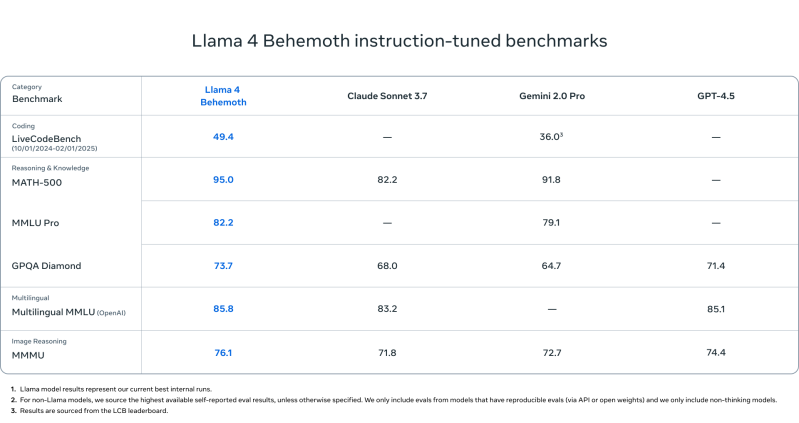
The Rise of DeepSeek and Its Impact on AI
In January 2025, the AI community experienced a significant shift when a relatively unknown Chinese startup, DeepSeek, launched its open-source language reasoning model, DeepSeek R1. Developed by High-Flyer Capital Management, this model quickly gained traction, outpacing established American companies like Meta.
As DeepSeek R1 became popular among researchers and businesses, Meta found itself in a state of alarm, realizing that this new technology had been created for a fraction of the financial investment compared to its premium models. Meta had been banking on its own generative AI, encapsulated in its Llama brand, to be the best in class. The sudden rise of DeepSeek forced Meta to rethink its strategy.
Meta’s Response
Meta’s response was rapid—the company introduced a new series of models known as Llama 4, just a month after the previous Llama 3.3 release, which now seemed outdated. The Llama 4 family includes two models currently available: the 400-billion parameter Llama 4 Maverick and the 109-billion parameter Llama 4 Scout. They are both accessible for download and fine-tuning through Meta’s platforms.
A further model, the Llama 4 Behemoth, boasts 2 trillion parameters but is still undergoing training. This series emphasizes multimodal capabilities, enabling the models to handle text, video, and imagery. Notably, Llama 4 Maverick can process inputs up to 1 million tokens, while Llama 4 Scout can manage up to 10 million tokens, equating to an impressive 15,000 pages of text. This feature is particularly beneficial for fields demanding a deep understanding of complex information.
Key Features of Llama 4 Models
The Llama 4 models come with several groundbreaking features:
Mixture of Experts (MoE): Utilizing a unique architecture combining various specialized models, Llama 4 is designed for efficiency. Each model incorporates 128 specialized experts, reducing computational load by engaging only the necessary components for specific tasks.
Cost Efficiency: The estimated inference cost for Llama 4 Maverick ranges between $0.19 and $0.49 per million tokens, significantly lower than the $4.38 for proprietary models like OpenAI’s GPT-4o.
- Enhanced Reasoning Capabilities: Llama 4 models excel at tasks requiring reasoning, coding, and systematic problem-solving. They are built to compete with other major models, although they still need refinement in certain reasoning capabilities compared to specialized alternatives.
Training Enhancements
Meta has employed advanced training methodologies to enhance the models further:
Selective Focus: During training, it eliminated over 50% of simpler prompts, opting instead for more challenging scenarios to promote robust learning.
Continuous Learning: The reinforcement learning loop provides progressively tougher challenges, ensuring continuous improvement in performance across various domains.
- Hyperparameter Tuning: A new methodology, MetaP, allows for efficient hyperparameter adjustments to maintain model performance while scaling up, saving both time and resources.
Performance Comparison
Despite their impressive capabilities, Llama 4 models are not necessarily the most powerful in the AI landscape. For instance, Llama 4 Behemoth outperforms models like GPT-4.5 and Gemini 2.0 Pro in certain benchmarks, yet it still lags behind peers like DeepSeek R1 in others such as MMLU scores.
In head-to-head comparisons, Llama 4 Behemoth shows competitive performance, particularly when compared to DeepSeek R1, with mixed results across several metrics:
- MATH-500: Llama 4 Behemoth scored lower than both DeepSeek R1 and the OpenAI o1 model.
- GPQA Diamond: It exceeded DeepSeek but fell short against OpenAI.
- MMLU: It showed strong performance but did not outperform both major competitors.
Safety and Bias Considerations
Meta has placed significant emphasis on safety and alignment, introducing tools like Llama Guard and CyberSecEval, designed to enhance model reliability and mitigate political biases. Meta claims improvements in addressing traditional biases found across many large language models, indicating a move towards a more balanced performance in discussions around sensitive topics.
With the launch of the Llama 4 series, Meta is positioning itself as a formidable player in the AI space, aiming to provide open-source alternatives that rival established proprietary offerings, pushing toward a more inclusive future in AI development.